INTRODUCTION
Granting the security of students in a smart city is imperative. Taking city residents and their need for student accounts is key when it comes to creating smart cities[1]. To achieve the daily state in which students feel secure on the premises of an educational institution, up-to-date security solutions are to be implemented. The fast evolution and extended use of ICT has deeply affected the knowledge management[2]. Smart cities are unimaginable without modern security solutions. By the implementation of up-to-date camera systems, students’ security may be guaranteed, and trespassers could be kept out. The cameras featuring artificial intelligence may provide help in face detection, face recognition, and headcount, and may utilize deep learning. With the simultaneous implementation of these capabilities, it will be possible to compose an attendance register by security cameras. Being part of smart cities, the composition of an automatic attendance register by security cameras may provide great help for teachers, as their daily administrational burden may be lessened this way.
SPECIFICATIONS OF SECURITY CAMERAS
There are numerous types of security cameras. The resolution of modern cameras is at least of HD-class, e.g. of 1280×720 pixels, and in the case of full HD, of 1920×1080 pixels. Some of these features even higher resolution: 4K, of 3840×2160 pixels, while the professional level, high-tech gadgetry is characterized by 8K resolution of 7680×4320 pixels. Besides resolution, another important parameter of security cameras is the image repetition frequency, which is given in units called frame/second (fps). Typical fps-parameter values considering cameras are: 25, 50, 60. It is highly recommended that the fps and resolution values are inversely proportional, so good shots are rarer than normal resolution values. Furthermore, the parameter of bit rate is also worthy to mention, the average being between 10 and 100 Mbit/s. Camera imaging must also be tied to the decisive factor of illumination. With an average camera, the illumination of 0,5 lux is sufficient, whereas, in the case of a camera monitoring a schoolyard, this parameter can be lower, as low as even 0,01 lux. The cameras employing infra-red technology are capable of quality shots even in pitch dark[3].
SPECIFICATIONS OF SECURITY CAMERAS FACE DETECTIONS AND RECOGNITION
While face detection and recognition may represent an easy task for a human being, it is a complex and cumbersome process for security cameras. The development of the technology ensures that cameras are reaching ever higher image resolution, so they can identify faces with increasing precision[4]. Facial biometry represents the most commonly accepted biometric identification process these days. It is utilized in many fields, like on border crossings or at police departments. Frauds, however, do everything in their power to thwart the identification, or rather, to be misidentified. Modern camera systems try to use this meta-data efficiently and to minimize the possibilities for error[5]. One of the most implemented methods is to record the facial images from two different perspectives, as an input image. The deep information may be proofed by this method, as the high-quality 2D printed fake images may be filtered out this way[6]. In the case of a video-surveillance system, it must be firstly determined on what part of the individual frames human faces appeared (if these appeared at all). Then, with the extraction of suitable characteristics, a person is identified, based on an existing database of images. Besides the efficacy of recognition, processing speed is also a significant practical requirement[7]. A human face, by itself, represents an agglomeration of information, from which the person’s age, sex, and emotional state may be derived. However, to utilize the data gained, it is worth to assign the task of recognition to artificial intelligence, as early as within the security camera system itself. This represents a fairly complex image-processing task. To obtain the mentioned meta-data (sex, age, hair color), statistical learning algorithms are commended (Support Vector Machine)[8]. Successful face recognition requires fulfillment of numerous conditions, thus, it represents a more complex task for the processors mounted in the cameras or recording units (DVR, NVR) than just ‘simple’ face detection. Security camera technologies utilizing facial recognition are gaining ground ever-increasingly on the premises of universities. Formerly, cameras were installed on these premises for safety reasons, while today, the behavior of students and their emotions may be increasingly surveyed[9]. The object of the survey may be to identify the emotional states of the students, such as anger, depression, sadness, and happiness[10].
HEADCOUNT
Just a couple of years ago, technology in cameras, capable of efficient headcount was non-existent, as developers tried to count persons with systems featuring only single cameras. These systems often mistook other objects, identifying them as humans, so the information was inaccurate. The most serious problem with systems featuring only single cameras is that they lack a three-dimensional perspective, so it is impossible to examine how tall is the given person or the object. This means, that the system may identify all sorts of lifeless objects – from shopping carts to baby carriages – as humans. The problem is exaggerated if the visibility changes and even shadows may be counted as persons[11]. The most dynamically developing field of science in our age is artificial intelligence – and Deep Learning as a part of it. It gets an ever more important role in the world of security technology. This technology is capable of ‘learning’ based on the data provided, and it is becoming more and more accessible, being able to support a wide variety of security tasks directly or indirectly. The headcount, attempted by systems utilizing traditional cameras were often aggravated by factors influencing accuracy, like unfavorable lighting conditions, shadows, or objects carried across the point of surveillance. The dual-lens head counting camera features two optical devices, and a Deep Learning algorithm, so it provides a highly accurate student headcount. Furthermore, it has a decisive advantage versus the camera algorithms, as it may learn continuously, by data obtained from the outside world. This means that the longer the camera has been operating, the ‘smarter’ it will become[12].
DEEP LEARNING
Deep Learning is a method of machine learning by which models can be constituted more accurately than by the former ones. As machine learning exists in Neural Networks, by the analogy of living organisms, there are machines capable of learning. Through machine learning, a machine – the learning system – gains information from its environment, improving its capacities[13]. The cameras capable of composing attendance registers, by all means, must feature artificial intelligence. The processing of metadata is possible by cameras featuring the deep learning function. The more metadata a camera acquires through its machine learning process, the fewer mistakes it will commit, and the output values will be more accurate. The most important metadata may come from the students’ attire and hairdos. The attire, worn at a given moment, may greatly aggravate identification, as also may increase the possibility for error. Such may be a hat and a scarf in winter, or sunglasses in summer. By the implementation of artificial intelligence, the factors preventing identification may decrease, as the camera learns to identify these ‘aggravating’ factors. An important element of machine learning may be the recognition of students’ hairdos, as it has frequently happened that a person had sometimes shorter, and sometimes long hair. This may cause a serious problem for a machine. In the case of security camera systems, artificial intelligence means the recognition of perpetual alterations, variations.
COMPOSITION OF ATTENDANCE REGISTERS BY SECURITY CAMERAS
Face recognition is an operation based on a black and white list. Students’ mugshots may be stored on the camera system’s hard disk, thus, the system becomes capable of identifying the students. In case when the system does not find a match, an alarm is switched on, as there is a possible intruder, trying to access an area from which he/she is denied. Access control systems are most suitable for the composition of attendance registers. This is not their specific feature, but this may change rapidly. These days, cameras possess such specific abilities and artificial intelligence by which these tasks may be completed. The composition of an attendance register can be utilized in education extensively. As a first step, the mode of image processing would have been chosen. This may happen by a centralized or by a decentralized method. In the case of the centralized method, the intelligence is built into the network’s recorders. In this case, the images are examined in NVR, so the system may operate with any kind of IP camera, lowering the costs, and avoiding copyright infringement. Its drawback is the need for bandwidth, so the network is more encumbered, as the whole image data must be sent to the recorder, while the factual recording happens only in case of movement. If the image processing of the camera is implemented with a decentralized method, the camera includes intelligent software, and the analysis happens before the forwarding of the image data. Therefore, the need for bandwidth decreases, the network is not encumbered very much, and the needed storage space is reduced, as data is sent only when there is an event happening[14]. As a second step, the cameras should be programmed to recognize which subject is taught in which classroom. This would be easily achieved by entering the time-table. The list of subjects taught may be defined in the cameras’ database. This data may be expanded with the list of lecturers and teachers, to send them the attendance register from the camera system. If needed, even the mugshots may be forwarded with the attendance register in predefined time intervals to a given IP address. As a further step, the list of students, along with their mugshots could be recorded. The camera would recognize the students and could compose an attendance register with the date and time of attending the classes. The system’s complete operation is shown in Figure 1:
THE MOST IMPORTANT STAGES OF AN ATTENDANCE REGISTER COMPOSING CAMERA SYSTEM’S DESIGNING PROCESS
Before the practical realization, even during the phase of designing, numerous difficulties and problems emerged. The up-to-date cameras feature high pixel density, so they generate oversized data quantities. Thus, it is expedient to choose the best possible video compressing solution. These days, the most used compression procedure is the MJPEG, the H.264, and the H.265 standards. To achieve a successful establishment of an attendance register composing camera system at universities, it is advised to deploy a security camera in front of each auditorium. In the case of larger educational institutions, this means numerous cameras implicitly. Therefore, during the building up of the system, it is expedient to foresee a deployment of 24 cameras. Table 1 shows the primary computation of the data quantity, generated by the attendance register composing camera system consisting of 24 cameras.
As it can be seen, from the computation in the present table, the number of days spent with education has been strictly observed, as the lectures on the faculties usually take place in a time period from Mondays to Fridays. Furthermore, the cameras presumably record 12 hours a day at best, so data has been taken accordingly. Based on the preliminary computation, it may be stated that the implementation of the codec H.265 seems to be the best solution.
THE CHOICE OF THE SECURITY CAMERAS BASED ON MEGAPIXELS
These days, the most common security cameras feature resolutions of about 1,3MP to 10MP. The higher the resolution of a camera, the more details its recording conveys. For educational institutions, the mentioned progress may mean both advances and drawbacks. The more precise identification and zoom-in capability with a minimal decay in picture quality, through a greater resolution, would mean an advantage. The overly great quantity of generated data may mean a hindrance, because of its storage and processing representing a serious task for the NVR (Network Video Recorder) unit, and for the security cameras. Therefore, during the design, 3 types of cameras were chosen, all participating in the testing of the online attendance register composing system. These were cameras featuring 1,3 MP-s, 2 MP-s, and the most modern one with 10MP-s of resolution. The mentioned camera types were included because of the significant differences in resolution. While the camera featuring 1,3MP-s of resolution is characterized by cost-effectiveness, the camera capable of 10MP-s of resolution represents the absolute pinnacle of development. The cost-effectiveness, or rather the best price/value rate is recommended to be observed in the case of universities. So, before the tests began, three camera types had been chosen. All of these utilized the compression process of standard H.265, in favor of lessening of the data quantity in question.
EXPERIENCES GAINED DURING THE REALIZATION IN PRACTICE
The practical testing was completed in a period of six weeks. Each camera type had an allotted testing period of 2 weeks. The cameras all possessed artificial intelligence and could perform all important analytic functions, such as facial detection, facial recognition, headcount, and black and white-listing. The testing of the cameras included 57 university students, during the cold, winter months, with the testing of all the cameras subsequently. This was necessary, to avoid significant changes in dressing and hairdo of the persons in question, as the opposite would influence the efficiency of the cameras tasked with identification. If some of the cameras were tested in the winter, and some in the summer, the accuracy of the identification would suffer greater deviations, negatively influencing the exactness of the measuring. Therefore, the primary aim was the provision of nearly identical conditions. The facial recognition completed on the students was influenced by numerous negative factors. One of these was overlapping, as in case of many students, during the entering the lecturing hall, some individuals were overlapped by others. The identification was further encumbered by the fact that some students wore glasses or scarves, thereby covering some facial details which, if not covered, would aid in efficient identification. During the deployment of the cameras, special attention had to be directed towards the ambient light conditions. One of the cameras was deployed incorrectly, therefore the light conditions and the shadows greatly hampered the task of the artificial intelligence of the camera in question. In practice, this meant that the facial recognition capability of the camera deteriorated, and it was not capable of the efficient identification of the students. During the testing of the attendance register composing system, it was proven that the cameras featuring artificial intelligence need some time to learn. The longer a camera had been operating, the higher efficiency it achieved. The cameras’ efficiency in operation is shown in table 2.
Groups of resolution | Accurate identification of students during week 1 | Accurate identification of students during week 2 |
1,3 MP (HD) camera | 43 | 51 |
2 MP (HD) camera | 46 | 52 |
10 MP camera | 51 | 55 |
As it can be clearly seen, the cameras were operating more efficiently than during the week before. The cameras were learning continuously, so the efficiency of the recognition improved significantly by the end of the testing period. Based on the results of the research, it may be stated that the camera featuring a resolution of 1,3 MP-s, initially identified the students at a 75 % rate, while during the second week, it correctly identified them at an 89 % rate. The camera featuring a resolution of 2 MP-s, could initially reach an efficiency of 80 %, while during the next week it operated at the efficiency rate of 91 %. Last, but not least, the camera featuring a resolution of 10 MP-s could initially reach an efficiency of 89 %, improving gradually to 96 % in the end[15].
EMPIRICAL RESEARCH
During the empirical research, the lecturers’ opinions were examined, concerning the online attendance register composing system based on security cameras. During the examination of the empirical research, as typical means of measurement in pedagogy, inquiries were utilized. The received data were analyzed with the help of the program named SPSS.
HYPOTHESIS
It may be presumed that the lecturers are open to new technological solutions, so they would gladly utilize the online attendance register composing camera system during their lectures. The composition of the attendance register represents an important part of lecturers’ duties. The lecturers compose an attendance register of the students present on their lectures. Thus, it is expedient to automatize such a routine, daily task as part of a smart city. Following this concept, the teachers were asked if they found the new, attendance register composing solution useful in aiding their day-to-day activities. The received answers were presented in Figure 2.
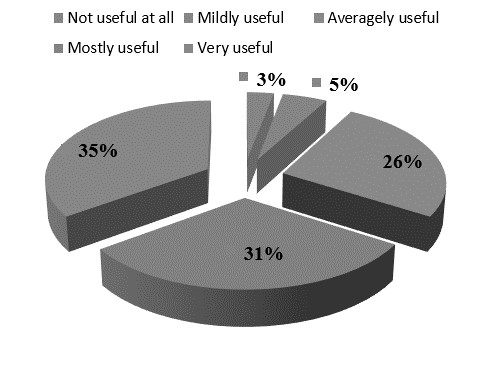
Figure 2. In your opinion, could a camera system capable of composing attendance registers be useful at the universities? (n = 70). The specificity of the educationalists’ work is that faculty lecturers continuously compose new, more modern lectures to be taught to their students. The lecturers’ work requires creativity and open-mindedness, so it may be presumed that the educationalists would gladly utilize an attendance register composing solution based on a camera system during their day-to-day activities. Answering the question, about having the possibility of utilizing an attendance register composing system based on a security camera system, or a paper-based solution, the majority of the lecturers stated that they would utilize the first possibility (p = 0,075), independently from universities. The received answers are shown in table 4 .
Based on the teachers’ answers, it may be stated that the hypothesis has been proven, by which, the teachers are open towards new technical solutions, so they would gladly utilize in practice the camera system composing online attendance register during their lectures.
CONCLUSION
A sufficient level of safety in the educational institutions of smart cities cannot be reached without modern security cameras. To lessen the administrative encumberment of lecturers, it is advisable to equip the camera systems with the function of composing an attendance register. Thus, the existing level of safety may be increased, and very broadly speaking, the paper-based attendance registries could be replaced with automated digital attendance registries. To achieve this, the camera system must feature a deep learning function. Based on a substantial quantity of metadata, the system could learn to recognize the errors of identification arising from the encumberments, thus, a type of reliable and efficient camera system could be attained, with the point of recognizing the students with the fewest errors possible, as well as sending the attendance register to a predefined, teachers’ and lecturers’ email address periodically. The research uncovered that through artificial intelligence, the cameras were learning continuously. It may be stated that by the end of the testing period, the cameras’ efficiency had improved significantly, concerning the identification of students. As part of the empirical research, it was determined that the teachers, included in the present research, may utilize a camera system composing online attendance registers in practice if the possibility were given.