Uvod
In forestry, measurement is a very important function. Measurement parameters are determined according to the desired analysis. Parameters are determined considering their positive or negative effects on ecosystems. They are classified according to ecological, economic, social, and technical criteria (Bjorklund, 2006; Gumus et al., 2008). The accuracy of the parameters that are measured may affect the performance of the analysis.
Parameters such as aspect, slope, elevation, digital terrain model, topographic moisture index and drought index are used for measurements in forestry (Kazama et al., 2021). These parameters are determined by the direct effect on the ecosystem, and they may affect human-oriented or environment-oriented activities. Forest road (FR) construction can be counted among human-oriented activities (Gumus, 2017; Akay et al., 2018). On the other hand, landslides (LSs) are a significant example of environment-oriented activity.
FRs are mainly involved in the production of wood raw materials and the protection of forests (Acar et al., 2003). Planning of FRs is a challenging process as both technical and environmental factors must be considered. The studies show that forest roads may have negative environmental impacts (Caliskan, 2013), such that they cause soil movements (Fransen et al., 2001), that forest areas are destroyed while constructing them (Kastridis, 2020), they divide ecosystems (Robinson et al., 2010), they reduce biodiversity (Boston, 2016). These are some important criteria while planning forest roads. Furthermore, some criteria are also considered in forest road planning such as construction cost, maintenance cost, slope, aspect, potential timber production and soil lithology (Kazama, 2021). Caliskan (2013) showed that geographical features such as slope and aspect both affect the construction and maintenance costs. Therefore, the measurement of geographical features becomes crucial.
LSs also affect the environment as well as FRs (Zumpano et al., 2018). Therefore, identifying the LS areas and determining the risk of LS has been of a significant interest in the literature. The studies show that various features must be considered while identifying LS areas such as geographical features, geological structure, geological composition, soil type, water content, water saturation, water drainage, and vegetation (Glade and Crozier, 2005). It is not sufficient to identify LS areas alone, but a risk assessment is also required. The studies suggest that geographical features, lithology, land cover, precipitation, and seismicity should be considered while assessing the risks of LS (Chalkias et al., 2014). These studies suggest that geographical features such as elevation (Feizizadeh et al., 2013), slope (Martinovic et al., 2016) and aspect (Hong et al., 2015) are very important for both identifying and assessing the LS areas (Pham et al., 2017).
With the spread of information technologies, analyses based on geographical information systems (GIS) have started to be carried out (Bugday, 2019). GIS-based studies are based on both objective and subjective parameters. Since these parameters are used in studies that employ impact analysis, multi-criteria decision-making and network analysis, the results of the studies can also be interpreted as subjective or objective (Caliskan et al., 2019). In these studies, aspect is one of the parameters that can be measured. Some researchers associate the aspect mainly with precipitation (Guzzetti et al., 1999), while others associate it with the general morphology of the region (Kavzoglu et al., 2014). According to many researchers, the aspect plays an equal role in LS formation to the slope (Chen et al., 2017; Chen et al., 2019). Climate, plant ecology, and vegetation are typically related to the aspect factor. The direction of the wind influences the amount of precipitation and the rate of erosion on sun-facing slopes, which are both influenced by aspect (Fan et al., 2017). Additionally, it has an impact on elements including temperature, soil moisture, wind, sunlight, rain direction, and air dryness (Kumar and Anbalagan, 2016). In three key ways, the aspect directly impacts the land. These are precipitation, sun, and wind.
Alexakis et al. (2014) and Kouli et al. (2014) determined that the northeast and northwest-facing slopes receive heavy rainfall. The slopes with the most precipitation were accepted as the most dangerous, and the slopes with the least precipitation were accepted as the least dangerous (Ayalew and Yamagishi, 2005). Slopes that receive precipitation reach soil saturation more quickly and cause higher water pressure on the ground (Chen et al., 2017).
Aspect directly affects the amount of energy received from the sun and therefore indirectly affects the water loss through transpiration and evaporation (Rahman et al., 2017). The slopes most exposed to sunlight show evapotranspiration (Hadji et al., 2016). This affects the moisture in the soil. Some researchers determined that LSs occur more intensely on slopes that are more exposed to sunlight (Hadji et al., 2016). In the literature, some studies determine that slopes that receive sun are more prone to LSs than slopes that receive rain. Wind controls soil moisture (Bui et al., 2012). Aspect also plays an important role in the evaluation of soil stability, because it controls the vegetation distribution, type, and density in a land (Chen et al., 2017; Ozsahin, 2015).
In Türkiye, south (S), southeast (SE), southwest (SW), and west (W) directions are often referred to as sunny directions because they are warmer. On the contrary, north (N), northeast (NE), northwest (NW), and east (E) aspects are also called shaded directions because they are cooler. The sun exposure times of these two groups differ significantly (Sessions, 2007; Hosseini et al., 2012; Caliskan and Karahalil, 2017). Evaporation is faster in the south and west aspects since these regions are more exposed to sunlight. Otherwise, since the evaporation is slow and the soil remains moist for a long time, the risk of flooding is higher on the north and east aspects in case of excessive rainfall (Yılmaz, 2008). Ozsahin (2015) determined that the probability of LS is highest in the north and west aspects in their study and stated that moisture is relatively higher on the slopes facing these aspects.
Considering the aspect factor in terms of FRs and LS areas, 10 studies (16.1%) were included in 3 databases (Scopus, Science Direct and Web of Science) over 62 articles between the years 2009 and 2019 (Kazama et al., 2021). The land aspect affects factors such as exposure to sunlight, precipitation, and winds that dehumidify the soil. In the northern hemisphere, low precipitation, high wind speed, intense solar radiation and high potential evapotranspiration are seen in southern aspects. Therefore, it is assumed that there is less damage to the surface of the land depending on the water concentration. The aspect of LS areas and FRs is one of the variables that affect forest soil (Sessions, 2007; Hosseini et al., 2012; Caliskan and Karahalil 2017). In the study, Cellek (2021) reported that LSs are more commonly seen in the southern aspects than in the northern aspects, and that there is an inverse relationship between them. If a LS occurs more in the southern aspect, it is associated with sun exposure, drought, and lack of vegetation. If an LS occurs more in the northerly aspects, it is mostly related to heavy rainfall, humidity and the water holding capacity of the soil. In the same study, it was stated that the aspect determinations differ for various researchers. The fact that these determinations are ambiguous causes the scoring to be done very differently. For all these reasons, the aspect factor in LS areas and FRs are scored on a user basis. In general, southern aspects are given higher scores, while northern aspects are given lower user-based scores (Table 1).
Table 1. Aspect scores for direction according to the studies in the literature. NS stands for Not Specified as the studies did not mention the corresponding directions. We assigned the scores according to the assumptions in the corresponding studies. (N = North, NE = Northeast, E = East, SE = Southeast, S = South, SW = Southwest, W = West and NW = Northwest)
Tablica 1. Ocjenjivanje orijentacije prema smjeru prema istraživanjima u literaturi. NS je kratica za Not Specified jer istraživanja nisu spominjala odgovarajuće upute. Ocjene smo dodijelili prema pretpostavkama u odgovarajućim istraživanjima. (N = sjever, NE = sjeveroistok, E = istok, SE = jugoistok, S = jug, SW = jugozapad, W = zapad i NW = sjeverozapad)
Acar et al. (2007), Gumus et al. (2008), Kayastha et al. (2013) and Bugday (2019) gave the highest scores for the S aspect and the lowest scores for the N aspect in their study. On the contrary, Abay et al. (2019) gave maximum points to the SE aspect and minimum points to the W aspect. Bugday and Akay (2019) gave maximum points to the W aspect and minimum points to the SE aspect. Chiteculo et al. (2022) gave maximum points to the NW aspect and minimum points to the SE aspect. Panchal and Shrivastava (2022) gave maximum points to the SE aspect and minimum points to the NW aspect. It should be noted that one of these studies is about LSs, and the other is about FRs. Arabameri et al. (2020) gave a maximum score to the S aspect and a minimum score to the E aspect in their study. Sathyan et al. (2022) gave maximum points to the W aspect and minimum points to the E aspect in their study. The details on how the above- mentioned studies scored the aspect can be seen in Table 1. As it can be seen from the table, aspect scoring is subjective and ambiguous in the literature.
The original value of this work can be listed as follows:
It has been shown that the aspect can be measured objectively rather than being a subjective measurement.
While measuring the aspect, instead of a scoring based on direction determination, the angle value is measured to ensure so that it is closer to objectivity. The proposed method scores the aspect ranging between 0-1.
Evaluation of basic risk and plan parameters was provided by using an objective aspect measure on FRs and LS areas.
The method was presented to the end user as open source with the module developed in ArcMap software.
The aim of this study is to present an objective and unique aspect scoring method for FR plans and LS area analysis. Thus, in the decision-making methods to be applied for planning and analysis, the aspect score will move away from subjectivity.
Materijali i metode
Materials - Materijali
Maçka Forestry Enterprise (MFE), located within the boundaries of Trabzon Regional Directorate of Forestry in the Eastern Black Sea Region of Türkiye, has been identified as the research area. The research area is limited by the forest areas and FR network in the Maçka district, which is mapped in Figure 1. Factors that are effective in determining the area are suitability for functional forest management planning and the existence of areas with various aspects in the study region.
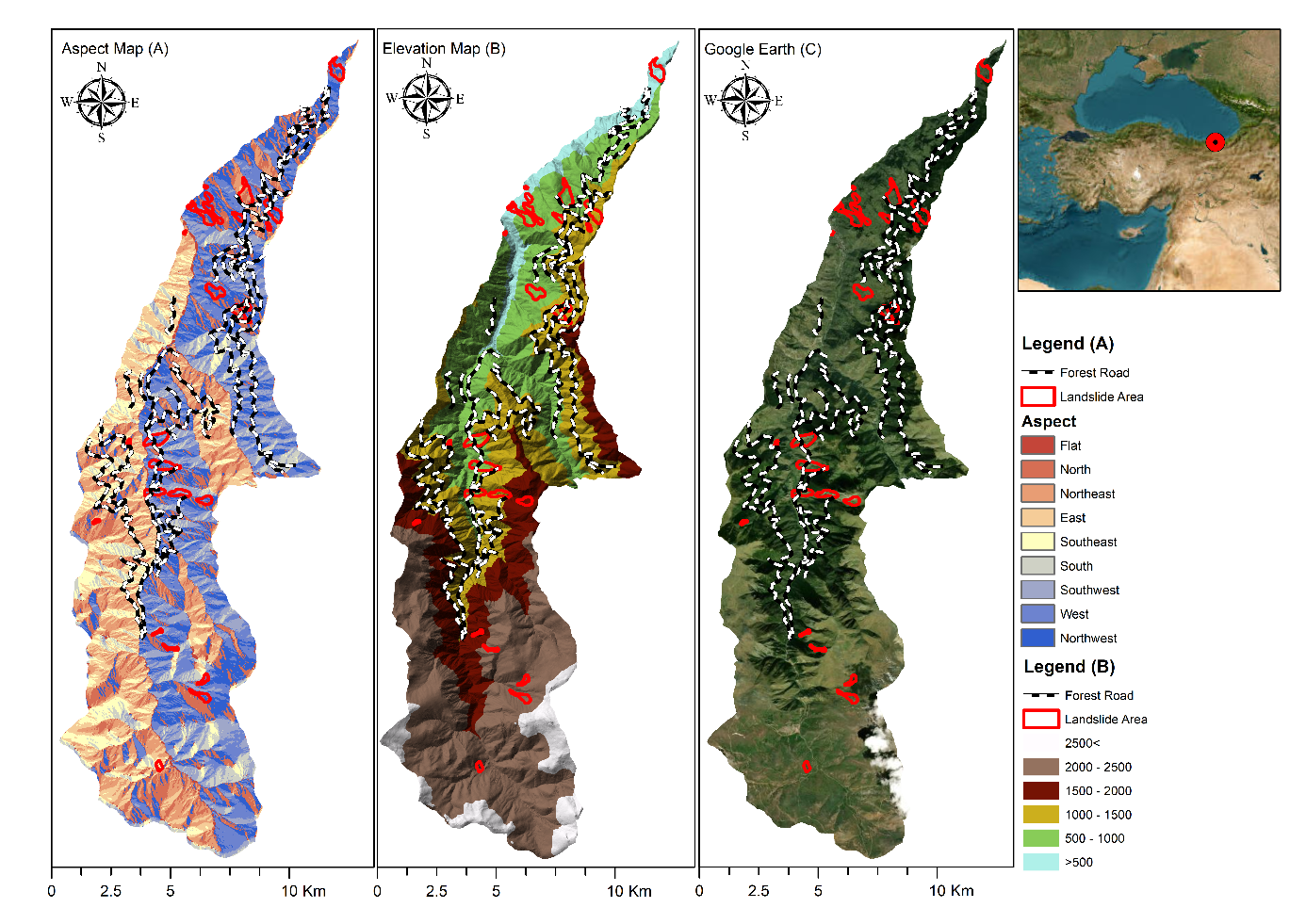
Figure 1. (A) Aspect map of MFE, (B) Elevation map of MFE, (C) Textured elevation map of MFE
Slika 1. (A) Karta orijentacije MFE-a, (B) Karta visine MFE-a, (C) Karta visine MFE-a s reljefom
The study area covers 18,170.80 ha, while 40.48% (7,360.40 ha) of the total area is forest area. There are 137.53 km of FRs in the existing road network plan (GDF, 2023). The highest elevation of the study area is 1820 m, and the lowest elevation is 130 m. The aspect distribution of the study area is as follows: 1.5% (276.43 ha) is flat, 13.6% (2,466.97 ha) is north aspect, 13.3% (2,368.41 ha) is northeast aspect, 11.8% (2,136.22 ha) is east aspect, 7.8% (1,411.59 ha) is southeast aspect, 6.3% (1,147.01 ha) south aspect, 12.1% (2,191.89 ha) southwest aspect, 17.7% (3,212.27 ha) west aspect, and 16.3% (2,959.28 ha) northwest aspect. The research region contains an LS area of 2.17% (394.29 ha) (GDMRE, 2023).
Methods - Metode
For the aspect score model, three elements were required: contour lines, study boundaries, and sample items for aspect score calculation. The model is illustrated in Figure 2.
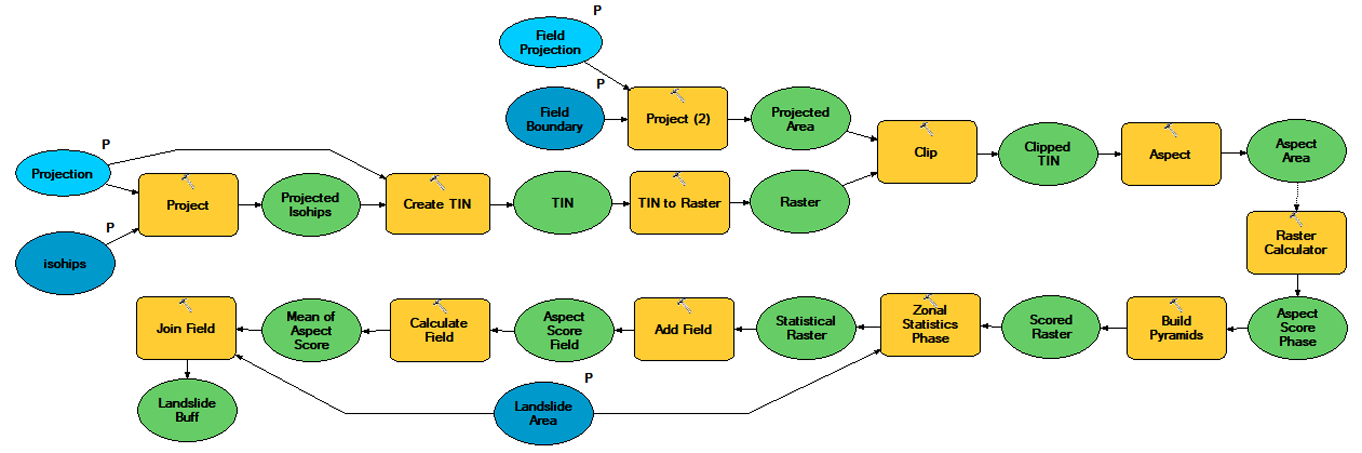
Figure 2. LS model for calculating the aspect score. This model was produced in the ArcGIS model builder.
Slika 2. Model klizišta za izračun ocjena orijentacije. Ovaj model izrađen je pomoću programa za kreiranje modela ArcGIS.
The map projection has been adjusted to ensure compatibility between layers. A Triangulated Irregular Network (TIN) was created from contour lines. Then raster was created over TIN. The study area was extracted from the raster which was created with contour lines, and the aspect function provided by ArcGIS was used. After the aspect was calculated, the aspect values were converted into aspect scores pixel-wise with a predetermined formula using the ArcGIS raster calculator tool.
Calculation of the Aspect Angle - Izračun kuta orijentacije
Assuming that the matrix given in Figure 3C is an elevation map, the first step to compute the aspect is to compute the gradients of the map in both directions x and y. In Figure 3, the vertical gradient mask (A) and the horizontal gradient mask (B) were given.
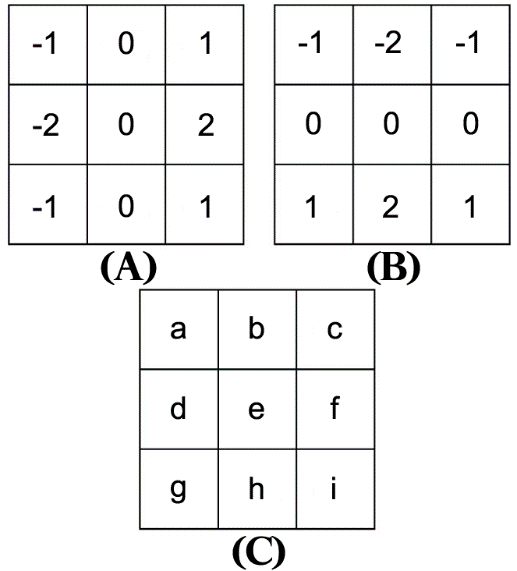
Figure 3. Masks for calculating the aspect: (A) vertical gradient mask, (B) horizontal gradient mask, and (C) elevation map example.
Slika 3. Predlošci za izračunavanje orijentacije: (A) predložak okomitog gradijenta, (B) predložak horizontalnog gradijenta i (C) primjer karte nadmorske visine.
For vertical gradient calculation, the elevation map was convolved with the mask given in Figure 3A. The convolution function is given in Equation 1.
in which z is the elevation map pixel corresponding to the pixel e, x is the vertical gradient operator, and a, b, c, d, e, f, g, h and i are the pixel values. Similarly, for horizontal gradient calculation, the elevation map was convolved with the mask given in Figure 3B. The convolution function is given in Equation 2.
in which z is the elevation map pixel corresponding to the pixel e, y is the horizontal gradient operator, a, b, c, d, e, f, g, h and i are the pixel values.
After calculations, two layers were obtained. Since these layers represent the horizontal and vertical gradients of the elevation map, the aspect can be obtained by computing the angles between the layers for each pixel. For this, Equation 3 was utilized. It should be noted that the aspect value in Equation 3 ranges from 0° to 360°.
Aspect Score - Ocjenjivanje orijentacije
Given that the aspect value in Equation 3 ranges from 0° to 360°, the aspect value is not sufficient for a multi-criteria decision-making analysis since the aspect value does not provide any suitability. Therefore, the studies in the literature gave their effort to define aspect scores. Aside from the studies being subjective when scoring aspects, they are contradictory as they differ in the way they score.
When looking deeper into the scoring procedure of the studies given in Table 1, it can be easily seen that when the authors scored a direction with a full score, they scored the opposite direction with a minimum score. Also, the studies classified the directions into eight main categories, and they lacked the sensitivity of the angles.
If it is assumed that a mathematical expression was built to eliminate the subjectivity of aspect scoring and increase the sensitivity of angles, this expression should accept inputs in the form of angles and provide aspect scores. Additionally, the opposing direction should be minimum when an aspect score of one direction is the highest. Therefore, an additional parameter should be set to define which direction should be full-scored. The aspect scores should lastly range from 0 to 1. Since nobody utilized any expression like this before, we suggest the expression given in Equation 4 after considering all the qualities above.
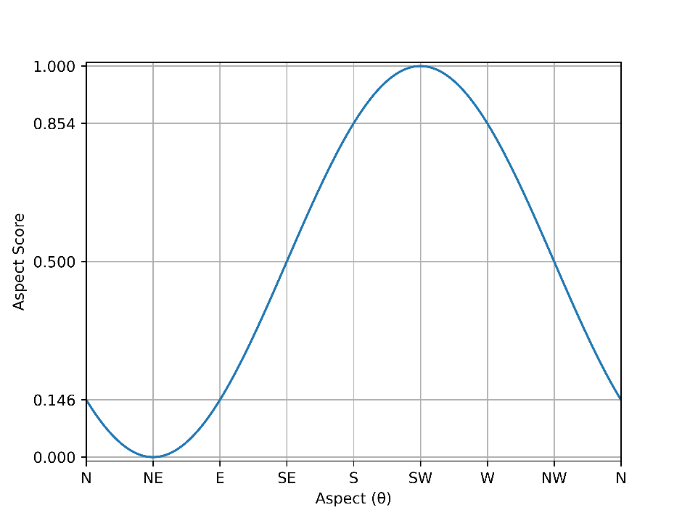
Figure 4. Graph showing the aspect scores given in the function where the southwest aspect is maximized, and the northeast aspect is minimized ( = 225°).
Slika 4. Grafikon prikazuje ocjene orijentacije dane u funkciji gdje je jugozapadna orijentacija maksimizirana, a sjeveroistočna orijentacija minimizirana ( = 225°).
In Equation 4, θ is the aspect value of the pixel, and ϕ is the direction angle which is desired to be fully scored. Note that the sin function is max-min normalized in order to make the expression range from 0-1. Figure 4 illustrates the aspect scores of directions when SW was full-scored, and NE was minimum-scored. The ϕ value in Equation 4 was chosen to be 225° since in our study we assumed that the SW aspect should be full-scored as suggested in the related literature mentioned above. It should be noted that our study area is located in the northern hemisphere.
Rezultati i diskusija
The model was designed in the ArcGIS module to score the aspect of FRs and LS areas. Considering the block diagram given in Figure 2, the aspect map shown in Figure 5(A) was obtained from the elevation map given in Figure 1(B). The mathematical expression of this procedure is detailed in Equations 1, 2 and 3. By applying this map to Equation 4, a new aspect score map given in Figure 5B was created. According to the equation, the pixel values of the new aspect score map range from 0 to 1. Thus, instead of making user-based scoring for the aspect value in the topographic structure of the area, an objective measurement value has been revealed. These objective values are shown with values close to 0 in more northern aspects (north, northwest, northeast and east), while values closer to 1 are shown in more southern aspects (south, southeast, southwest and west).
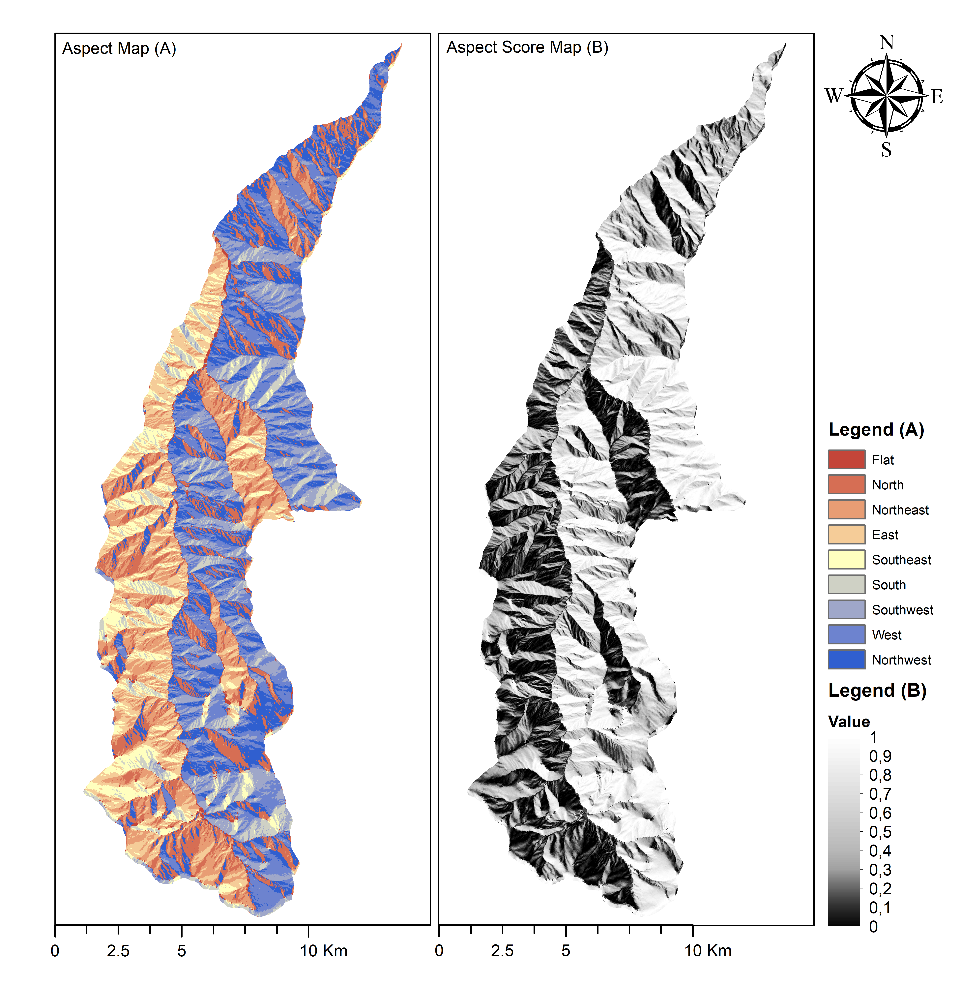
Figure 5. (A) Aspect map of MFA, (B) Aspect score map of MFA
Slika 5. (A) Karta orijentacije MFA-a, (B) Karta ocjena orijentacije MFA-a
Many studies have been carried out to score the aspects of FRs and LS areas. However, the papers reveal that the scoring methods differ for each study. Table 1 shows the studies which score aspect in FRs or LS areas and their scores for each direction. For a fair comparison, we min-max normalized the scores and showed them in Table 2. Table 2 reveals that each study has its own way of scoring aspect.
Table 2. Normalized aspect scores for direction according to the studies in the literature. Data are arranged from Table 1.
Tablica 2. Normalizirane ocjene orijentacije prema smjeru prema istraživanjima iz literature. Raspoređeni su podaci iz Tablice 1.
Study | The scope | N | NE | E | SE | S | SW | W | NW |
---|---|---|---|---|---|---|---|---|---|
Acar et al. (2007) | FR | 0 | 0 | 0.5 | 1 | 1 | 1 | 0.5 | 0 |
Gumus et al. (2008) | FR | 0 | 0 | 0.5 | 1 | 1 | 1 | 0.5 | 0 |
Kayastha et al. (2013) | LS | 0 | 0.23 | 0.62 | 0.71 | 1 | 0.81 | 0.59 | 0,1 |
Abay et al. (2019) | LS | 0.36 | 0.34 | 0.92 | 1 | 0.41 | 0.08 | 0 | 0.04 |
Bugday and Akay (2019) | LS | 0.25 | 0.25 | 0.25 | 0 | 0.25 | 0.5 | 1 | 0.5 |
Bugday (2019) | LS | 0 | 0 | 0 | 1 | 1 | 0.5 | 0.5 | 0 |
Arabameri et al. (2020) | LS | 0.06 | 0.25 | 0 | 0.5 | 1 | 0.75 | 0.19 | 0.31 |
Chiteculo et al. (2022) | FR | 1 | 0.75 | 0.75 | 0 | 0 | 0.5 | 0.75 | 1 |
Panchal and Shrivastava (2022) | LS | 0.04 | 0.52 | 0.15 | 1 | 0.28 | 0.14 | 0.04 | 0 |
Sathyan et al. (2022) | FR | 0 | 0 | 0.33 | 0.33 | 0.67 | 0.67 | 1 | 1 |
Table 3. For some of the selected FRs and LS areas, the normalized aspect scores according to the proposed study and the studies in the literature
Tablica 3. Za neka od odabranih područja FR i LS, normalizirane ocjene orijentacije prema predloženoj studiji i studijama u literaturi
Features |
Aspect by Sight (direction) |
Aspect by the study (degree) | Acar et al. (2007) | Gumus et al. (2008) | Kayastha et al. (2013) | Abay et al. (2019) | Bugday and Akay (2019) | Bugday (2019) | Arabameri et al. (2020) | Chiteculo et al. (2022) | Panchal and Shrivastava (2022) | Sathyan et al. (2022) | The study |
---|---|---|---|---|---|---|---|---|---|---|---|---|---|
FR-4380-020 | North | 27 | 0 | 0 | 0.06 | 0.12 | 0.25 | 0 | 0.07 | 1 | 0.05 | 0 | 0.42 |
FR-4380-022 | Northeast | 62 | 0 | 0 | 0.09 | 0.12 | 0.25 | 0 | 0.1 | 0.75 | 0.2 | 0 | 0.25 |
FR-4380-025-1 | West | 286 | 0.5 | 0.5 | 0.13 | 0.06 | 1 | 0.5 | 0.09 | 0.75 | 0.05 | 1 | 0.65 |
LS-26767 | Southwest | 270 | 1 | 1 | 0.16 | 0.07 | 0.5 | 0.5 | 0.18 | 0.5 | 0.08 | 0.67 | 0.73 |
LS-26768 | South | 280 | 1 | 1 | 0.19 | 0.13 | 0.25 | 1 | 0.22 | 0 | 0.13 | 0.67 | 0.72 |
LS-26772 | West | 300 | 0.5 | 0.5 | 0.13 | 0.06 | 1 | 0.5 | 0.09 | 0.75 | 0.05 | 1 | 0.6 |
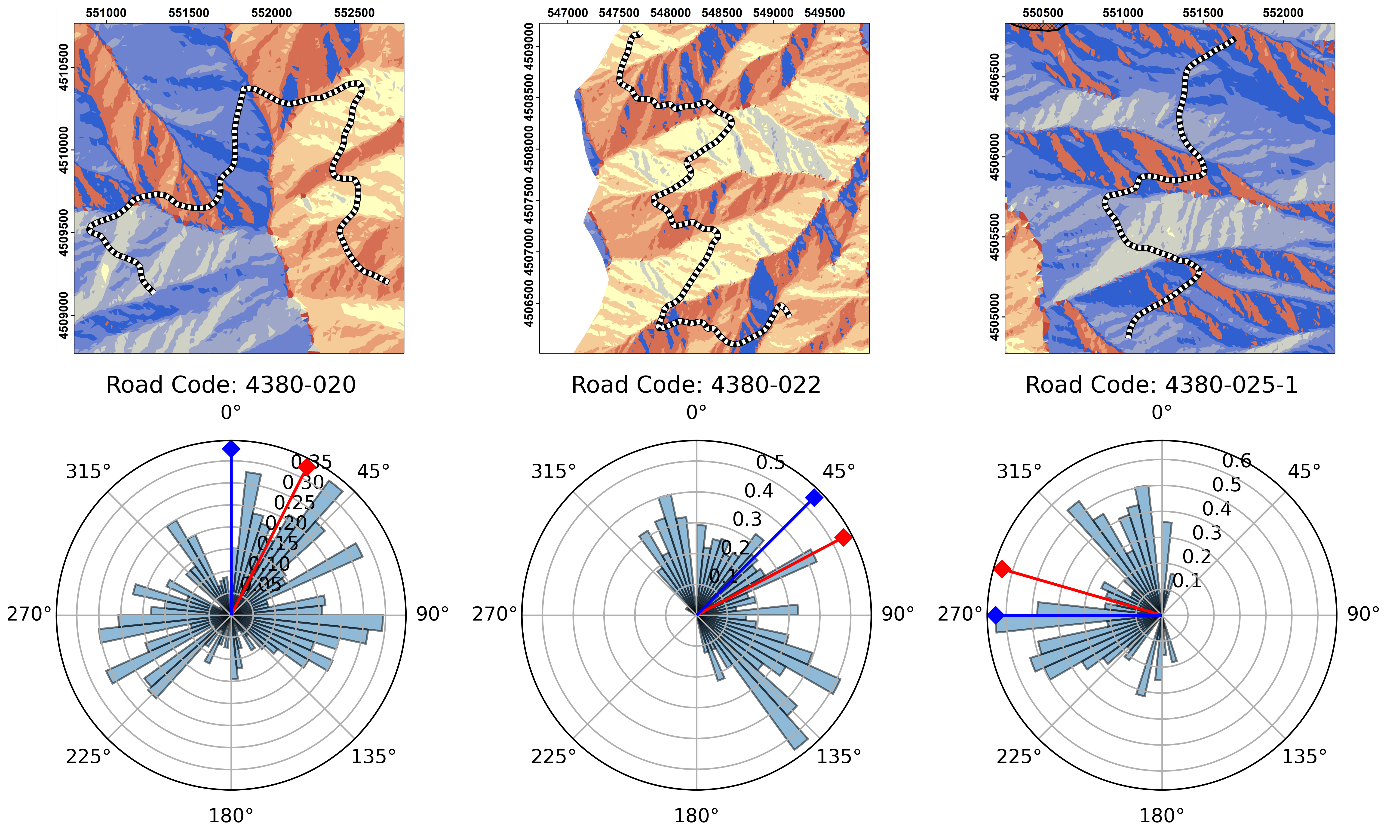
Figure 6. The aspect map of the FRs (top) and their corresponding pixel densities of aspect values (bottom). In the radar plot, the bar plot represents the density of the pixels in FRs. The blue stem represents the aspect of the FR when the aspect was determined by sight. The red stem represents the circular average of the pixel values.
Slika 6. Karta orijentacije šumskih cesta (gore) i njihove odgovarajuće gustoće piksela za vrijednosti orijentacije (dolje). U radarskom dijagramu, stupčasti dijagram predstavlja gustoću piksela u područjima šumskih cesta. Plava linija predstavlja orijentaciju šumske ceste kada je orijentacija određena vizualno. Crvena linija predstavlja kružni prosjek vrijednosti piksela.
When FR-4380-020, FR-4380-22 and FR43-025-1, which were selected as examples in FR data, were examined, the aspect values of the pixel values of the roads were revealed. Average aspect values based on pixels were 27°, 62° and 286°, respectively, as it can be seen in Figure 6. When FRs were examined, the pixel distributions corresponded to different aspects. When the aspect was evaluated according to the land surface, the FR aspects were north, northeast, and west, respectively. FRs coded FR-4380-020 and FR-4380-22 were in the north aspect. The FR coded FR4380-25-1 was on the south aspect. It is assumed that FRs will be scored low in northern aspects and high in southern aspects (Sessions, 2007; Hosseini et al., 2012; Caliskan and Karahalil, 2017). Aspect scoring was made by the equation determined based on this assumption. When the aspect values of FRs are applied using the equation, the scores obtained are 0.420, 0.253 and 0.645, respectively, as it can be seen in Table 3. As it can be seen in this scoring, the aspect of the roads gets closer to 1 as they get closer to the southern aspect. The evaluation of the selected FRs with other FRs is also shown in Table S1.
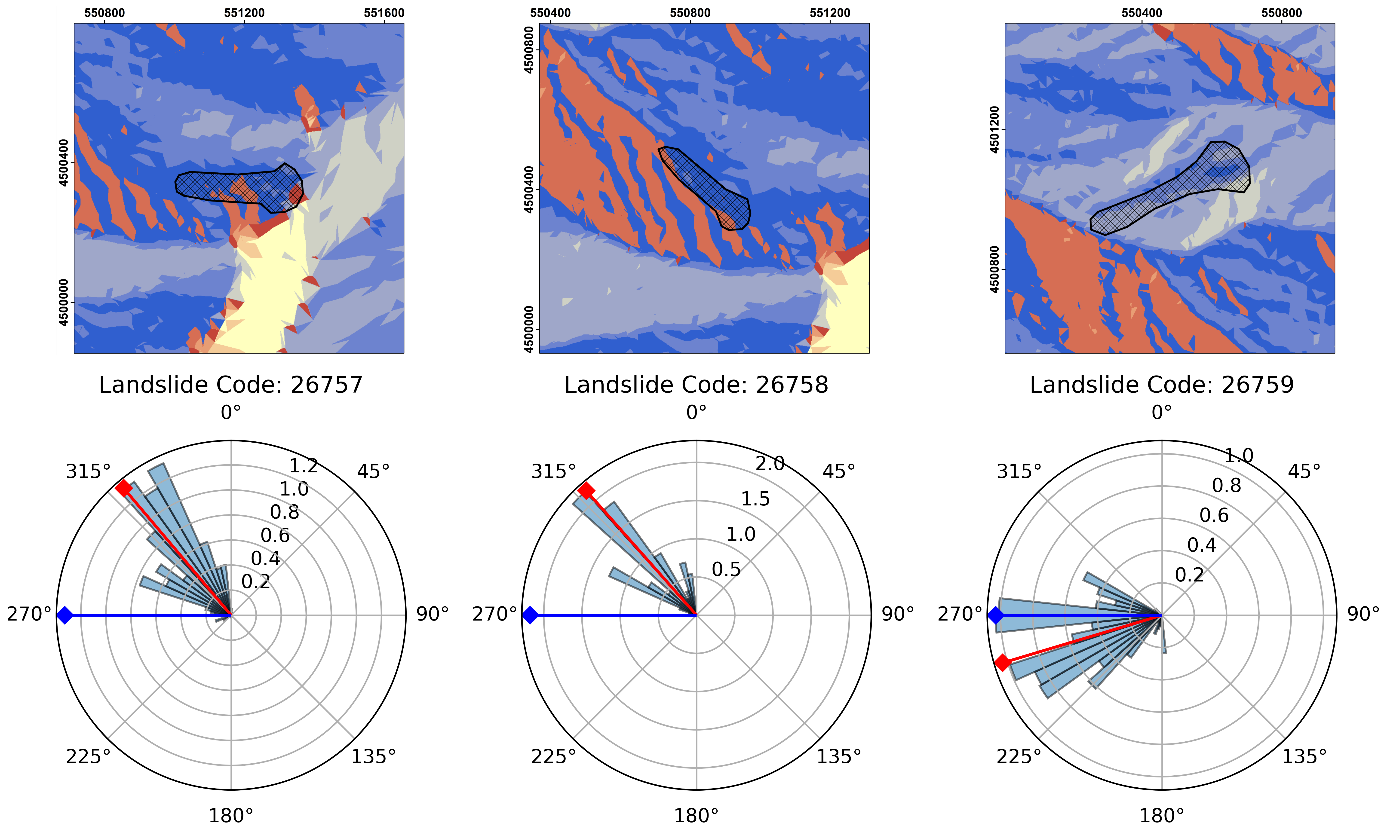
Figure 7. The aspect map of the LS areas (top) and their corresponding pixel densities of aspect values (bottom). In the radar plot, the bar plot represents the density of the pixels in LS areas. The blue stem represents the aspect of the LS area when the aspect was determined by sight. The red stem represents the circular average of the pixel values.
Slika 7. Karta orijentacije područja klizišta (gore) i njihove odgovarajuće gustoće piksela za vrijednosti orijentacije (dolje). U radarskom dijagramu, stupčasti dijagram predstavlja gustoću piksela u područjima klizišta. Plava linija predstavlja orijentaciju područja klizišta kada je orijentacija određena vizualno. Crvena linija predstavlja kružni prosjek vrijednosti piksela.
When LS-26767, LS-26768 and LS-26772, which were selected as examples in the LS data, were examined, the aspect values of the pixel values of the LS areas were revealed. Average aspect values based on pixels were 270°, 280° and 300°, respectively as it can be seen in Figure 7. When LS areas were examined, the pixel distributions corresponded to different aspects. When the aspect was evaluated according to the land surface, the LS area aspects were southwest, south, and west, respectively. Especially in LS-26772, there was a small difference between the pixel-wise view and the value observed on the land surface. Aspect scoring for LS areas were 0.733, 0.717 and 0.601, respectively as it can be seen in Table 3. Since these areas are on the west side, they should be scored higher. In LS-26767, although the number of pixels belonging to the northern aspect values is high, when the average aspect value is considered, it can be seen that it has a southern aspect. The evaluation of selected LS areas with other LS areas is also shown in Table S2.
For the forest road FR-4380-20, which is in the north aspect, while Chiteculo et al. (2022) gave a full score, Acar et al. (2007), Gumus et al. (2008), Sathyan et al. (2022) and Bugday and Akay (2019) scored it with 0, which is the lowest score. However, in our study, the score of the same FR was found to be 0.42. For LS-26768, which is in the south aspect, while Acar et al. (2007), Gumus et al. (2008), Sathyan et al. (2022) and Bugday and Akay (2019) gave it a full score, Chiteculo et al. (2022) scored it with 0, which is the lowest score. However, in our study, the score of the same FR was found to be 0.72.
Table 4 shows the correlation values between studies. In the correlation phase, FRs (30 units) and LS areas (30 units) were used. The table shows that the aspect scores used by Sathyan et al. (2022) (0.466) and Bugday and Akay (2019) (0.464) are directly related to our study. In addition, it can be seen that our study is inversely related to Abay et al.’s (2019) study.
Table 4. Correlation matrix of the studies
Tablica 4. Korelacijska matrica istraživanja
Studies | Acar et al. (2007) | Gumus et al. (2008) | Kayastha et al. (2013) | Abay et al. (2019) | Bugday and Akay (2019) | Buğgday (2019) | Arabameri et al. (2020) | Chiteculo et al. (2022) | Panchal and Shrivastava (2022) | Sathyan et al. (2022) | The study |
---|---|---|---|---|---|---|---|---|---|---|---|
Acar et al. (2007) | 1 | ||||||||||
Gumus et al. (2008) | 1 | 1 | |||||||||
Kayastha et al. (2013) | 0.957 | 0.957 | 1 | ||||||||
Abay et al. (2019) | 0.041 | 0.041 | 0.205 | 1 | |||||||
Bugday and Akay (2019) | 0.017 | 0.017 | -0.13 | -0.989 | 1 | ||||||
Buğday (2019) | 0.849 | 0.849 | 0.722 | -0.247 | 0.264 | 1 | |||||
Arabameri et al. (2020) | 0.296 | 0.296 | 0.264 | 0.274 | -0.302 | 0.316 | 1 | ||||
Chiteculo et al. (2022) | -0.928 | -0.928 | -0.934 | -0.18 | 0.151 | -0.805 | -0.368 | 1 | |||
Panchal and Shrivastava (2022) | 0.602 | 0.602 | 0.705 | 0.54 | -0.542 | 0.396 | 0.18 | -0.818 | 1 | ||
Sathyan et al. (2022) | -0.143 | -0.143 | -0.269 | -0.824 | 0.852 | 0.149 | -0.053 | 0.343 | -0.776 | 1 | |
The study | 0.29 | 0.29 | 0.191 | -0.449 | 0.464 | 0.395 | 0.229 | -0.163 | -0.212 | 0.466 | 1 |
For the study, a module was created in ArcMap 10.8.2 via the Arcpy library and model builder. Thus, ArcGIS users can reproduce their own results just by providing the contour lines, area boundaries and their own sample items. The module can be reached on the Github repository (Github, 2023).
Zaključak
In this study, an objective method was introduced to be used in various analyses in scoring the aspect score. With the proposed method, 30 FRs and 30 LS areas were rescored. The study area was located in the northern hemisphere. Therefore, in this scoring system northern aspects were scored closer to 0, while southern aspects were scored closer to 1. The study was conducted with the assumption that the southwest aspect is scored as maximized, and the northeast aspect is scored as minimized. This use of scoring contributed to the replacement of user-based scoring with objective scoring. The relationship between user-based scoring and objective scoring was examined. Among the 10 studies evaluated, 2 of them, namely by Sathyan et al. (2022) (0.466) and Bugday and Akay (2019) (0.464), were found to have more positive and significant relationships with objective scoring. An ArcGIS module was created for the end user to have easy access to the objective scoring system. The effects of sunny and shaded aspects were especially studied, with the aim of contributing to the new analysis studies with a unique value.
Conflict of interest: The authors declare that they have no known competing financial interests or personal relationships that could have appeared to influence the work reported in this paper.
Appendix. Aspect Scores of Forest Roads and Landslide Area
Table S1. Aspect scores of forest roads according to studies
Tablica S1. Ocjene orijentacije šumskih cesta prema istraživanjima
Table S2. Aspect scores of landslide area according to studies
Tablica S2. Ocjene orijentacije područja klizišta prema istraživanjima