INTRODUCTION
Zeolites are porous, crystalline aluminosilicates. Natural zeolites, also known as clinoptilolite, have microporous structures with AlO4 and SiO4. The aluminosilicate networks create micropores and cavities in size between 4–12 Å (1) (2). The most important properties of zeolite are high ion exchange capacity, reversible dehydration, and high absorption capacity (3). They have widespread use in agronomy, industrial processes, ecology, cosmetics, and medicine. Since zeolite is generally used to detoxify the body, it is interesting to obtain information about its elemental composition, which content may pose a risk to the quality, efficacy, and safety of the product (4). Zeolite, as a relatively new dietary supplement, shows numerous positive health effects, such as immunostimulatory, antioxidant, antidiarrheal, and antitumor effects (5–7).
The crystal structure of zeolite contains a large number of clearly defined cavities in which monovalent and bivalent cations, water molecules, and other polar molecules can be found (8). Manganese (Mn), iron (Fe), and zinc (Zn), which can be found in zeolite structure, are important as enzyme cofactors and therefore are important for cellular metabolism and antioxidant defense (7, 9, 10). Determination of elemental composition is significant for the quality, safety, and origin of zeolite samples. The most common techniques used for elemental analysis are spectroscopic techniques such as inductively coupled plasma-mass spectrometry (ICP-MS), inductively coupled plasma optical emission spectrometry (ICP-OES), and flame atomic absorption spectrometry (FAAS) (11). The main disadvantage of these techniques is the total destruction of the organic matrix by chemical treatment. Today, the focus is on techniques with less or no sample pretreatment and with the possibility for multi-elemental analysis (12).
This work describes the development of a suspension method for the determination of Mn, Fe, and Zn by total reflection X-ray fluorescence spectrometry (TXRF) (13) using response surface methodology (RSM) (14) in the sample of micronized zeolite clinoptilolite. TXRF has the possibility of direct analysis of solid samples as a suspension, without previous complete decomposition of the sample, and therefore it can be a valuable analytical technique for simple, fast, environmentally friendly, and economic analysis.
TXRF is a type of energy-dispersive X-ray fluorescence spectrometry (EDXRF) that has a high sensitivity and detection limits in the ppb range (15). The main reason for this is that the primary beam hits the sample at a very small angle and the detector is placed very close to the sample, resulting in improved detection capability and lower background noise (16). In order to perform an analysis under total reflection conditions, the samples must be prepared as (ultra)thin films. For this purpose, a small amount of the material (in the range of μL to μg) is applied to the surface of a reflective carrier and then dried before the TXRF analysis is performed. In addition, preparing the samples as a thin film largely eliminates matrix effects such as absorption or secondary excitation (17, 18), so that internal standardization can be used directly for quantification in TXRF analysis (19). Internal standardization is based on the addition of an element called an internal standard, which itself should not be present in the original sample, should not interfere with the target elements, should have a suitable XRF response, and should have a concentration in the middle concentration range of all elements to be determined.
TXRF is mostly used for the analysis of liquid samples (with or without dilution, depending on the complexity of the sample matrix) or solid samples after digestion (20, 21). The TXRF method also allows the use of small amounts (e.g., a few milligrams) of solid samples to perform the analysis with a suspension sample preparation. Recent applications of this method include suspending a few milligrams of the sample in a suitable disperser for multi-element analysis of various solid samples.
Requirements for less sample manipulation and avoiding time-consuming digestions reduce chemical consumption, environmental impact, risks, costs, and time (22). One of the goals of modern chemistry is the application of analytical methods that incorporate the principles of "green chemistry", which is why TXRF has become an important tool for qualitative and quantitative multi-elemental analysis (23, 24). Multi-variate experimental designs allow the simultaneous optimization of several control variables and therefore are more cost-effective and faster to implement in comparison to univariate analysis. Box-Behnken design is a very efficient response surface design providing information on the effect of experiment variables and overall experimental error in a minimum number of required runs (25). Since zeolite clinoptilolite is used for its medicinal benefits, the impact of zeolite on two commonly used dietary supplements with good antioxidant properties, green tea, and ascorbic acid, as well as the antioxidant potential of zeolite itself, was assessed.
EXPERIMENTAL
Chemical reagents and materials
Stock solutions of 1.000 mg L–1 in 2 % nitric acid (Kemika, Croatia) of the investigated elements (Mn, Fe, and Zn, purchased from Sigma-Aldrich, USA) were used to prepare standard solutions in the appropriate concentration range for FAAS analysis (USA). High-purity water used for dilution of stock solutions and samples was obtained from a Milli-Q purification system (Millipore Corp., USA. The zeolite clinoptilolite, purchased from local drug store, was digested in the microwave with nitric acid (≥ 69 %, Sigma-Aldrich), hydrofluoric acid (≥ 48 %, Sigma-Aldrich) and hydrogen peroxide solution (≥ 30 %, TraceSELECT®, Sigma-Aldrich).
The internal Ga standard (Agilent technologies) solution concentration of 200 mg L–1 was prepared from a Ga stock solution of 1.000 mg L–1 (in 5 % nitric acid, TraceCERT®, standard for ICP). In TXRF analysis, the sample carrier plays an important role in achieving optimal analytical results. In this work, considering the higher resistance and lower background, quartz glass reflectors with a diameter of 30 mm and a thickness of 3 ± 0.1 mm were used as sample carriers for introducing the sample into the TXRF device. All quartz glass disc reflectors were coated with a silicone solution in isopropanol (Serva GmbH & Co, Germany). This created a hydrophobic coating that facilitated the deposition of the samples.
The certified reference material, NIST 1573a (tomato leaves) from the National Institute of Standards and Technology (USA) was used to verify the accuracy of the developed TXRF method. All glassware used for standard preparation was carefully cleaned with MilliQ water after soaking overnight in 15 % nitric acid before use.
2,2-Diphenyl-1-picrylhydrazyl (DPPH), rosmarinic acid (97 %), chlorogenic acid (95 %), and gallic acid (98 %) were purchased from Sigma-Aldrich. Ethanol was purchased from Gram-Mol (Croatia). Ascorbic acid was obtained from Acros Organics (Belgium). Green tea was purchased from Suban (Croatia).
Sample preparation for TXRF analysis
Different amounts of the powdered sample (10, 20, and 40 mg) were filled into polypropylene tubes and 1 mL of the dispersant solution was added. In the present study, solid suspensions were tested with Mili-Q water or with a 1 and 5 % solution of a commercially available non-ionic detergent (Triton X-100, Sigma-Aldrich,) and the deposition volume of the sample (2, 5, and 10 μL) on the sample carrier was also investigated. The second preparation method consited of digesting the zeolite sample using the same procedure as for the FAAS analysis in order to use it as a reference value for the entire study. Ga was used as an internal standard (IS) in both sample treatments. Then, 50 µL of a 200 mg L–1 Ga solution was added to each sample to obtain a final concentration of 6 mg kg–1 of Ga for quantification purposes.
The experiment design method (response surface analysis) was applied to select the best strategy for sample preparation method for TXRF analysis. Subsequently, based on the results obtained, sample suspensions were prepared by weighing 10 mg of the sample and adding 1 mL of 5 % Triton X-100 with 10 μL of 200 mg L–1 Ga (final concentration of 6 mg kg–1) as IS. The resulting suspension or solution was thoroughly homogenized (using a vortex device, 30 s), and a 10 μL aliquot was transferred to a quartz glass sample carrier pretreated with silicone in isopropanol and dried on a hot plate (approx. 40 °C)for subsequent TXRF analysis. Duplicates were prepared for each sample.
Sample preparation for FAAS analysis
A microwave acid digestion method was employed for the digestion of zeolite clinoptilolite prior to the FAAS analysis. In a previous publication, the suitability of this method for the complete digestion of organic matrix samples was already demonstrated (13). The microwave oven used was an MLS120 Omega (Milestone, Italy). A microwave acid digestion, based on the EPA method 3052 (26), was employed for the preparation of zeolite clinoptilolite samples. In brief, about 150 mg of sample was added to high-density Teflon vessels with 9 mL of nitric acid (65 %), 1 mL of hydrofluoric acid (48 %), and 1 mL of hydrogen peroxide (33 %). The vessels were closed and heated following a two-stage digestion program consisting of a first step of 5 min to reach 180 °C and a second step of 10 min at 180 °C. After cooling, digested sample solutions were transferred to a 20 mL flask and brought to volume with ultrapure water.
TXRF analysis. – TXRF analysis was performed with a benchtop S2 Picofox TXRF spectrometer (Bruker Nano GmbH, Berlin, Germany) equipped with Mo X-ray source working at 50 kV, and 1000 μA and silicon drift detector (SDD) with a resolution of less than 139.43 eV at Mn-Kα. The acquisition time was
600 s.
Evaluation of the TXRF spectra and the calculation of the net peak areas of the analytes were performed using the commercial software linked to the systems (SpectraSoftware 7.8.2.0). With this software, the spectral background, composed of the detector shelf and scattered excitation radiation, is calculated by a mathematical algorithm, and subtracted from the spectrum. In TXRF analysis, since samples are prepared as thin films on a reflective carrier, matrix effects are usually neglected, and quantification can be performed directly by internal standardization using Equation 1 (20):
(1)
where ci is analyte concentration, Ni analyte net peak area, cis IS concentration, Sis instrumental sensitivity for the IS, Nis IS net peak area, and Si instrumental sensitivity for the analyte.
FAAS analysis. – The Atomic Absorption Spectrophotometer Perkin-Elmer Analyst 800 (Perkin-Elmer Instruments, USA) with deuterium background correction under optimized measurement conditions with suitable hollow cathode lamps and at optimal flame height (air-acetylene) was used for the elemental determination of zeolite clinoptilolite sample. The results were recorded and processed using AAWinlab 32 software (Perkin-Elmer).
An external calibration was performed for the determination of the metals of interest. Good linearity was observed for all elements at the concentration intervals tested (Mn 1–10 µg mL–1; Zn 0.05–2 µg mL–1; Fe 1–20 µg mL–1). The correlation coefficients were in the range of R2 = 0.9951–0.9993. For each sample, three replicates were performed in independent work sessions.
The recovery was calculated for each experiment considering the data obtained from the analysis of the zeolite clinoptilolite sample prepared by the microwave acid digestion method using FAAS. The percentage of recovery was calculated using the Equation 2 and was the selected response:
Recovery ( %) = (2)
where is the metal concentration obtained in the zeolite clinoptilolite sample using the suspension preparation method and measured by TXRF and is the metal concentration obtained in the zeolite clinoptilolite digested by the microwave acid method and measured by FAAS.
The response surface method (RSM) is a multivariate technique that mathematically adjusts the investigated experimental area in the theoretical design by a response function (27). RSM is an empirical modeling technique that evaluates the relationship between a set of controlled experimental factors and observed outcomes. This optimization process essentially involves three steps: conducting the statistically designed experiments, estimating the coefficients in a mathematical model, predicting the response, and testing the adequacy of the model. In this paper, the development by RSM using a Box-Behnken matrix for three variables is described (28). The significant variables such as sample amount, volume deposition, and dispersant were selected as critical variables and labeled A, B, and C respectively. The low, medium, and high values of the individual variables were labeled –1, 0, and +1 respectively, and are listed in Table I. The complete experimental design was performed in random order and 17 combinations were performed with five replicates at a central point to estimate the error (Table II). The experimental data were analyzed using multiple regression equations to create a second-order polynomial model. The model equation used (Equation 3) is shown below:
y = (3)
where y is the estimated response, is the constant term; are the linear coefficients; are the interactive model coefficients between the tree factors (; , and are the quadratic coefficients.
An experimental design method was used to determine the most important parameters for sample preparation. The analytical response was the percentage recovery of the elements tested (Mn, Fe, and Zn), using as reference values the metal concentrations obtained from the analysis of the prepared sample by microwave digestion and FAAS analysis. The regression analysis of the results showed optimal combinations of three independent variables (sample preparation conditions) taking into account the values obtained for the dependent variables, Mn, Fe, and Zn content.
Table I. The levels of variables chosen for the Box-Behnken experimental design
Levels | ||||
---|---|---|---|---|
Independent variable | Code | –1 | 0 | 1 |
Sample amount (mg) | A | 10 | 20 | 40 |
Deposition volume (μL) | B | 2 | 5 | 10 |
Disperser agent | C | Mili-Q water |
1 % Triton X-100 |
5 % Triton X-100 |
Table II. The Box-Behnken design for the optimization method for sample preparation
Run | Sample amount | Deposition volume | Disperser agent |
---|---|---|---|
1 | 1 | 0 | 1 |
2 | –1 | 0 | 1 |
3 | 0 | 1 | –1 |
4 | 0 | 1 | 1 |
5 | 0 | 0 | 0 |
6 | –1 | –1 | 0 |
7 | 0 | 0 | 0 |
8 | –1 | 1 | 0 |
9 | 1 | 1 | 0 |
10 | 1 | 0 | –1 |
11 | 0 | –1 | 1 |
12 | 0 | 0 | 0 |
13 | 0 | 0 | 0 |
14 | 0 | –1 | –1 |
15 | 1 | –1 | 0 |
16 | 0 | 0 | 0 |
17 | –1 | 0 | –1 |
DPPH radical scavenging activity
DPPH radical scavenging activity was assessed according to the method described by Harput et al. (29) with minor modifications. Serial dilutions of phenolic acids (100–0.2 µg mL–1) in 96 % EtOH were mixed with a 10-fold higher concentration of zeolite clinoptilolite and incubated for 1 hour. Green tea (2 g) and ascorbic acid (0.5 g) were each dissolved in 250 mL of water with 2 g of zeolite clinoptilolite and incubated for 1 hour. Freshly prepared 0.36 mmol L–1 DPPH• (70 µL) was added to the 130 µL of tested samples and incubated for 30 minutes in the dark. The absorbance was measured at 492 nm using a microplate reader (Chromate, USA). The radical-scavenging activity was determined by comparing the absorbance of the sample with that of the blank (100 %) containing only DPPH• and solvent. The percentage of DPPH radical scavenging activity was calculated according to the Equation 4:
(4)
where A0 represents the absorbance of the blank and A1 represents the absorbance of the test solution. The IC50 values were calculated by linear regression extrapolation.
The software Design Expert, version 12.0.8.0. (Stat-Ease, USA) was used for experimental design and statistical analysis. The accuracy and suitability of the model were assessed by analysis of variance (ANOVA), with p-values below 0.05 considered statistically significant. Various descriptive statistical parameters were used, such as the p-value, an F-test, degrees of freedom (df), the coefficient of determination (R2), and the goodness-of-fit coefficient (R2adj). In addition, 3D surface plots were used to describe the effects of the interaction between two factors on the desired response.
RESULTS AND DISCUSSION
In this study, a method based on suspension preparation for the determination of elements in samples of zeolite clinoptilolite powder by total reflection X-ray spectrometry was developed. A Box-Behnken design was used to find optimal conditions for the method through a response surface study. Three variables (sample amount, sample deposition volume, and disperser agent) were considered as factors in the optimization study. The zeolite clinoptilolite used in the optimization study was first analyzed by flame atomic absorption spectrometry (FAAS) and TXRF after microwave acid digestion treatment and the content of Mn, Fe, and Zn was determined. The results obtained with TXRF were compared with those obtained with FAAS for recovery studies. The FAAS method was used as a reference, and these values were used as reference values to evaluate the effects of the different parameters used as factors in the optimization study.
In order to verify the suitability of the suspension preparation and the TXRF analysis for the determination of Mn, Fe, and Zn in the samples, the limits of detection (LODs) and limits of quantification (LOQs) for the analysis of the certified reference material NIST 1573a (tomato leaves) were calculated in a first step.
LODs and LOQs for TXRF analysis were estimated from the spectra parameters (background area, Nbkg, and analyte net peak area, Ni) obtained for triplicate analysis of the NIST 1573a (tomato leaves) and using the following expressions (recommended by the TXRF system manufacturer) (Equations 5 and 6):
(5) (6)
where ci is the concentration of a given analyte, Nbkg is the background area and Ni is the analyte net peak area.
The results obtained are shown in Table III. The LODs and LOQs for FAAS are also given for comparison purposes.
LODs and LOQs for FAAS analysis were estimated using the criteria displayed below. As it is shown, intercept and sy/x values of the regression line obtained in the analysis of a set of calibration standards close to origin were used: LOD = yB + 3 sy/x
where yB is the intercept and sy/x is the random error of the response), after the use of respective regression equation (30).
Accordingly:
LOQ = 3.3×LOD
It is interesting to note that the LOQ values for the Mo-TXRF method are similar to those of the FAAS method.
Table III. Limits of detection and limits of quantification for Mo-TXRF and FAAS methods
LOD (FAAS) (mg kg–1) |
LOD (Mo-TXRF) (mg kg–1) |
LOQ (FAAS) (mg kg–1) |
LOQ (Mo-TXRF) (mg kg–1) | |
---|---|---|---|---|
Mn | 0.6 | 0.6 | 2.1 | 2 |
Fe | 1.20 | 0.4 | 3.1 | 1.3 |
Zn | 0.08 | 0.2 | 1.3 | 0.7 |
In the first phase of this study, all samples (zeolite clinoptilolite and certified reference material) were analyzed by FAAS after acid digestion to investigate the performance of the TXRF method in comparison to reference methods. FAAS techniques are generally very commonly used for elemental determination in biological samples (31). The accuracy of the results was evaluated by analyzing the certified reference material NIST 1573a prepared in the same way as the sample by microwave acid digestion (see Experimental), and the content of Mn, Fe, and Zn was determined by the FAAS and TXRF methods. The comparison was made for the elements of interest and the values obtained were compared with the manufacturer's certified values (Table IV) and expressed as recoveries. The percentage recovery of each element ranged between 88 and 95.6 % for the FAAS method and between 104.9 and 111.4 % for the TXRF technique. The results showed that all elements were within the acceptable range (80–120 %) for both methods. The one-way ANOVA test applied to the found and certified values of the certified reference material showed that the results were not statistically different at the significance level of p-values < 0.05. These results confirm the reliability of the TXRF method compared to the reference techniques.
Table IV. Results obtained for the determination of Mn, Fe, and Zn in certified reference material by TXRF and FAAS analysisa
Certified value (mg kg–1) |
Found (FAAS) (mg kg–1) | Recovery (%) |
Found (TXRF) (mg kg–1) | Recovery (%) | |
---|---|---|---|---|---|
Mn | 246 ± 8 | 227 ± 4 | 92 | 258 ± 6 | 105 |
Fe | 368 ± 7 | 351 ± 6 | 96 | 405 ± 12 | 110 |
Zn | 31 ± 1 | 27 ± 0.5 | 88 | 34 ± 1 | 111 |
a n = 6
Regarding the precision, average relative standard deviations were higher for suspension preparation and TXRF analysis (Mo-TXRF: 2–10 %) in comparison with FAAS analysis (FAAS: 1.6–6 %). This fact can be related to the inhomogeneity of the suspension and also the deposition of the solid sample on the reflector as has been pointed out in recent publications dealing with the solid suspension analysis by TXRF (32). Despite the lower precision of Mo-TXRF in comparison with the FAAS method, the Mo-TXRF method presents also some interesting advantages including a simpler sample treatment and quantification approach as well as a reduction of measuring costs and harmful reagents. After the accuracy test, a sample of zeolite clinoptilolite was prepared by microwave acid digestion in the same way as the certified reference material. Mn, Fe and Zn content of the prepared sample was determined by FAAS and TXRF method. As shown in (Table V), in most cases a good agreement was found between the concentrations determined by both methods, but the values determined by the FAAS method, which is prescribed in the Pharmacopoeia for testing the content of elements, were used as reference values for the optimization of the suspension method.
Table V. Results obtained for the determination of Mn, Fe, and Zn in clinoptilolite zeolite material by TXRF and FAAS analysisa
FAAS (mg kg–1) |
TXRF (mg kg–1) | Recovery (%) | |
---|---|---|---|
Mn | 487 ± 28 | 525 ± 53 | 108 |
Fe | 31257 ± 122 | 32751 ± 106 | 105 |
Zn | 124 ± 6 | 156 ± 8 | 126 |
a n = 6
Optimization of experimental conditions for suspension preparation method
The experimental design (Box-Behnken) was used to determine the most important parameters for sample preparation for TXRF measurements of suspensions. Several variables were selected that could potentially influence the effect of the measurement: sample amount, deposition volume, and dispersant.
The analytical response was the percentage recovery of the tested elements (Mn, Fe, and Zn), using as reference values the metal concentrations obtained from the analysis of the prepared sample by microwave digestion and FAAS analysis. The experimental data were processed using the Design Expert program. The regression analysis of the results revealed optimal combinations of three independent variables (sample preparation conditions) considering the values obtained for the content of dependent variables, Mn, Fe, and Zn.
The experimental design matrix and the recovery values as a response achieved under the individual conditions are shown in Table VI.
A recovery of nearly 100 % would represent a better quantitative response of the measurements. Each response variable is the percentage of recovery of each element. Each experiment was performed in triplicate and all calculations were based on the average values; the maximum difference between the three values was less than 3 % of the mean.
Table VI. Box-Behnken design for optimization of sample amount, deposition volume, and disperser agent
a Recovery values
The experimental design matrix and the recovery values obtained by the Box-Behnken design are compared with the experimental data in Table VI. and it was found that the influence of the sample preparation conditions on the concentration of the measured elements in the case of Mn, Fe, and Zn can be represented by a quadratic polynomial equation model (A – sample amount; B – deposition volume; C – dispersant). The relationship between the analytical response (recovery ( %)) and the uncoded forms of the three variables is shown in the following equations. The analytical response is determined by comparing the TXRF and FAAS results for each element.
Mn (%) = 91.32 – 4.4875(A) + 1.1675(B) – 1.385(C) – 3.27(A2) – 4.39 (B2) + 10.37(C2) – 2.535(A)(B) + 5.03(A)(C) + 2.975(B)(C)
Fe (%) = 86.064 – 5.535(A) + 4.5963(B) + 8.7988(C) + 8.4518(A)2 – 3.1107 (B)2 – 2.506(C2) + 7.125(A)(B) + 4.275(A)(C) + 1.2178(B)(C)
Zn (%) = 88.2384 – 0.97(A) + 4.53(B) + 5.8425(C) – 11.3617(A2) + 3.1383 (B2) – 1.5633(C2) – 9.045 (A)(B) + 5.73(A)(C) – 10.055(B)(C)
The model equation for Mn indicates that the recovery is significantly influenced by the sample amount (A) (p < 0.05, F = 29.2), followed by the dispersant (C) and the deposition volume (B) for which the F value is lowest (19.7). The direct influence on the analytical response is indicated by the positive coefficients of B, AC, BC, and the quadratic term (C2). In contrast, the negative terms A, C, and AB, as well as the quadratic terms, A2 and B2, had a reverse impact on the recovery %.
For Fe, the model equation indicates that the disperser agent (C) has a major effect (p < 0.001, F = 15.58) on the recovery. This is followed by the sample amount (A) and the deposition volume (B), which have a relatively low F value of 4.25. The positive coefficients of B, C, AB, AC, and BC, together with the quadratic term (A2), clearly demonstrate a direct influence on the analytical response. In contrast, the negative term A, as well as the quadratic terms, B2 and C2, had an opposite impact on the percentage recovery for that particular element.
Furthermore, the model equation for Zn demonstrates that the disperser agent (C) has a statistically significant impact (p < 0.001, F = 27.1) on the recovery. This effect is similar to that observed for Fe, with the deposition volume (B) and sample amount (A) following closely behind with a relatively low F value of 6.47. The positive coefficients of B, C, and AC, together with the quadratic term (B2), clearly demonstrate a direct impact on the analytical response. On the other hand, the negative terms A, AB, and BC, together with the quadratic terms A2 and C2, impose a reverse effect on the percentage recovery of Zn.
The above-obtained results for the dependent variables are represented in Fig.1.
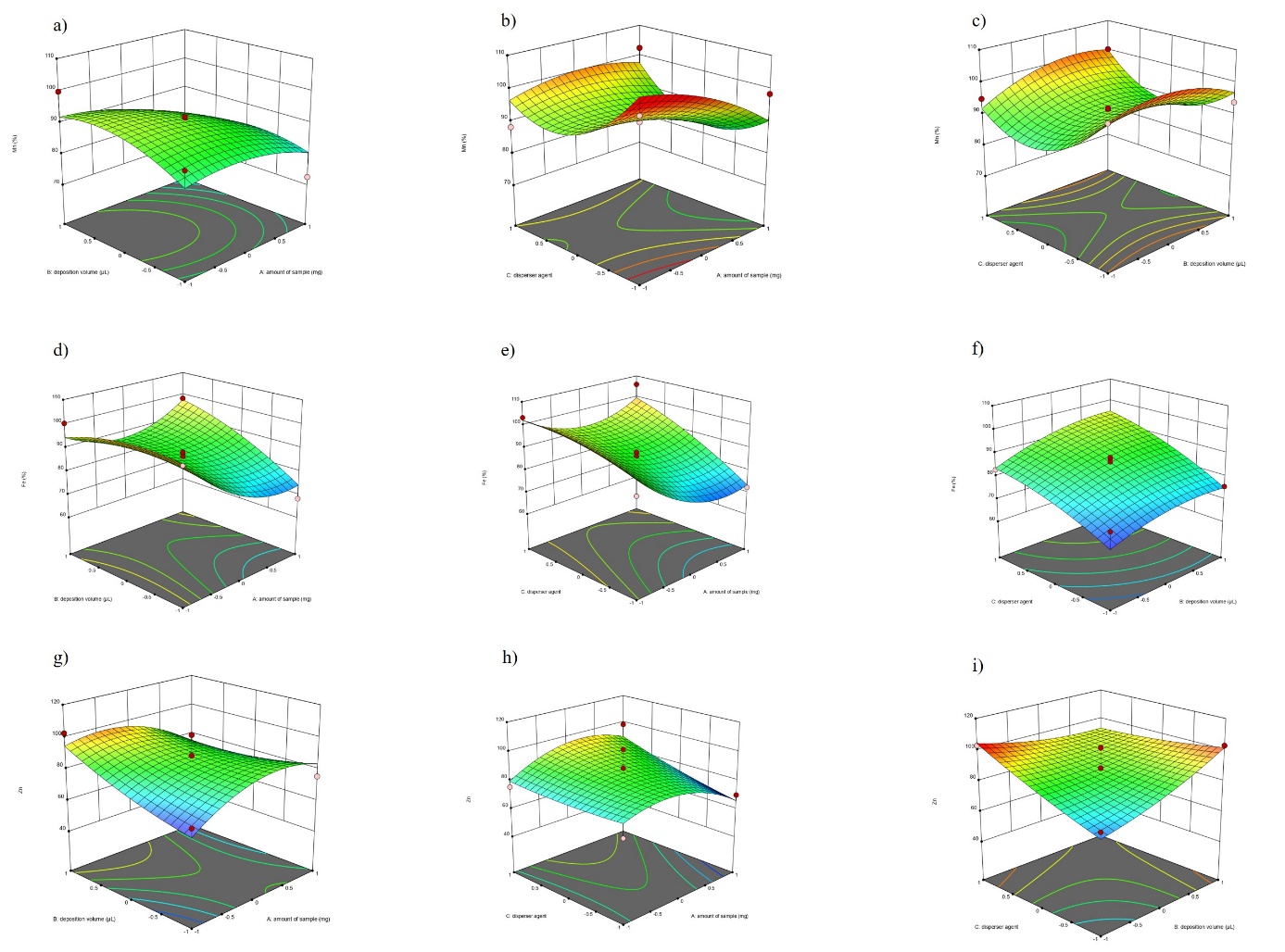
Fig. 1. The response surface plots obtained from Box-Benhken matrix: a) effect of sample deposition volume and sample amount on the recovery of Mn; b) effect of disperser agent and sample amount on the recovery of Mn; c) effect of disperser agent and sample deposition volume on the recovery of Mn; d) effect of sample deposition volume and sample amount on the recovery of Fe; e) effect of disperser agent and sample amount on the recovery of Fe; f) effect of disperser agent and sample deposition volume on the recovery of Fe; g) effect of sample deposition volume and sample amount on the recovery of Zn; h) effect of disperser agent and sample amount on the recovery of Zn; i) effect of disperser agent and sample deposition volume on the recovery of Zn.
The significance of each variable examined was determined using analysis of variance (ANOVA). After fitting the experimental data to the corresponding polynomial models, the validity of the model was confirmed by analysis of variance. Moreover, the ANOVA findings (Table VII) indicated that this regression model performed quite well for all examined elements (Mn, Fe, and Zn) with p < 0.0001 and F = 182.2, p < 0.0011 and F = 146.6, and p < 0.0002 and F = 200.8, respectively. The selected quadratic models were found to be statistically significant (p-value < 0.05). In addition, the regression analysis data showed that the quadratic term, B2, is not significant (p > 0.05) in the case of Mn and Fe.
Table VII. ANOVA analysis for response Mn, Fe, and Zn
The coefficients of determination for all representative data included in the analysis were R2 > 0.9979 and the adjusted R2adj > 0.9955. These values are nearly equivalent to one, suggesting a high level of efficacy of the model.
The larger the amount of suspended sample is, the thicker the deposition layer is, which leads to a reduction of the total reflection effect due to the attenuation of matrix absorption and thus to a higher matrix absorption of the fluorescence signal (33). In this work, among all the factors studied, the sample amount and the disperser agent were the variables that had the strongest effect on the recovery of the elements. The influence of the sample deposition volume (B) on the quantified values was very small. An important factor influencing the quantification of the data was the combination of the two variables A and C (AC). This underlines the importance of the concentration of the sample particles suspended in the dispersant, which influences the thickness and distribution of the deposition layer on the reflector.
Since the best results were obtained by suspending 10 mg of zeolite clinoptilolite in 1 mL of 5 % Triton X-100 solution and using 10 µL of sample deposition volume, this method was identified as the optimized method.
Evaluation of the method's accuracy
The accuracy of the optimized sample preparation procedure was confirmed by the determination of Mn, Fe, and Zn in certified reference materials, NIST 1573a. The results are shown in Table VIII. The statistical comparison using the t-test showed no significant difference between the certified reference material and the experimental results.
Table VIII. Determination of Mn, Fe, and Zn in certified reference materiala
Certified values | TXRF | Recovery ( %) | |
---|---|---|---|
Mn | 246 ± 8 | 249.7 ± 3.5 | 101.4 |
Fe | 368 ± 7 | 369.6 ± 10.2 | 100.6 |
Zn | 30.9 ± 0.7 | 31 ± 1.7 | 100.2 |
a n = 6
The impact of zeolite clinoptilolite on the antiradical activity of phenolic acids was accessed by the DPPH radical scavenging activity assay. The tested concentrations of phenolic acids were mixed with a 10-fold higher concentration of zeolite to investigate the impact of zeolite clinoptilolite on the antiradical activity of selected phenolic acids. Fig. 2b. shows the comparison of IC50 values of pure phenolic acids and the mixture of zeolite clinoptilolite and phenolic acids after 1 hour of incubation.
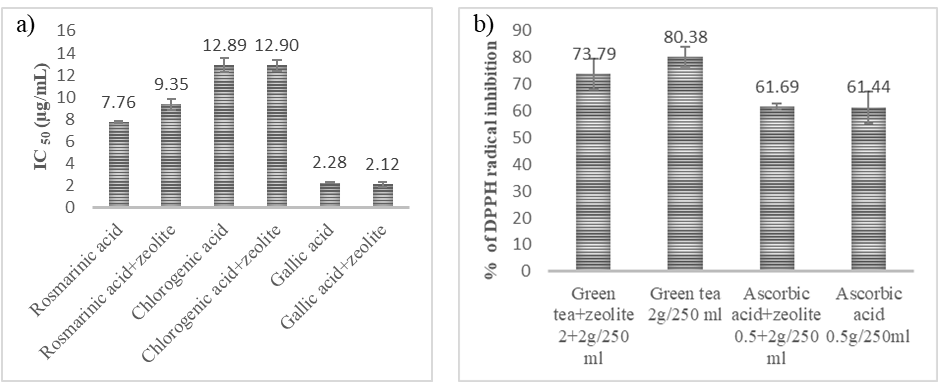
Fig. 2. Impact of zeolite clinoptilolite on IC50 values of: a) phenolic acids and b) on antiradical properties of green tea and ascorbic acid.
A slight increase in IC50 value for the mixture of rosmarinic acid and zeolite clinoptilolite in comparison to pure rosmarinic acid was observed. Zeolite clinoptilolite had no impact on IC50 values of chlorogenic and gallic acid, and zeolite clinoptilolite itself did not show an antiradical effect (data not shown). The influence of zeolite clinoptilolite on the antiradical potential of frequently used antioxidants, green tea, and ascorbic acid was also investigated (Fig. 2b).
The tested concentrations were chosen as the average amount of green tea used for beverage preparation (2 g) while ascorbic acid was tested in the amount which is often found in ascorbic acid supplements (0.5 g). The tested dose of zeolite was chosen according to the recommended individual dose (1 teaspoon which is approximately 2 g). The mixtures were dissolved in 250 mL of distilled water. A slight decrease in antiradical activity was recorded for the combination of green tea and zeolite clinoptilolite, whereas there was no difference between the scavenging activity of ascorbic acid and the mixture with zeolite clinoptilolite. The zeolite clinoptilolite daily intake reaches up to 30 g according to some manufacturer's instructions. It is recommended to use it with tea or juice. Since zeolite clinoptilolite can bind molecules, within this investigation we wanted to reveal the interactions of zeolite clinoptilolite with common dietary phenolic acids, and natural antioxidants. The previous findings of interactions of zeolite with polyphenols are scarce. Thiel et al. (33) concluded that beta-zeolites were promising adsorbents for isolation of polyphenols. Two tested zeolites (a beta-zeolite A with a SiO2/Al2O3 ratio of 35 and a beta-zeolite B with a SiO2/Al2O3 ratio of 150) had high adsorption percentage for ferulic, p-coumaric and caffeic acid (99.1–89.7), while adsorption percentage for sinapic acid was only 48.5. These results were obtained for 10 mL of solution containing 0.1 g L–1 of phenolic acids and 50 mg of zeolite. Our findings do not support this pattern for rosmarinic, chlorogenic, and caffeic acid, since we observed no significant decrease of IC50 values. However, we used a 10-fold higher amount of zeolite clinoptilolite with regard to phenolic acids, while Thiel et al. (34) used a 50-fold higher amount of zeolite. Zeolites have high adsorption capacities and polyphenol affinities, and therefore, when taken together, can cause reduced availability of phenolic acids. Some zeolite-promoting websites claim zeolite has the ability to trap and inactivate free radicals (35, 36), but within our research zeolite clinoptilolite had no impact on DPPH radicals. In previous research, among other pleiotropic effects, zeolite was confirmed as a good antioxidant agent. It activates antioxidant enzymes, such as peroxidase, catalase, and superoxide dismutase, reduces lipid peroxidation, reduces hyperlipidemia, maintains gut electrolyte equilibrium, and reduces oxidative stress (5, 37–39). However, it has no direct radical scavenging activities in in vitro conditions. The exact mechanism of zeolite antioxidant activity is not yet fully understood but may be attributed to the ion exchange process, which releases physiologically important cations such as Mn, Fe, Zn, Ca, and Mg, and makes them available to the organism and antioxidant mechanism (5). Our results revealed that zeolite clinoptilolite did not quench DPPH radicals, nor had an impact on frequently used dietary antioxidants.
CONCLUSIONS
In the present study, preparation of a suspension and analysis by TXRF measurements was developed for the determination of trace elements (Mn, Fe, and Zn) in zeolite clinoptilolite samples by RSM using a Box-Behnken matrix. The main variables such as sample amount, volume deposition, and dispersant were selected and evaluated as critical variables. The method was optimized and validated with certified reference material (NIST 1573a) and then compared with a widely used and accepted method such as FAAS.
The best sample preparation procedure consisted of suspending 10 mg of zeolite clinoptilolite in 1 mL of 5 % Triton X-100 solution, applying 10 μL of the suspension to a siliconized quartz reflector, and drying at 40 °C on a hot plate. TXRF analysis was performed using a standard benchtop instrument with a 600-second run time. The results of the TXRF method were comparable to those of the FAAS method, indicating that this technique can be used instead of the conventional methods. It is easy to handle, and the risk of contamination and loss of analytes is lower compared to conventional methods.
The TXRF method has several advantages over other spectroscopic methods, including the small amount of sample required and the low need for reagents and consumables, as no gas or cooling media are needed for operation. The method developed was simple, fast, cost-effective, and environmentally friendly. Since only a small amount of sample is required, the method can be used especially in research areas where only a small amount of sample is available. Considering the accuracy achieved in the present study as well as the simplicity of the procedure and the low cost, sample suspension followed by TXRF analysis can be recommended as the standard procedure for multi-elemental analysis of different types of zeolite samples.
Although the anti-radical properties of zeolite are often highlighted, in our research, we did not confirm its ability to quench free radicals. Zeolite clinoptilolite also had no impact on the antioxidant activity of dietary antioxidants.
Acknowledgments. – This work was supported by the project FarmInova (KK.01.1.1.02.0021) funded by the European Regional Development Fund.
Conflicts of interest. – The authors declare no conflict of interest.
Authors contributions. – Conceptualization, J.J. and M.B.Š.; methodology, J.J. and M.B.Š.; analysis J.J., M.B.Š., D.P. and E.K.; writing, original draft preparation, J.J. and M.B.Š.; writing, review and editing, J.J., M.B.Š., D.P. and E.K. All authors have read and agreed to the published version of the manuscript.
J. Hao, I. Stavljenić Milašin, Z. Batu Eken, M. Mravak-Stipetic, K. Pavelić and F. Ozer, Effects of zeolite as a drug delivery system on cancer therapy: a systematic review, Molecules 26 (2021) Article ID 6196 (33 pages);https://doi.org/10.3390/molecules26206196