INTRODUCTION - Uvod
Throughout human existence, forest ecosystems have been a source of numerous important natural resources. For example, humans have long been taking advantage of these resources for their own benefit. While timber is the primary forest resource utilized by humans, non-timber forest products (NTFPs) also play a crucial role in providing numerous benefits to people. These products refer to all plant and tree parts, such as shrubs, and any other types of plants harvested for commercial or non-commercial purposes within forest ecosystems. NTFPs offer a wide range of benefits to humans (Leakey et al., 1996). Recently, the significance of non-timber forest products (NTFPs) has gained more prominence and their popularity has increased within forest ecosystems. As a result of intensive usage due to the increasing global population, non-timber forest products (NTFPs) in forests become even more functional. Therefore, studies related to the ecology of non-timber forest products (NTFPs) have gained considerable importance in recent years, as well as in different disciplines (Farnsworth et al., 1985; Demirci, 2011; Ludvig et al., 2016; Enescu, 2017; Lovric et al., 2020; Muttilainen and Vilko, 2022).
The World Health Organization (WHO) has reported that when approximately 4 billion people around the world encounter any health problems, they first try to solve them with herbal drugs. It has also been stated that approximately 25% of the active ingredients in the most used prescription drugs in developed countries are of plant origin (Farnsworth et al., 1985). The significance of non-timber forest products (NTFPs) becomes evident when looking at their use in the food industry, medicine and pharmacy, and cosmetic industry. Furthermore, it is evident from import and export figures worldwide that non-timber forest products (NTFPs) provide significant financial benefits to countries and people (Yuan, 2022; Barbu and Tudor, 2022).
It has been stated that thyme, bay leaves, cumin, anise, fennel seeds, juniper bark, mahlab, fenugreek, rosemary, licorice root, mint, sumac, sage, and linden flower are among the products that Türkiye exports the most (Kurt et al., 2016). The economic and medicinal significance of these species increases the demand for them. Indeed, the importance of preserving these species in their natural habitats and maintaining their existence is crucial for meeting the demand for these products smoothly. Ensuring sustainable supply to meet the demand for non-timber forest products requires the effective preservation and maintenance of these species in their natural habitats. Therefore, it is crucial to accurately identify the ecological requirements of these species in their natural environments to meet the growing demand. Species distribution modeling is considered the most effective approach for achieving this objective, and various modeling techniques have been developed over time. Depending on the structure of the target species and the independent variables, it can be referred to as species distribution modeling, ecological niche modeling or habitat suitability modeling. These methods analyze the conditions of species under different ecological and climatic conditions, producing practical and effective results. In fact, at this point, it has become possible to simulate the obtained models in the past or future. Therefore, the popularity of the aforementioned methods has increased day by day (Thuiller et al., 2003). In recent years, many studies have been conducted using these methods to analyze aquatic organisms (Drake and Bossenbroek, 2004; Sousa et al., 2006; Herborg et al., 2007), wildlife species in forests (Martinez et al., 2003; Lopez-Lopez et al., 2007; Morelle and Lejeune, 2012; Süel, 2019), and plant species (Munoz and Felicisimo, 2004; Liu et al., 2005; Marmion et al., 2009; Gülsoy et al., 2017; Karataş et al., 2019; Şentürk et al., 2019). Various modeling techniques have contributed to the ecological knowledge of countless other species in nature except for these basic groups of organisms. In this regard, there has been a significant increase in modeling studies on non-timber forest products in recent years (Özdemir and Özkan, 2016; Karataş et al., 2019; Karakaya and Yücel, 2021).
In Türkiye, forest ecosystems cover approximately 23.1 million hectares, which corresponds to approximately 29.5% of the total country area (Vatandaşlar et al., 2023). A significant portion of Türkiye's existing forest cover is located in the southwestern Anatolian region. In addition, it should be noted that the Fethiye region, which is the subject of the study, is also included in this geographical area. The forests of the Fethiye region, where primary forest tree species such as pine, cedar, and juniper dominate, also harbor numerous plant taxa and endemic species (Tuzlacı, 2002). In the forested areas of the region where species diversity is rich, the distribution and utilization of non-timber forest products by the local community are significantly high. It is known that many species are gathered, particularly by the local community, for medicinal purposes. It has been observed that the community gathers these species both to meet their own needs and for commercial trade (Gurdal and Tunç, 2020).
This is especially the case for the species investigated in the study, Pistacia terebinthus L., Origanum onites L., and Arbutus andrachne L., which are prominent species in the region. Some studies conducted on Pistacia terebinthus L. have indicated various properties of this species. Topçu et al. (2007) reported that Pistacia species contain flavonoids and other phenolic compounds, which confer antimicrobial, antioxidant, anti-inflammatory, and cytotoxic activities. Kıvçak et al. (2004) found significant amounts of volatile oil and components in the leaves and young shoots of the species. Flamini et al. (2004) noted varying levels of volatile oil and components in the species' raw and ripe fruits. Studies conducted in different regions and climate conditions have also highlighted the presence of varying proportions of volatile oils and important components in various organs of the species, such as leaves, fruits, and shoots (Dhifi et al., 2012; Ulukanlı et al., 2014; Pulaj et al., 2016; Piras et al., 2017; İnan, 2021). In studies conducted on the species O. onites L., Tanker and Tanker (1990) identified the major components of this species as carvacrol and thymol. They observed that carvacrol is always in liquid state, whereas thymol has crystallizable properties. Furthermore, they highlighted that thymol is widely used in the pharmaceutical industry and finds significant application in the cosmetic and perfumery industries due to its positive effects on skin treatment. Cingi et al. (1991) reported that thyme has analgesic and muscle relaxant effects due to its pharmacological and toxicological properties. Similar studies on O. onites L. conducted under different regional and climatic conditions have indicated variations in the proportions of volatile oil and components depending on the region, collection techniques, collection timing, and even the time of day when the plant is harvested. These studies have also demonstrated that the species exhibits various biological activities, including antioxidant, antimicrobial, larvicidal, insecticidal, anticancer, acaricidal, antigenotoxic, hepatoprotective, antiviral, antidiabetic, anti-inflammatory, cardiopulmonary, cholinesterase inhibitor, analgesic, and fumigant toxicities. Particularly, the main components, carvacrol and thymol, have shown positive results in cancer treatments, and due to their antibacterial and anti-inflammatory properties, O. onites L. is commonly used for conditions such as arthritis, muscle and joint pain, skin irritations, infections, diarrhea, stomach disorders, and digestive problems (Başer, 2001; Oflaz, 2001; Yaldız et al., 2005; Ayanoğlu et al., 2006; Toncer et al., 2009; Tepe et al., 2016; Yıldırım and Kutlu, 2016). Lastly, research conducted on A. andrachne L. has revealed significant health effects of the species' phenolic components, including their antimicrobial, hypotensive (blood pressure-lowering), antipyretic (fever-reducing), laxative for the stomach and intestines, and liver decongestant properties (Dingil, 1990). It has also been noted that A. andrachne L. possesses diuretic properties (Şeker and Toplu, 2010) and exhibits antibacterial, antioxidant, antimicrobial, and excellent phenol source characteristics, which can be used against various diseases (Serçe et al., 2010; Abidi et al., 2016; Saral et al., 2017; Baskan et al., 2019).
In accordance with the mentioned reasons, potential distribution modeling was carried out for economically valuable species including P. terebinthus L., O. onites L., and A. andrachne L. in the Fethiye-Babadağ region. Despite the fact that the study area hosts a significant number of plant species that have the potential to be used as non-timber forest products, there have been no previous studies conducted on the distribution models of these products. The species included in the study are among the important ones that have a wide distribution in the region. By revealing the potential distribution areas of these species and promoting their dissemination and expansion, it will be possible to establish the foundation for more effective utilization and sustainability of these species. Moreover, it is believed that the results obtained from this study will contribute to determining the non-timber forest product potential of the region.
MATERIAL AND METHODS – Materijali i metode rada
Study area – Područje istraživanja
The study was conducted in Babadağ and its surroundings, located within the boundaries of the Fethiye district in the Muğla province. The study area selected, Babadağ and its surroundings, covers an area of approximately 44,000 hectares (Figure 1). The reason why this area was chosen as the study area is because it is one of the 9 hot spots in Türkiye. The area is located in a very important position with this feature. The elevation range of the area extends from sea level at 0 meters to mountainous areas at an altitude of 1950 meters. The western side of Babadağ consists of very steep slopes that extend all the way down to the sea. The upper parts of the southern side consist of steep slopes, while the lower parts are less sloping. The northern side of the mountain exhibits a similar topography to the southern side. Forests represented by Pinus brutia cover the altitude range of 0-1000 meters and are followed by Pinus nigra and Cedrus libani above this zone. The uppermost zone is characterized by a mixture of Juniperus species and cushion-forming plant communities with thorns (Kırmacı and Ağcagil, 2018).
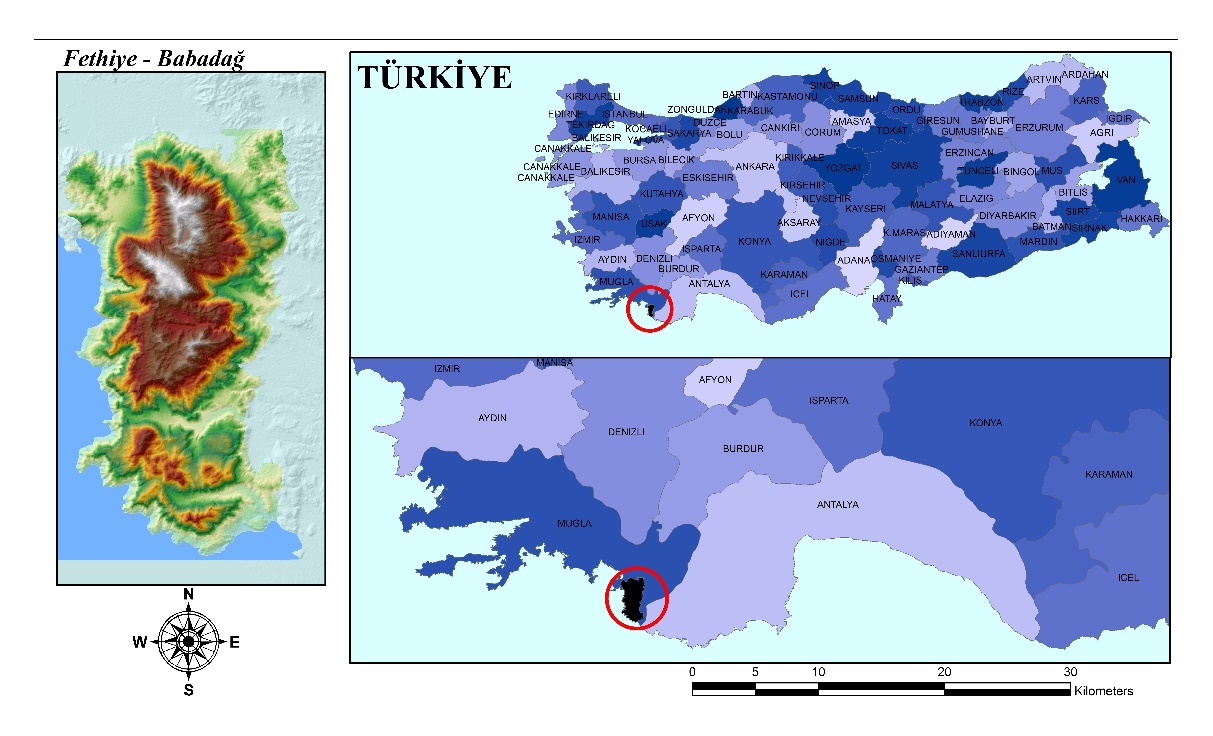
Figure 1. The location map of the study area
Slika 1. Karta lokacije područja istraživanja
As previously mentioned, the economic and commercial importance of A. andrachne L., O. onites L., and P. terebinthus L. has been the subject of this study within the study area. A. andrachne L. is a species that generally occurs between 0 and 800 m altitude in the Eastern Mediterranean region (Stevens, 1978). The species O. onites L. is an Eastern Mediterranean element and is generally distributed in the elevation range from 0 to 1400 meters (Tepe et al., 2016). The species P. terebinthus L. is found in the western and southern regions of Türkiye, as well as in certain parts of Anatolia. Its distribution is generally in the altitude range of 0-750 m. (Yaltırık, 1967). The three species studied in this research have various recognized uses worldwide. Remarkable studies have been conducted on the health effects of the secondary metabolites contained in them (Abidi et al., 2016; Tepe et al., 2016; Piras et al., 2017; Baskan et al., 2019; İnan, 2021).
Method - Metode
As previously stated, the research area spans 44,000 hectares. As a result, this area included a few villages and agricultural land. In these places, no sampling was carried out. To guarantee a homogenous distribution, the remaining research area was visited for this purpose. Consequently, there were 204 sample regions. Inventory and 100 m × 100 m sampling were made in each sample area. In the inventory studies, data on the presence of three non-timber forest products of economic importance in the area were recorded. These species are A. andrachne L., O. onites L. and, P. terebinthus L.
After the fieldwork was completed, digital base maps of environmental variables were created in order to model the target species and determine their potential distribution areas and maps. Elevation, slope, heat index, radiation index, ruggedness index, roughness index, and hillshade index maps of the region were obtained. In addition, bio-climatic maps providing information on the climate of the study area were downloaded from the Chelsa Climate database (https://chelsa-climate.org/) (Riley et al., 1999; Gallant, 2000; Evans et al., 2014). During the modeling process of the potential distribution areas of the species in the study area, 10 environmental variables and 19 climate variables included in the study were assigned abbreviation codes and recorded in digital format (Table 1).
Table 1. The abbreviations used for the environmental variables and climate data of the study area for statistical analysis
Tablica 1. Kratice korištene za varijable okoliša i klimatske podatke područja istraživanja za statističku analizu
During the statistical analysis stage, Pearson’s correlation analysis was first applied among the environmental variables and factor analysis among the climate variables. The reason for these applications was to prevent the multicollinearity problems that might arise during modeling processes. During the statistical analysis phase, Pearson’s correlation analysis was first applied among the environmental variables, while factor analysis was applied among the climate variables to address potential issues of multicollinearity during the modeling process. As a result of Pearson’s correlation analysis, some variables with high correlation were eliminated and representative variables were identified for the eliminated ones. Furthermore, through factor analysis of the climate variables with high correlation, representative variables were selected which could effectively represent all climate data (Kano and Harada, 2000; Alin, 2010).
In the modeling stage, the maximum entropy approach, also known as the Maxent method, was used. The working principle of this method, which only uses presence data, is based on predicting suitable and unsuitable areas for the target species by identifying the ecological requirements of the species. MaxEnt method is a widely preferred approach for species distribution modeling. The reasons behind this include its ability to work with smaller sample areas compared to other methods, the ability to provide better results with less presence data, and high prediction accuracy (Obiakara et al., 2020; Özdemir et al., 2020; Hussein and Workeneh, 2021; Karakaya and Yücel, 2021).
Rezultati
In the study, Pearson’s correlation analysis was applied among the bio-climatic variables, and many of the climate variables were found to have a high correlation with each other at the level of p≥0.80.
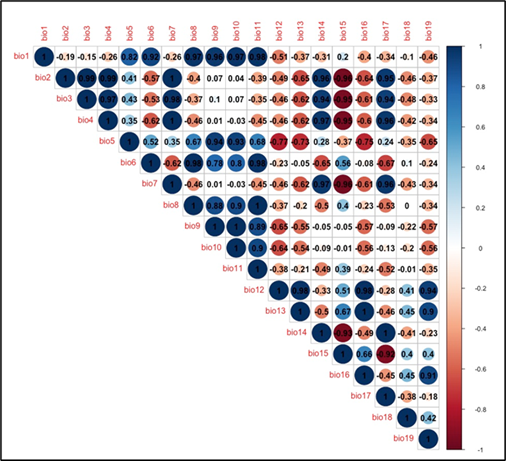
Figure 2. Correlation analysis results between climate variables
Slika 2. Rezultati korelacijske analize između klimatskih varijabli
During the modeling phase, representative variables were determined among the bio-climatic variables using factor analysis to avoid the models being affected by multicollinearity problems. According to the results of the factor analysis, the bio1 variable, which had the highest explanatory coefficient among the temperature variables, was chosen as the representative variable. Among the precipitation variables, bio15, which had the highest explanatory coefficient value, was selected as the representative variable. Once representative climatic variables were determined, correlation analyses were performed for each species separately to select the variables and proceed to the species distribution modeling stage.
Based on the analysis results, a correlation analysis using Pearson’s correlation coefficient was applied among 10 topographic and 2 climatic variables recorded in 18 areas for A. andrachne L. Variables showing a correlation of p≥0.80 with each other were eliminated. As a result, a significant correlation was observed between bio1 and elevation, between heat index and slope/radiation index, and between roughness index and slope/heat index at a p≥0.80 level. Therefore, elevation, heat index, and roughness index variables were not included in the model. As a result of the modeling process conducted with 9 independent variables, 4 variables have contributed to the model. The training AUC value of the most efficient MaxEnt model obtained was 0.954, and the test AUC value was 0.941. The jackknife plot and marginal response curves of the variables that constitute this model are presented in Figure 3 and Figure 4, respectively.
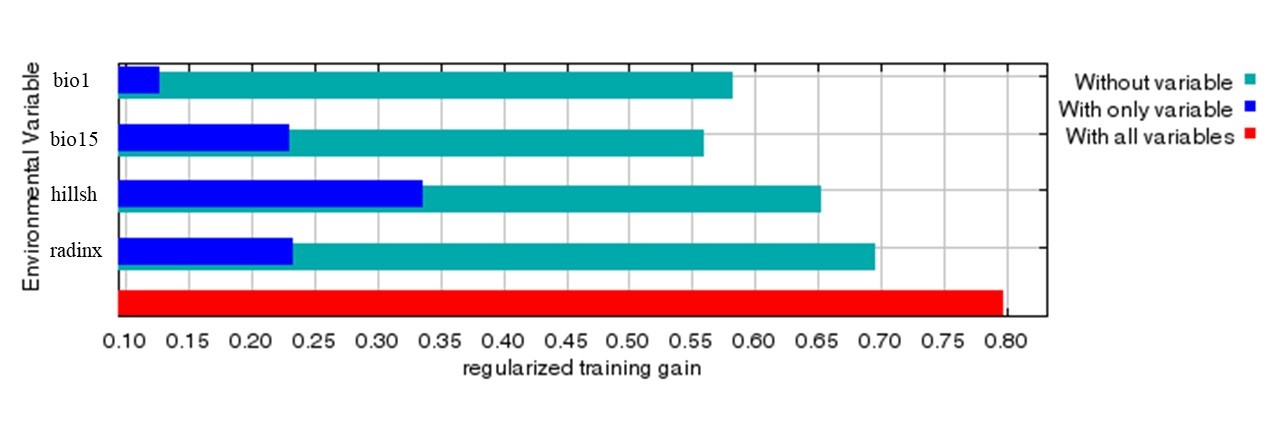
Figure 3. Jackknife test results for variables of A. andrachne L.
Slika 3. Rezultati jackknife testa za varijable A. andrachne L.
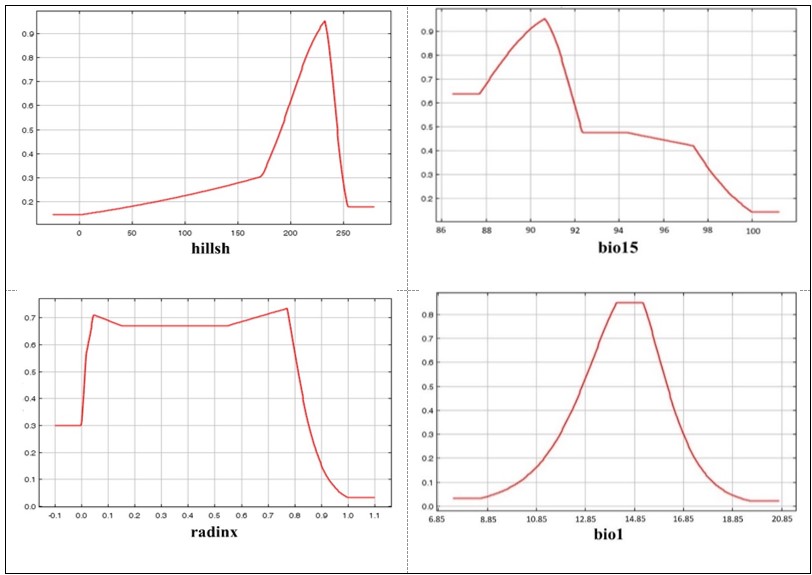
Figure 4. The marginal response curves of the variables that constitute the model for A. andrachne L.
Slika 4. Krivulje graničnog odgovora varijabli koje čine model za A. andrachne L.
The variables that shaped the model for the species, in order of their contribution rate, were hillshade index, bio15, radiation index, and bio1. The potential distribution map for the A. andrachne L. tree species in the study area was generated using the descriptive environmental variables obtained through the modeling process and it is presented in Figure 5.
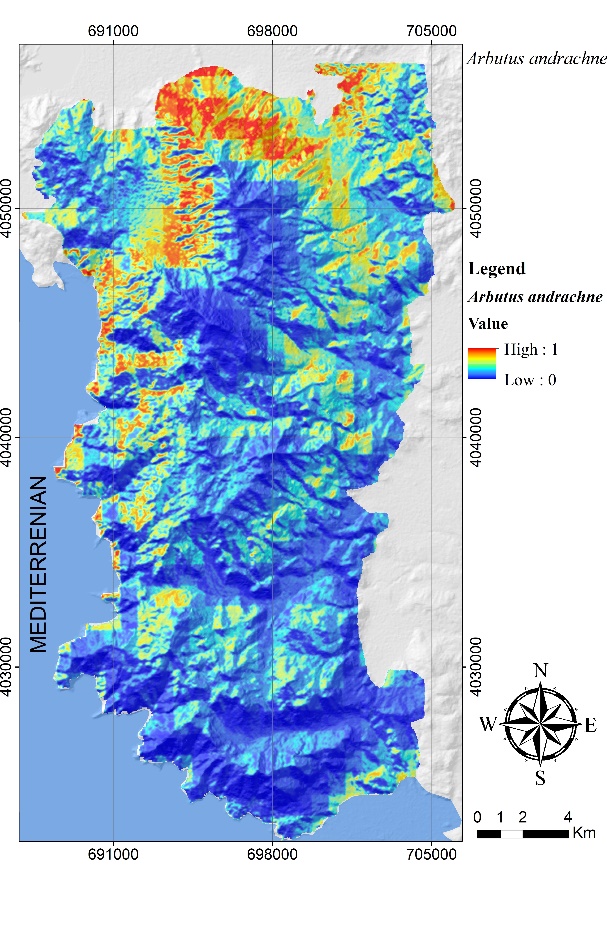
Figure 5. The potential distribution map for A. andrachne L.
Slika 5. Karta potencijalne distribucije za A. andrachne L.
Upon examining the potential distribution map of the species, it can be observed that the most suitable areas for the species are the partially western and predominantly northern slopes of Babadağ.
Pearson’s correlation analysis was conducted among the environmental variables of the 49 locations where O. onites L. species were observed in the study area. Therefore, elevation and roughness index variables were not included in the modeling stage. The model developed during the modeling process yielded a training AUC value of 0.822 and a test AUC value of 0.764. The jackknife graph and marginal response curves of the variables that constitute the model are presented in Figure 6 and Figure 7, respectively.
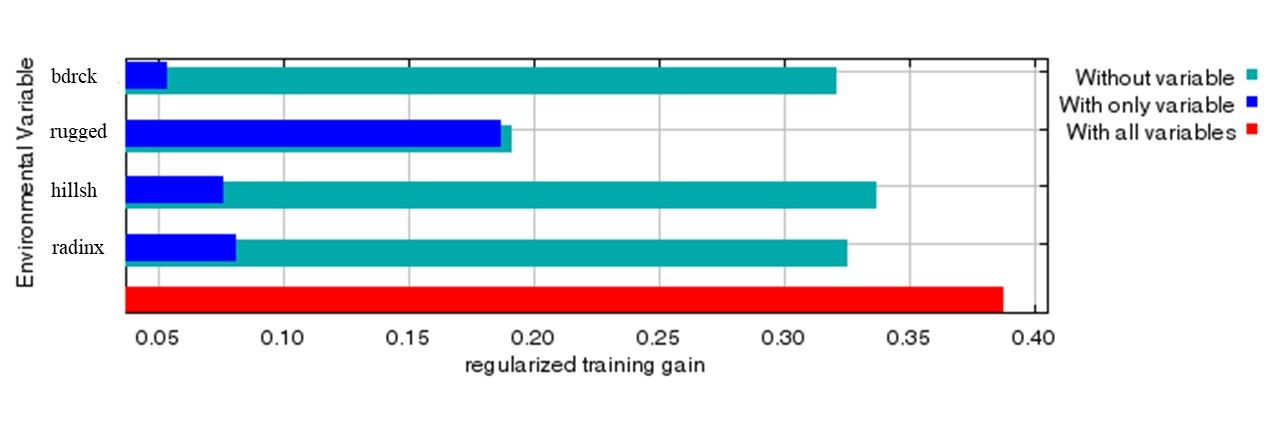
Figure 6. Jackknife test results for variables of O. onites L.
Slika 6. Rezultati jackknife testa za varijable O. onites L.
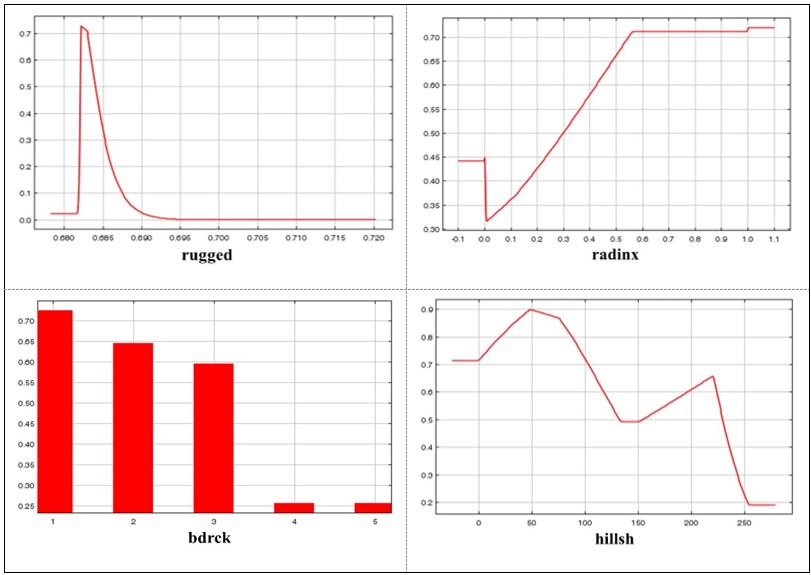
Figure 7. The marginal response curves of the variables that constitute the model for O. onites L.
Slika 7. Krivulje graničnog odgovora varijabli koje čine model za O. onites L.
The variables that contributed to the model in order of their importance were ruggedness index, radiation index, bedrock, and hillshade index. The potential distribution map for O. onites L. in the study area was generated using the descriptive environmental variables obtained from the modeling process and it is presented in Figure 8.
.
Figure 8. The potential distribution map for O. onites L.
Slika 8. Karta potencijalne distribucije za O. onites L.
Upon examining the potential distribution map of the species, it was determined that there are many suitable habitats for O. onites L. in several areas within the study area.
Finally, Pearson’s correlation analysis was applied to 11 topographic and 2 climatic variables for the areas where presence data of P. terebinthus L. species were recorded. The variables with a correlation coefficient of p≥0.80 were elevation and bio1. Therefore, the bio1 variable was not used in the modeling process. The resulting model had a training AUC value of 0.806 and a test AUC value of 0.774. The jackknife graph and marginal response curves of the variables that constitute the model are presented in Figure 9 and Figure 10, respectively.
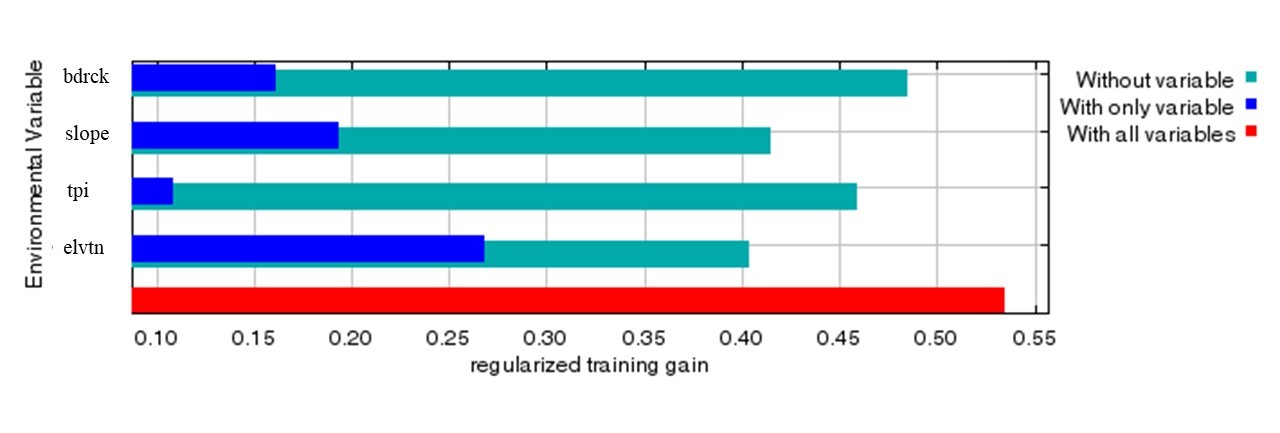
Figure 9. Jackknife test results for variables of P. terebinthus L.
Slika 9. Rezultati jackknife testa za varijable P. terebinthus L.
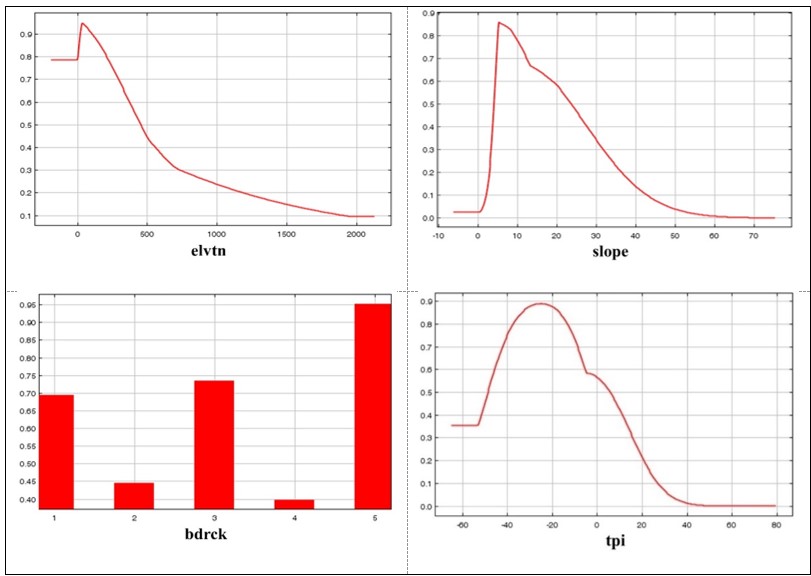
Figure 10. The marginal response curves of the variables that constitute the model for P. terebinthus L.
Slika 10. Krivulje graničnog odgovora varijabli koje čine model za P. terebinthus L.
It was determined that the variables contributing to the model in order of importance were elevation, slope, bedrock, and topographic position index. The potential distribution map for P. terebinthus L. in the study area was generated using the descriptive environmental variables obtained from the modeling process and it is presented in Figure 11.
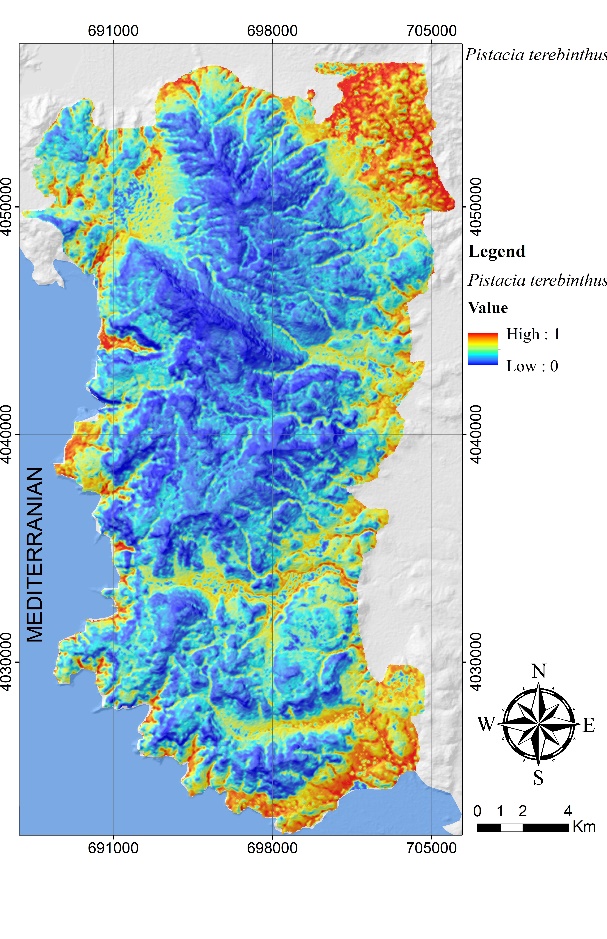
Figure 11. The potential distribution map for P. terebinthus L.
Slika 11. Karta potencijalne distribucije za P. terebinthus L.
Upon examining the potential distribution map of P. terebinthus L., it was determined that the most suitable areas for terebinth were those with low elevation near the coast and inland.
DISCUSSION - Rasprava
In particular, over the last 30 years, the rapid development of technology and medicine in parallel has led to the discovery of new uses for non-timber forest products of plant origin. Additionally, people's preference for avoiding chemical products has also contributed to an increase in demand for these products (Faydaoğlu and Sürücüoğlu, 2011). As a result, efforts to ensure the sustainability and widespread use of non-timber forest products (NTFPs) have increased day by day. The most important stage of the process is obtaining ecological information for the relevant species and processing this information to create a conservation and utilization component for species in their natural habitats. The most effective method for implementing this process is to convert the potential distribution areas of the species into model outputs and to create species distribution maps using the information obtained from these outputs. Based on this approach, the potential distribution areas of three important plant-based NTFP species that have both traditional and commercial use have been identified in the Fethiye-Babadağ region in this study. According to the classification of model performance, the model obtained for A. andrachne L. was rated as "excellent", while the models obtained for O. onites L. and P. terebinthus L. were rated as "good" (Swets, 1988).
In the scope of the study, first, the potential distribution modeling of A. andrachne L. was carried out, and its map was revealed. When the variable that contributes the most to the potential distribution of the species in the obtained model is examined, it can be seen that the values of the shading index correspond to the levels of 180-240. These values of the shading index represent the more shaded and north-facing areas of the region. When examining the variable contributions to the model, it can be observed that the bio15 variable has the second highest impact on the model. The potential areas of the species increase in regions where the average rainfall variability is around 90%. Another variable that significantly affects the distribution of the species is the radiation index, where negative effects on the species are observed when the value reaches 0.8. In terms of average temperature values, areas between 12-17°C are effective in the potential distribution of the species in the region. Previous studies on A. andrachne L. indicate that the species is distributed in the coastal areas influenced by the Mediterranean climate, particularly in the scrublands and Pinus brutia forests between 0-800 m. Additionally, it is suggested that the species prefers sunny and warm areas in terms of its ecological requirements, but it is also resilient to semi-shaded conditions (Stevens, 1978; Genç, 2007; Mamıkoğlu, 2010).
Another species that was subjected to potential distribution modeling within the scope of the study is O. onites L. When looking at the variables that contributed to the potential distribution model of O. onites L., it can be seen that suitable habitats for the species are formed in areas where the ruggedness index values are in the range of 0.681-0.684. This indicates that the species does not prefer steep areas that suddenly arise within the area. The result obtained from the radiation index curve indicates that the species does not prefer areas with values below 0.3. Areas with values in the range of 0.3-1.0 are potential areas for the species. In a study conducted by Özdemir and Özkan (2016) on modeling the potential distribution areas of O. onites L., they stated that radiation index was one of the most effective variables in the distribution of the species. When examining the contribution of another significant variable to the model, the bedrock type, it was revealed that alluvium, sandstone, and limestone parent rock types coded as 1-2-3 were suitable for the species. According to a study conducted by Gülsoy and Negiz (2014), soil and bedrock structure were found to be important factors in the distribution of the species, which is similar to the result obtained in this study, where bedrock type was identified as a significant variable. When examining the contribution of the last variable that contributed to the model, the hillshade index, it was determined that the species found suitable habitats in the levels between 0 and 240. Therefore, it was concluded that the species does not prefer highly shaded areas.
Another species that was modeled in the study is P. terebinthus L. Upon examination of the contributing variables in the model for the menengiç species, the potential distribution areas of the species were found to be within the elevation range of 0-500 m. In a study conducted by Gülsoy et al (2022), it was indicated that P. terebinthus L. was distributed in low elevations below 700 m. When the slope, another influential variable in identifying potential areas, was interpreted, it was observed that the potential areas of the species were located in areas with slopes of 25% or less. Another variable, the curve of the bedrock, indicated that alluvium (1), sandstone (3), and ophiolitic (5) bedrock types with code numbers 1, 3, and 5 respectively, were potential areas for the species. It was also noted in the study conducted by Gülsoy et al (2022) that the parent material and soil structure were effective in the distribution of the species. Finally, when the topographic position index curve was examined, it was found that the potential areas of the menengiç species were between the values of -45 and +5. The result obtained from this is that the species prefers flat and concave land surface forms.
Zaključak
In conclusion, NTFPs of plant origin, from which countries obtain significant financial benefits, have gained even more importance within the framework of ecosystem-based management and functional planning. In other words, it has become imperative to create management plans to prevent uncontrolled consumption and ensure continuity of NTFPs of plant origin. Increasing the distribution areas of species is also a strong management strategy that should be included in the management plans to be created. In the Fethiye region, the excessive and sometimes indiscriminate collection of NTFPs of plant origin by the local people makes it even more crucial to establish a management plan for the forest areas in this region. Furthermore, it is also important to protect the natural habitats of the species along with their forest stands. It is a fact that the potential areas where these species are abundant or can be found need to be protected against roads, marble quarries, or other types of structures. In addition, in silvicultural interventions to be carried out in these forest stands, the ecological requirements of the species should be taken into consideration.