Introduction
Thermal stress has a great impact on milk production in temperate (Lim et al., 2021; Ogundeji et al., 2021) and tropical and subtropical zones (Dikmen et al., 2020; Mbuthia et al., 2021). Climatic conditions in zones of northern Mexico are hot for most of the year. Thus, chronic heat stress adversely impacts milk yield in intensive dairy farming (Rodriguez-Venegas et al., 2022). Seasonal patterns and milk production per cow greatly differ across geographical zones (Guinn et al., 2019). Therefore, a precise milk yield and composition projections by particular zones would benefit dairy farm managers and the market in making policy decisions considering seasonal milk yield and composition. Noticing whether milk yield is more likely to happen on a particular calendar date would improve the understanding of daily milk yield variation in hot environments, its causes, and suitable responses.
High-quality milk is essential for processing high-quality dairy products. Therefore, milk processing plants use bulk tank milk analysis to reward farmers who produce high-quality milk. Hence, composition of cow's milk is economically crucial to both, milk producers and processors. Milk is nutritionally vital to consumers worldwide due to its high-quality proteins, high calcium and phosphorus content, some microelements, and significant amounts of fat-soluble and water-soluble vitamins, especially vitamin B (Pereira, 2014; Antunes et al., 2022).
Many factors affect milk composition, one of them being heat stress. Heat stress has been associated to a decline in total protein and fat content (Bernabucci et al., 2015; Hill and Wall, 2015; Ouellet et al., 2019). Additionally, heat stress changes the triacylglycerol profile and reduces phospholipids, which modify the physical properties of milk fat as well as the nutritional value of milk (Liu et al., 2017).
Most studies on the effect of thermal stress on milk yield and quality have been conducted in zones with short, warm periods. In some areas of northern Mexico, numerous consecutive months of heat-stress conditions exist. These consecutive days of thermal stress significantly impact milk yield and composition, reducing the chance for cows to dissipate body heat at night. Consequently, the body temperature surge is accumulated daily for months, leading to significant declines in milk yield, reproductive performance, and animal health (Polsky and von Keyserlingk, 2017).
Because of the combined effects of the current and past milk yield and milk component levels on future lactation, it is vital to accurately forecast the milk yield and its quality at the herd level. Therefore, this study aimed to predict trends, seasonal effects, and future yields in cow’s milk in a hot environment through time series analysis. Another objective was to evaluate the seasonal microbial properties of raw milk throughout the year.
Material and methods
Dairy herd
All experimental procedures complied with The Guide for Care and Use of Agricultural Animals of the Autonomous Agrarian University Antonio Narro (#3001-2423). A commercial dairy farm in northern Mexico (25 °N, elevation 1140 m, mean annual temperature 24.6 °C, mean annual rainfall 230 mm) was used. During the study period, the maximum ambient temperature ranged from 1 °C to 41.4 °C, and the relative humidity ranged from 13 % to 81 %. The herd ranged from 2256 to 3299 lactating Holstein cows (mean= 2931; median= 2973; standard deviation= 234). Animals were kept outdoors in open-dirt pens with fans operating during the warm weather. Pens were equipped with ample shade structures and feeding alleys. Cows were fed diets formulated to meet or exceed the suggested daily nutrients for 670 kg dairy cows producing 38 kg/day of 3.5 % fat-corrected milk (NRC, 2001). Some cows in this study initiated their lactation with an abortion, and about 18 % of the cows had prolonged lactations (>400 days).
Cows were fed a total mixed diet containing ground-shelled corn and soybean meal in the concentrate mix (50 % of the diet); the forage portion of the diet was corn silage and alfalfa hay. Diets are pretty homogeneous all year round in terms of ingredients and nutrient content. Cows were fed two times per day. Animals had a free access to water at all times.
Milk yield recording
The lactation number of cows included into the study varied from one to eight. Cows were milked thrice daily (04:00, 12:00, and 20:00 hours), and milk yield was recorded electronically at each milking for individual cows. The daily milk yield per cow was estimated as the sum of the harvested milk from midnight to midnight divided by the number of milked cows. The daily milk yield's mean, standard deviations, median, and range were 30.4, 2.5, 30.1, and 25.3-35.1, respectively. Cows completed their tenure when they reached 210 days of pregnancy or produced ≤20 kg of milk/day. The actual 305-day rolling herd average for the se dairy herds was about 10350 kg, and the percentage of culled cows per year varied from 26 % to 33 %. Milk yield and composition were recorded for seven consecutive years (2014- 2020).
Collection and processing of bulk tank milk samples (BTM)
BTM samples were obtained weekly for 365 days to record milk components. A volume of 50 mL of milk was collected at 4 °C no later than eight hours after milking. Milk collection was performed by using sterile plastic screw-cap centrifuge tubes after an agitation cycle. The tubes were placed into an icebox and transferred to the laboratory for analysis within 15 min. The total solids (TS), fat, protein and lactose content in milk were measured by near-infrared spectroscopy using a MilkoScan FT120 (Foss, Hillerød, Denmark). Milk was tested for urea nitrogen using a FOSS 4000 analyzer (Foss North America, Eden Prairie, Minnesota, USA). All analyses of raw milk were carried out in triplicate.
Bacteriological analysis of raw milk
The milk was collected daily and was a mix of three consecutive milkings. Raw milk samples from the bulk tank were placed in sterile 50-mL tubes. The milk samples were mixed thoroughly several times, and 20 mL of this milk was transferred to a snap-cap vial to determine BTM somatic cell count. The remaining part of the milk sample was used for bacteriological analysis and milk components.
Milk samples were examined for the standard plate count (SPC) by a pour plating method using standard growth medium followed by aerobic incubation for 48 h at 32 °C, after which bacterial colonies were enumerated and expressed as colony-forming units per mL (cfu/mL). Coliform count (CC) was assessed by spreading a milk sample onto a MacConkey Agar followed by incubation at 32 °C for 24 h; colonies were expressed as cfu/mL. The thermoduric psychrotrophic count (TC) was assessed by placing milk samples into sterile 20 x 125-mm test tubes and incubated in a water bath at 63 °C for 30 min. Samples were cooled in an ice-water bath for 10 min, placed in duplicate on plate count agar (Remel Inc., Lenexa, KS), and incubated at 7 °C for 10 days. Somatic cell counts (SCC) were performed using a Bentley Somacount 300 (Chaska, MN) according to manufacturer’s procedures.
Statistical analyses
For forecasting daily milk yield and milk components, we set out a back-testing framework for two years of forecasting using a time series approach. STATGRAPHICS software was employed to select a forecasting model that compared multiple models and automatically picked the model that maximized the specified information criterion. A usual practice of this procedure in Six Sigma is to choose an ARIMA model of the ARMA(p,p-1) form, which, unlike most other control diagrams, does not assume independence among sequential measurements.
ARIMA is a mixture of auto-regressive and moving average, represented by p, d, and q. Where p represents the number of lag observations incorporated in the model, d denotes the number of times raw observations undergo differencing, and q is the degree of moving average. The model is as follows:
Yt= ϕ1yt−1+ϕ2yt-2+...+θ1yt−p+ εt
θ1εt – 1 +θ2εt – 2 +…+θ1εt−q
The final automatic model selection provided the optimal model based on the lowest value of the Akaike information criterion. When the model was constructed, the autocorrelation function (ACF) and partial autocorrelation function (PACF) of model residuals were depicted to confirm autoregressive and the moving average parameters and evaluate the goodness of fit. The Box-Jenkins procedure was used to estimate p, d, and q values. 30 days cycles were chosen as the measurement unit for analysis of season effect on milk yield and its components because this interval is commonly used in the dairy industry for monitoring milk production. The cross correlation between the milk yield and the THI over time was calculated utilizing the Wessa software (2017) that computes the cross-correlation function for univariate time series.
Also, analysis of the milk components was conducted using the MIXED procedure of SAS (SAS Institute Inc., Cary, NC), with month included in the model as a random effect to account for the clustering of samples within month. The PDIFF option of SAS using the Bonferroni test (PDIFF ADJUST=BON) was used for multiple comparisons of means.
One-way ANOVA (PROC GLM of SAS) was performed on log10 values (for better homogeneity of the distribution of residuals) for each bacterial and cell somatic count. For this analysis, seasons were classified as winter (December to February), spring (March to May), summer (June to August), and autumn (September to November). The PDIFFoption of SAS was used to compare means.
Results and discussion
Figure 1 shows a seasonal variation in the time series within years. Also, tendencies suggest an additive model as the seasonal fluctuations are roughly constant over time. A well-defined trend for this trait could be recognized over the seven years. Predicted monthly daily milk yield of cows was highest in January (34.5; IC=32.9-36.1) and lowest in July (26.1; CI=23.7-28.5). The results indicate marked differences in average daily milk yield in cows during periods of high ambient temperatures compared to cooler temperatures during the year. Production determinants such as the feeding program, genetic merit, and health management were indistinguishable among cows, so the only apparent difference was the daily temperature and humidity. Climatic conditions were the most likely cause of the ample milk production levels during the year. These results align with previous studies in intensive dairy operations in temperate climates where an annual rhythm of milk yield has been established at the cow level (Vallimont et al., 2010) or country level (Salfer et al., 2019; Ferreira et al., 2020) data.
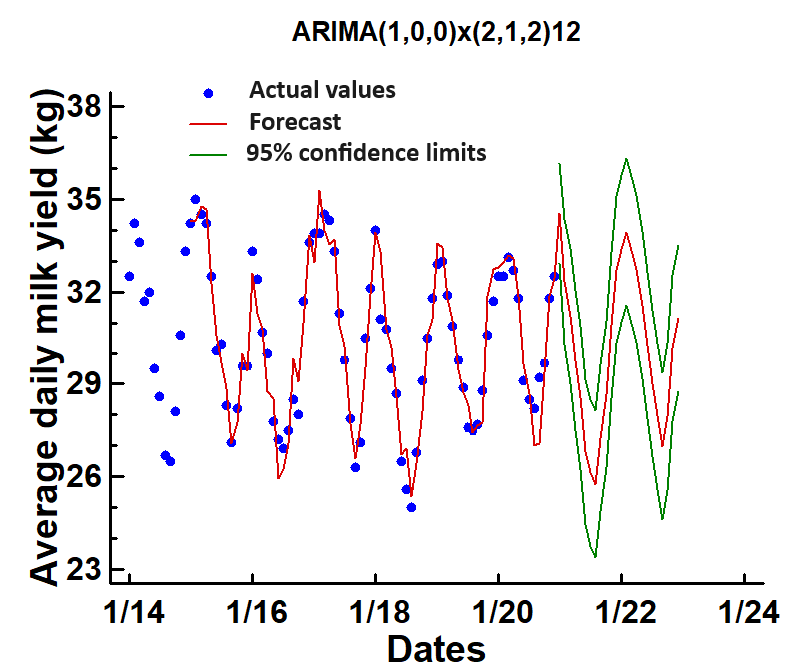
Figure 1. The differences of time series of mean daily milk yield of Holstein cows in a hot environment between 2014 and 2020, based on 12-month intervals. The forecasts are shown in red, and the green lines are 95 % confidence intervals
Figure 2 depicts the cross-correlations between THI (input variable) and daily milk yield (output variable). Correlations were significant and negative for the THI and the daily milk yield in the same month and at lags -1, +1, +2, and +3, indicating that the THI and monthly daily milk yield in cows were negatively correlated 2.5, 5, and 7.5 months earlier, and 2.5 months later, reaffirming the strong negative influence of thermal stress with residual and preceding effects on daily milk yield.
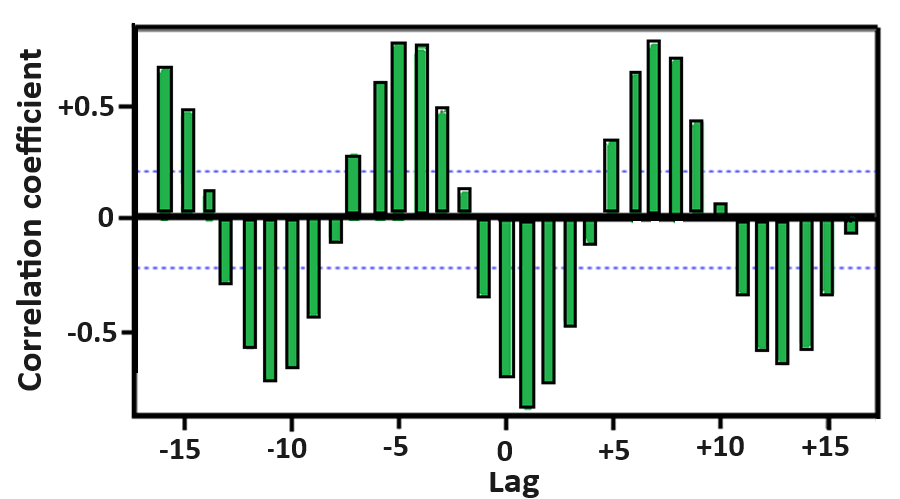
Figure 2. The cross-correlation functions between daily milk yield and monthly temperature-humidity index (THI) average. The spikes denote the cross-correlation coefficients. The dashed lines represent the bounds of cross-correlation functions. Spikes outside the bounds are significant cross-correlation coefficients
These results confirmed that heat stress is the primary driver of milk yield seasonality in intensive dairy operations in a hot-arid environment. Our model found unequivocally, in terms of peak timing, amplitude, or seasonal shape, that thermal stress alone explains milk yield seasonality. The predicted future value based on the past values showed a depression of 8.4 kg in daily milk yield in the hottest part of the year, which bears a significant economic loss in large dairy herds in this hot environment. Our study contributes to improving projections of future milk yield, which is essential for dairy operation planning. This forecasting can determine budget allocation implementation of spatial (e.g., housing, infrastructure, labor) policies.
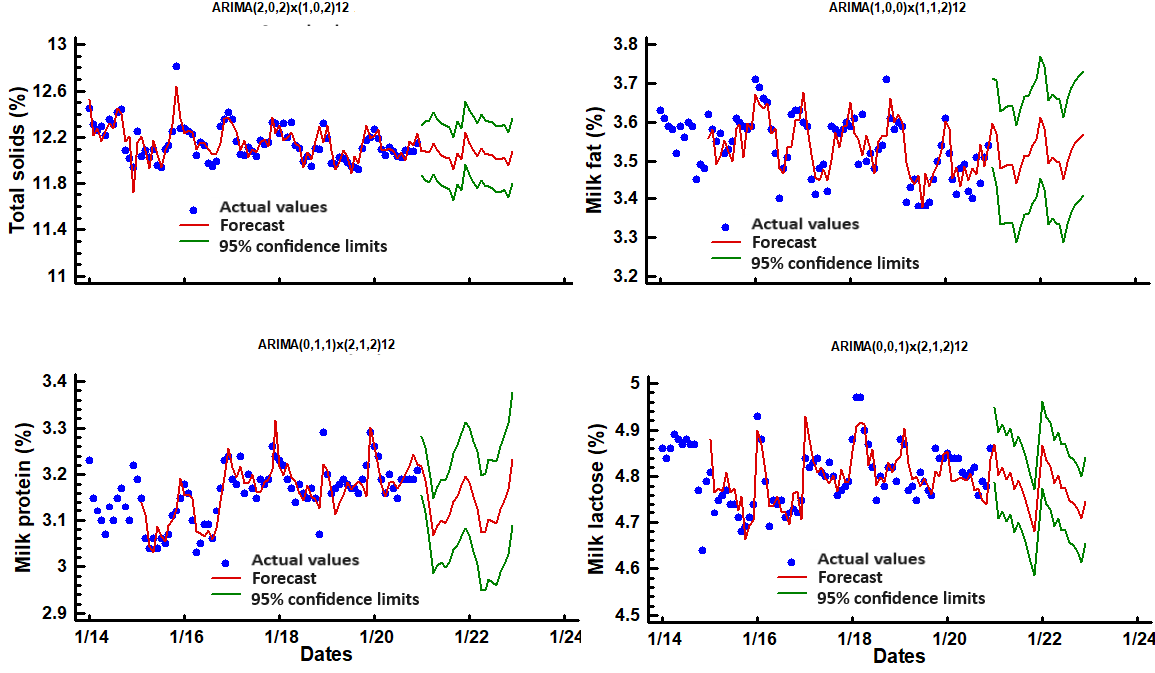
Figure 3. The differences of time series of mean milk total solids, milk fat percentage, milk protein percentage, and milk lactose percentage of Holstein cows in a hot environment between 2016 and 2020, based on 12-month intervals. The forecasts are shown in red, and the green lines are 95 % confidence intervals
Figure 3 shows the monthly mean values of total solids, fat, lactose, and protein content of raw milk of Holstein cows for seven consecutive years. Starting in January, the total solid content of milk steadily declined as the year progressed, with the lowest value in July (12.05 %; p<0.01), and then gradually started to increase to a maximum peak in November (12.31 %). A perusal of Figure 2 indicates an increasing trend in the total solids in the milk in the winter months. P-values for the root mean square error, the mean absolute error, the mean absolute percentage error, the mean error, and the mean percentage error were <0.01, reaffirming the occurrence of a defined trend for milk total solids. The predicted future values based on past values (ARIMA procedure) showed the highest value in January (12.09 %; CI= 11.86-12.31 %) and the lowest in August (12.02; CI= 11.75-12.29).
The values of the total solids in milk were a consequence of the reduction in protein fat and lactose during the warmest months, which is in agreement with the findings of Kadzere et al. (2002), who observed an 18.9 % decrease in solids-not-fat when Holstein cows were transferred from an air temperature of 18 to 30 °C. Chen et al. (2014) and Lohaj and Sulejmani (2020) observed a reduction in total solids content from winter to summer in dairy farms in Europe, which is also in line with the current study. Using the autoregressive integrated moving average (ARIMA), we forecasted the depression of total milk solids during two future years, with annual peaks detected.
Months also affected (p<0.01) the concentration of milk fat, with the highest mean predicted fat content observed in January (3.60 %; CI= 3.48-3.71 %) and the lowest in July (3.44 %; CI= 3.29-3.59 %). The statistical significance of the terms in the forecasting model was <0.01. The drastic decline in raw milk fat in July is generally in line with various studies in temperate zones (Hammami et al., 2015; Garcia et al., 2015). The reduction of milk fat may be partly a result of a decrease in dry matter intake in cows subjected to mild (Gorniak et al., 2014) or severe (Spiers et al., 2004) heat stress, which alters ruminal fermentation reducing acetate and butyrate, substrates required for de novo milk fat synthesis.
Additionally, partitioning of energy in thermoregulation-related mechanisms in response to heat challenge diminishes anabolic activities such as synthesis of fatty acids in mammary tissue, which may also reduce milk fat content during warm weather (Das et al., 2016; Moore et al., 2023). Also, in cows undergoing thermal stress, the circulating non-esterified fatty acid levels are reduced (Abeni et al., 2007), and the adipose tissue is less sensitive to catabolic signals in heat-stressed animals (Baumgard and Rhoads, 2012). In the current study, milk fat showed an increasing consistent trend during the coldest months, as indicated by the time series analysis. The predicting values revealed an annual peak, and these variations, although minimal (0.16 percent points), are likely to be meaningful for commercial dairy herds in this hot environment.
Month had a significant effect (p<0.01) on milk proteins, which varied from 3.12 to 3.20 %. Following previous observations, the forecast values for milk protein percentage were 3.22 % (CI=3.15-3.28 %) in January and 3.07 % (CI=2.98-3.1) in April. All terms in the forecast model (ARIMA) were significant (p<0.0001), which confirms the seasonality of milk protein percentage attributed to ambient temperatures.
Chen et al. (2014) observed that the total protein content was higher in spring than during summer and autumn in European countries. Similarly, Lindmark-Mansson (2003) and Heck et al. (2009) reported that the raw milk protein content was the highest in Sweden and the Netherlands during January and December. Karlsson et al. (2017) observed the highest protein content in November in Swedish raw milk. Similar to the studies mentioned above, in the present study, milk protein content presented minimum values in summer months and maximum values at the end of autumn and during the winter months. Given that cows in the present study were fed the same diets throughout the study period, monthly changes in the main raw milk components were not likely due to dietary variation. Yang et al. (2013) also found that seasonal changes in milk components of Holstein cows under intensive conditions were not due to diet changes, as these researchers found a distinctive milk component depression in summer without changes in the ration offered to cows.
Rhoads et al. (2009) also reported a decreased milk protein content in heat-stressed cows due to a lower synthesis of casein formation enzymes. The detrimental effect of heat stress on the milk protein reduction seems to arise, in part, from the fact that heat stress changes amino acids profile of dairy cows so that more amino acids are required for maintenance (immune response and gluconeogenesis) but not for milk protein synthesis under heat stress (Guo et al., 2018). Also, Guo et al. (2021) suggested that oxidative stress as a consequence of heat stress contributes to a reduction in milk proteins by causing apoptosis in mammary gland tissue and hampering milk protein synthesis by disrupting signalling pathways. The reduction in milk protein and fat content in hot months is particularly harmful in countries that use cow milk for cheese production.
Milk produced in July had the lowest (p<0.01) lactose percentage, with a slight variation in spring, autumn, and winter months compared to July. Even so, the ARIMA method detected that the average value in summer months differed from that in winter months. The extreme forecasting values were 4.87 % CI=4.79-4.95) for January and 4.77 % (4.68-4.86 %) in June. These results are surprising as lactose is well known to be one of the least variable milk components (Heck et al., 2009; Chen et al., 2014). However, Garcia et al. (2015) documented a reduction of milk lactose in Holstein cows under heat stress in a tropical climate. A metabolomics study by Tian et al. (2016) suggests that either less blood glucose is available to the mammary gland or lactose synthesis is disrupted in heat-stressed cows, which would explain the reduced lactose content in summer in the present study. Raw milk's mean urea nitrogen content over the year did not significantly vary with months (12.9±1.8 mg/dL).
Table 1. Seasonal bacterial content of raw milk from Holstein cows in a hot environment (means ± SD)
cfu= colony forming units
a,bWithin columns, means with different superscript differ (p<0.05)
The yearly mean SPC was 6896 cfu/mL for the seven years included in the study. Season affected the SPC, with winter milk having a significantly higher SPC than spring and summer milk (Table 1). The mean TC was higher (p<0.01) in raw milk obtained in autumn and winter than in spring and summer milk. CC was higher (p<0.01) in milk sampled in autumn than in other seasons. Finally, SCC was below the standards outlined in the U.S. Pasteurized Milk Ordinance (Food and Drug Administration, 2015). Thus, the month of sampling did not significantly affect the somatic cell count (mean=252x103±82×103 cells/mL).
Contrary to other studies where TDC in raw cow´s milk reached the highest values during the summer months (Vithanage et al., 2016; Stocco et al., 2023), in the present study, autumn and winter months favored the proliferation of these bacteria in raw milk. The reduced standard deviation for this variable suggests that cleaning practices in the dairy herd in this study did not substantially affect the presence of thermoduric bacteria in raw milk. These results indicate the need for dairy producers to pay distinctive attention to their cleaning-in-place procedures during the autumn and winter months to avoid contamination with thermoduric bacteria, as the primary sources of thermodurics in milk are contamination of the teat skin from bedding and soil (Gleeson et al., 2013).
No seasonal effect was observed on SCC, which is contrary to the findings of other authors in zones characterized by high ambient temperature in summer (Bertocchi et al., 2014; Bernabucci et al., 2015) or milder summer temperatures (Green et al., 2006; Olde Riekerink et al., 2007), where the higher SCC occurs in summer compared to other seasons. However, some other authors have not observed seasonal fluctuations in SCC in hot semi-arid environments (Lima et al., 2019). Bulk milk SCC in a herd is affected by subclinical and clinical mastitis, which depends on many factors, among which season is the most critical one (Zucali et al., 2011). Because the epidemiology of each microorganism causing mastitis is distinct, the effect on bulk milk SCC and its relationship to climatic and environmental factors might differ. Given the strong association between udder hygiene and bacterial counts in BTM (Elmoslemany et al., 2009), these data suggest that cows were dirtier in a particular season.
Conclusions
Long-term trends based on time series evidenced a considerable effect of the seasonality of the average daily milk yield with a predicted depression of 8.4 kg in daily milk yield in the hottest part of the year. Also, results of this study showed a considerable variation throughout the year in concentrations of the main bulk tank milk components in a high-input dairy herd of northern Mexico, with the highest depression of these components in the summer months. The ARIMA procedure was reasonably accurate in forecasting milk yield and components as the year progressed. Autumn and winter were the most critical seasons for standard plate count and thermoduric count in raw milk, with no changes in somatic cell counts in different seasons. Thus, seasonal fluctuations must be considered when processing milk, as they greatly affect the bacterial microflora in raw milk. Additionally, applying time series provided a practical methodology to distinguish seasonal patterns across time. It is essential knowledge for dairy producers to allow them to make ideal decisions from an economic perspective in this zone of intense and prolonged thermal stress.