Zero-Shot Learning in Maritime Domain: Classification of Marine Objects using CLIP
Keywords:
Clip, Marine Objects, Vision-language models, Transformers, Zero-shoot learningAbstract
Maritime security and monitoring are essential for global trade, environmental protection,
and national defense. Traditional machine learning models have been effective in recognizing and
classifying maritime objects, but their reliance on large, labeled datasets poses challenges,
particularly in dynamic environments where new and unforeseen objects frequently emerge. This
study explores the application of Zero-Shot Learning (ZSL) to the maritime domain, leveraging the
CLIP model to classify maritime objects with minimal labeled data. A custom dataset comprising
1,438 images was used to evaluate the performance of various CLIP model variants. Our findings
indicate that CLIP models, particularly the "clip-vit-large-patch14-336" variant, achieve high
classification accuracy, with AUC values approaching 1.0 across most classes. However, challenges
remain in handling rare or ambiguous classes such as cargo ships, where F2 scores suggest
variability in recall and precision. Additionally, the study highlights the potential limitations of
these models, including their dependency on dataset diversity and the risk of overfitting to specific
data characteristics. The "clip-vit-large-patch14-336" model is identified as the most balanced and
reliable option, offering a strong foundation for enhancing maritime situational awareness and
supporting diverse maritime applications.
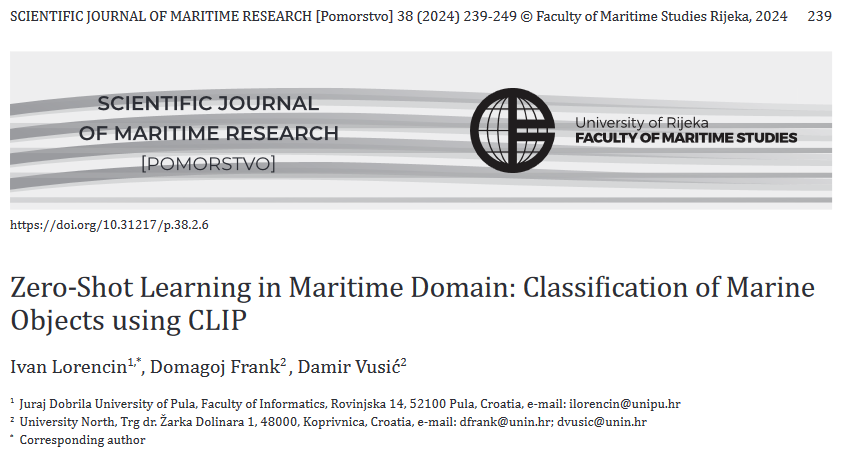
Downloads
Published
Issue
Section
License
Copyright (c) 2024 Ivan Lorencin, Domagoj Frank, Damir Vusić

This work is licensed under a Creative Commons Attribution-NonCommercial-NoDerivatives 4.0 International License.
Scientific Journal of Maritime Research understands the need for authors to disseminate and maximize the impact of their research. When submitting an article for publishing in Scientific Journal of Maritime Research, it implies that the Corresponding Author transfers, with the consent of all Coauthors, the copyright ownership in the referenced submission, including all versions in any format now known or hereafter developed, to the Scientific Journal of Maritime Research.
Copyright protects your original work and research material and prevents others from using it without your permission. Others will be required to credit you and your work properly, thus increasing its impact. Should your submission be rejected or withdrawn prior to acceptance for publication by Scientific Journal of Maritime Research, this transfer will be null and void.
Authors, users or readers of an article need clear instructions on how they can use the article. Scientific Journal of Maritime Research uses the Creative Commons Attribution-NonCommercial-NoDerivatives (CC-BY-NC-ND) 4.0 International License, which governs the use, publishing and distribution of articles by authors, publishers and the wider general public.
The authors are allowed to post a digital file of the published article, or the link to the published article (Scientific Journal of Maritime Research web page) may be made publicly available on websites or repositories, such as the Author’s personal website, preprint servers, university networks or primary employer’s institutional websites, third party institutional or subject-based repositories, and conference websites that feature presentations by the Author(s) based on the published article, under the condition that the article is posted in its unaltered Scientific Journal of Maritime Research form, exclusively for non-commercial purposes.