INTRODUCTION
Getting ahead in academia can be challenging, especially for young researchers. As academia is becoming increasingly competitive, both internationally and within the country and even within universities and faculties1,2, researchers often worry about the future of their careers3. An example of such competition is given by Musselin4 who explains how academic competition has evolved in the last decades and now includes not only competition for students, budgets, and professors but competition for quality as measured by algorithms which allow “comparison and rankings between individual researchers, research units, higher education and research institution and countries”4; p.677. Such an approach is seen as gradually transforming universities which are introducing management practices, starting to behave strategically and foregoing collegial relationships in favour of hierarchical ones based on productivity, success, reputation, and the ability to attract students and faculty not only from a regional and national pool but from a global pool of potential students and employees, be it by pulling them in or by branching out, opening research and educational centres in foreign countries4. Given that researchers’ academic promotion and funding are tightly linked to their previous achievement, which is often measured in terms of the number and quality of their publications5, which are, in turn, affected by the scientists’ reputation and funding, such concern about their future does not come as a surprise. When the Matthew effect or “the common cumulative advantage property”1; p.18, and the “success breeds further academic success” notion5; p.7 are considered, the unenviable position of younger scientists becomes even more prominent. Explaining the Matthew effect, Merton makes use of examples found in interviews with Nobel laureates, concluding how “they repeatedly observe that eminent scientists get disproportionately great credit for their contributions to science while relatively unknown scientists tend to get disproportionately little credit for comparable contributions”6; p.2. Such an effect is, according to Merton, especially visible both in cases collaboration with renowned scientists as well as in cases of “multiple discoveries made by scientists of distinctly different rank”6; p.2. So, as do rich get richer, scientists who have already experienced success with publications and are already further along in their careers, also have an easier time with their further career developments, funding, and collaboration1; p.18. Hence, as Petersen et al.1 put it, even a rather small success and achievement in the early stages of a career can have an important role in generating future success and career longevity. In order to achieve that success early, young scientists and PhD candidates seek to connect and collaborate with successful older colleagues and, as Billah and Gauch7state, some even choose their advisors based on their h-index score, as they, according to the latest research, should. This competitive academic environment affects faculties and other research institutions as well, so attracting and retaining successful scientists who generate lucrative projects, innovation and patents in that setting become paramount. Therefore, even when hiring new young researchers, faculties and institutes hiring committees try to assess candidates according to their success potential7,8. Predicting the scientific trajectories of all, and particularly young, researchers is therefore their daily task which takes into account scientists’ publication success and interdisciplinarity rate, length and quality of their training, amount of the funding they received, and again, as will be discussed in detail later, the standing and reputation of scientists’ PhD advisers3; p.201.
PREVIOUS RESEARCH
SCIENTIFIC EXCELLENCE AND INCREASING RESEARCH COLLABORATION
Research collaboration in terms of a growing tendency to publish in co-authorships has been on the rise for quite a long time. Authors like Henriksen9, Kuld and O’Hagan10, and Nabout et al.11 all show the steady rise of co-authorships across various scientific disciplines by analysing data about scientific publications in various time sections between 1966 and 2014. That way, Henriksen9, by analysing over 4,5 million articles cited in the Web of Science’s Social Science Citation Index over a period of 34 years, shows how both the number of authors per article, as well as the share of articles in domestic and international co-authorships have increased in the observed period. Kuld and O’Hagan10 came to a similar conclusion when examining articles in scientific journals in the field of economics published between 1996-2014, while Nabout et al.11show this trend is present even in scientific fields in which, unlike social sciences, co-authorships have always been considered the norm, analysing articles in the fields of botany, zoology, ecology, and genetics published between 1966 and 2015 and showing a drastic reductions in the number of single-author articles in each of the mentioned disciplines. Although at least some part of the increase in co-authorship practices can be attributed to a ubiquitous use of information and communication technologies12 from the 1990s onwards, which enabled authors from different universities, cities and states to collaborate seamlessly, the continuous rise of co-authorships cannot be seen as a sole product of technology, especially as its use has been commonplace for decades. As Brajdić Vuković et al.13 put it, examining collaboration practices among Croatian scientists, increases in the frequency of publishing and co-authorship practices can be traced to changes in academia, which, implementing market-oriented techniques became ever more focussed on efficiency and competition, leading to “increasing time pressures, the fragmentation of time into projects and work-packages and metric-oriented evaluation”13; p.2. Along the market-oriented focus on productivity, an increase in collaboration could also be explained by the changes in the number of published articles needed to get promoted or tenured. While Croatia’s policies about promotions in different science fields14-16show that the number of articles needed has been on the rise since 2005, Henriksen9 argues that such an increase in conditions incentivizes scientists to “game” the system by co-producing publications, be it by giving co-authorship to scientist who contributed little to the article or even those who did not contribute at all, expecting a future quid-pro-quo. Clearly, an increase in number of articles needed in order to get promoted in academia does not necessarily push all the scientists into the murky waters of fake co-authorships, but it could lead them to re-evaluate the advantages of collaboration through the perspective of social capital. Explaining a rise in co-authorships via a social capital and social network perspective would find authors engaging in repeated collaborations with a network of trusted peers, coordinated and maintained by mechanisms such as trust and reciprocal obligations. According to Hu and Racherla17, these networks do not only represent connections among their members, but also “the availability and exchange of knowledge resources within the network”17; p.303. Being part of such a “knowledge domain”, as Hu and Racherla17 define it, means having access to formal (academies and associations) and informal (work groups and task forces) networks that “provide organizational members with a commonly shared platform to collaborate and advance collecting knowledge”17; p.302 resulting in a growing number of co-authored articles in leading journals. Apart from being more productive when publishing in co-authorships, what proves to be relevant in the increasing scientific cooperation is a better visibility of the publications themselves, the potential increase in their quality, but also the impact that greater visibility and higher quality articles have on the career development of the researchers. As Abbasi et al.18 show, co-authored articles and researchers with a higher level of interdependence (i.e. betweenness centrality1), also have higher h-index scores, while Beaver19 shows that co authored articles, compared to single-author ones, will be more cited in the long run, even when published in journals with the same impact factor. Taking these findings into account, it seems that researchers who publish in co-authorships would not only benefit in terms of higher productivity but also in terms of higher impact (measured by h-index scores) which would consequently positively reflect on their career advancement. Although being part of a productive intellectual network, social capital and embeddedness in a productive social network is not just granted to anyone. As Costa20 argues, obtaining social capital entails an “investment cycle” in which scientists gain social capital by successfully conducting research, which requires resources, which in turn require research appointments and grants, which themselves require a history of publications, which close the cycle with scientists needing to conduct research in order to publish. Explaining how young scientists can enter this investment cycle, Costa20 turns to the work of Latour and Woolgar who see social capital as something that can be “borrowed” by advisors and mentors. In that way a protégé can gain access to their mentor’s social network by borrowing social capital, successfully completing their research project and getting awarded with their own social capital which they can then (re)invest in future research and collaboration opportunities. Measured in terms of centrality, a young scientist would “borrow” his or her mentors (weighted) degree2 and betweenness centrality scores, gaining access to connections, and potentially their funding, equipment, and knowledge he or she would not have access to otherwise.
STUDENT-MENTOR COLLABORATION AS A SUCCESS PREDICTOR
The impact that collaboration and co-authorship have on a scientists’ success is especially useful in understanding both early career and later success of the young researchers. Since numerous articles have shown that the collaboration of a PhD student with his or her impactful mentor leads to a better publication activity of young researchers and their greater career development3,8,21-23, the term “standing on the shoulders of giants” is gaining a whole new meaning. One of the greatest predictors of student’s later academic productivity is in fact the early publishing of his or her dissertation’s results. The supervisor’s encouragement is, in general, an important aspect of succeeding in that endeavour2,8, gaining access “to the highly productive section of the academic population”2; p.14, especially if the supervisor himself is part of that cluster. As Ma et al.23; p.14077 put it, “mentorship is arguably a scientist’s most significant collaborative relationship”. Given that early collaboration of young scientists with renowned experts in their field leads either to their repeated collaborations or opens the door to collaboration with other esteemed experts8 Li and thus puts young researchers on the path to their own academic “fame”, that notion seems to hold water. In this context it is particularly interesting to see multiple genealogical studies documenting bonds among the Nobel prize winners, meaning that older laurates trained the younger ones21-23. Apart from this inheritance of excellence, another other way mentors make their protégés’ careers easier lays in the so called “chaperone effect” in scientific publishing which refers to an increased likelihood of a researcher publishing in high-impact, especially multidisciplinary, journal if he or she have already published in the same journal as a young researcher8,22. In that way, not only do young researchers learn how to make their research publishable, but they also learn how to publish in prestigious journals and, more importantly, gain reputation and are given the opportunity publish repeatedly within specific journal22. Finally, it should be emphasized that not all scientific disciplines have the same academic trajectory patterns nor the same doctoral programmes, so it does not come as a surprise that the research to date has also shown some differences in their student-mentor collaborations. PhD students in social sciences generally receive less mentoring and experience lower levels of collaborative productivity than their counterparts in natural sciences. In that way, social sciences and their doctoral programs are still more focused on the individual excellence, where one needs to learn to successfully publish on his own, then they incorporate the systematic student-mentor co-authoring policy, currently more present in natural sciences2; p.14. Taking into account the potential influence mentors have on their protégés’ careers, research questions and hypotheses will focus on exploring whether mentors’ productivity has any impact on their protégés’ productivity in the Croatian context, as to the authors’ knowledge, no such research has been done on this specific research question.
METHODOLOGY
RESEARCH QUESTIONS AND HYPOTHESES
The main goal of this research is to check whether doctoral students who have gained their PhD under the mentorship of successful scientists have significantly higher level of scientific production after finishing their doctoral studies than students who have worked under the mentorship of less successful scientists. The following specific hypotheses arise from this goal: H1: doctoral students whose mentors are more productive will also be more productive upon completion of their studies,
H1a: doctoral students whose mentors are more productive will also be more productive five years after completion of their studies,
H2: doctoral students who co-authored with their mentors after the completion of their studies will be more productive than those who did not,
H2a: doctoral students who co-authored with “star” mentors after the completion of their studies will be more productive than other students,
H3: doctoral students whose mentors have higher betweenness centrality values will have higher betweenness centrality values upon completion of their studies,
H3a: doctoral students whose mentors have higher weighted degree centrality values will have higher weighted degree centrality upon completion of their studies.
As it can be seen, the moderating variable whose influence is being tested in this research is the productivity and networking of the doctoral students’ mentors, or to be exact their “star player” status and the lack of it.
SAMPLE AND DATA COLLECTION
This study focuses on young researchers with active profiles on the publication repository aggregator Croatian Scientific Bibliography (CROSBI) who gained their PhD in the period of 2010 and 2015 and whose PhD information are available on the CROSBI site. The data used in the analysis includes the scientific field of the dissertations, the year researchers obtained their doctoral degrees, the number and classification of articles they published before and after that year and the number and kind of their collaborations both with their mentors as with other researchers. Gathering information about young PhD holders is not enough, the other focus of this research is getting insight into their mentors’ scientific excellence, by gathering information on the number and classification of articles they published, and their collaborations with more and less successful researchers, all in order to determine their “star player” statuses. Additionally, in the few cases where PhD students had more than one mentor, only the most productive and networked mentor (in terms of weighted degree centrality) was retained, as a student would have access to connections of both his/her mentors, and, in order to test whether greater mentor productivity influenced his or her student’s productivity, a more productive mentor seemed as an appropriate choice. To investigate co-authorship networks of the early career researchers and their mentors, but also to make assessment on their excellence, this study uses the social network analysis approach. Social networks, as described by Marin and Wellman24, represent the set of relevant nodes connected with one or more edges, where nodes can be any object of measurement that can be connected to another, similar object of measurement. As was the case with most of the other research that applied social networks to a co-authorship study in the research communities, where nodes represented researchers and edges co-authorships between pairs of nodes7; p.27, this study will not be an exception.
DATA PREPARATION AND ANALYSIS METHODOLOGY
In order to efficiently test the hypotheses, certain values will need to be computed: productivity, first appearance, average productivity, a productivity scale, betweenness centrality, weighted degree centrality, mentor-protégés collaboration, and “star” status. Their logic, construction and applications will be briefly explained in the following text. Productivity will be calculated for every mentor and protégé, taking into account all original scientific articles, preliminary communications, review articles, and professional articles published by all respondents in the time period between 1997 and 2020. This will result in an author-specific absolute productivity value which will be further divided in order to test first and second hypotheses. Absolute productivity will be divided into three different variables (productivity –5, productivity 0 and productivity +5 years) based on the year in which every protégé published his/her doctoral thesis3. Specifically, if protégé A published his doctoral thesis in 2012, his productivity –5 would consider all articles published from 1997 to 2007, productivity 0 would take into account all articles published from 1997 to 2012, and his productivity +5 will take into account all articles published from 1997 to 2017. These different sets of productivity will allow us to test the impact mentors had on protégés’ careers. First appearance is a value which will be assigned to every mentor based on the year they defended their doctoral dissertation, functioning as an approximation of their career length. As this article focuses on respondents who attained a PhD in the period between 2010 and 2015, first appearance will be calculated for respondents who mentored doctoral dissertations in that time period. For mentors that did not upload their PhD dissertation to CROSBI their first scientific4 article will be taken as the year of first appearance. Mentors who did not upload their doctoral dissertations to the CROSBI database, did not publish relevant scientific articles or published only after the cut-off period of 2010 (Ntot = 69) will not be taken into consideration for data analysis and will be considered missing on the productivity variable. First appearance should be an important control value, considering that absolute productivity could offer misleading information, favouring older mentors who have been scientifically active for more time, hence having had the possibility of publishing more articles than the “newer” mentors. After calculating the first-appearance value for all mentors, their absolute productivity will be averaged with their period of activity, neutralizing for the most part, the advantage of older mentors compared to the newer ones. Specifically, if Mentor A has been active since 1997, publishing 50 articles in that period, his 1997-2010 average productivity will have a value of 3,9 (50 articles divided by 13 years of activity) while Mentor B, who has been active since 2005 and has published 25 articles will have an average productivity value of 5. Although not a perfect way to neutralize data imperfection, as it is possible that have not been active throughout their whole career (starting from the first-appearance point) or that they published a great deal of scientific articles before attaining their PhD, an average productivity should be a much more clear-cut indicator of mentor productivity that an absolute value. Finally, in order to test the first hypothesis, mentors’ average productivity will be divided in quintiles in order to produce the variable productivity scale, grouping mentors in five categories: 1 – extremely low productivity, 2 – low productivity, 3 – average productivity, 4 – high productivity, 5 – extremely high productivity. This scale will be based on values of average productivity, as, using absolute productivity values, Mentor A would probably be assigned a higher value than Mentor B. Centrality measures will be computed for all respondents by analysing their connections in the CROSBI database using Gephi, a network analysis software. To test respondents’ positions and centrality values in the scientific network of Croatia, all published articles and collaborations have been gathered from CROSBI, forming a network that includes 30 141 authors, 526 353 publications, and 1348 420 collaborations between authors in a time period between 1960 and 2020. As network values are very susceptible to missing data, since one missing connection between two authors could greatly affect their network reach and, subsequently, their centrality values, it was necessary for this network to include more types of publications5 than those examined for productivity. After gathering the publications and computing the network, betweenness centrality and weighted degree centrality scores will be computed for each respondent, with betweenness centrality functioning as a measure of how often a specific respondent finds himself as a link on the shortest path between any two other authors in the network, and weighted degree centrality as a measure of “reach”, showing with how many different authors has a specific respondent co-authored publications accounting for repeated collaborations, indicating stronger connections with authors who tend to repeatedly collaborate in research and publication. Betweenness and weighted degree centrality are both important in setting a protégé on a path of academic excellence, as mentors with higher betweenness centrality scores will be more often in the position of sending and receiving important information to their connections in the network6. On the other hand, mentors with higher weighted degree centrality will have access to a larger and denser pool of scientists and potential co-authors, which, according to Krackhardt25, often indicates a higher degree of trust and, consequently, a higher degree of willingness to help. As in the case of productivity, centrality measures will be computed as three different variables (–5, 0, and +5 years) in order to test whether mentors’ centralities values are correlated with the ones of their protégés’. To test whether collaboration with their mentors will have any effect on either productivity or centrality measures, a bimodal variable has been computed based on whether mentors and protégés have co-authored publications five years before or after the protégés have published their doctoral dissertation. These variables will help in further differentiating mentors’ impacts on their protégés careers. Finally, in order to better discern whether collaborating with highly productive mentors (stars) leads protégés to start publishing more, a final variable will be computed taking the top 20 % most productive mentors (by average mentor productivity) in each of the examined science fields. Crossing those values with collaboration after PhD attainment will result in a variable with four categories: star mentor that collaborated with respective protégé, star mentor that did not collaborate with protégé, not star mentor that collaborated with protégé, and not star mentor that did not collaborate with protégé. Using ANOVA to compare protégés’ productivity based on these four groups should give an answer to whether collaboration and mentors’ “star” status has an effect on protégés’ productivity. Testing the hypotheses will be achieved applying simple linear regression to the gathered data in order to investigate the correlation between mentors’ and protégés’ values, while ANOVA and t-tests will be applied to productivity scale and mentor-protégés collaborations in order to inspect whether more productive mentors are more often mentoring more productive protégés and whether protégés that collaborated with their mentors turn to be more productive or connected than those who did not.
RESULTS
In order to explore the effects of co-authorship networks, this study focused on mentors and PhD candidates active in the period between 2010-2015, which amounts to 2 233 of unique mentors and 4 106 of unique PhD candidates, shown in Table 1, grouped by scientific fields. The number of doctoral dissertations does not differ greatly between scientific fields, with the exception of biotechnical sciences, which, compared to the rests is a relatively young field. Average mentor productivity, on the other hand, shows that scientists in the fields of biomedicine, natural sciences, and biotechnical sciences are much more productive than those in the fields of social sciences, humanities, and, somewhat surprisingly, technical sciences7. Inspecting for collaborations between mentors and protégés shows that collaborating both before and after a protégés doctoral dissertation is much more common in the STEM8 fields than it is in the social and humanities with an average of 63 % and 67 % of mentors collaborating with their protégés in the STEM fields before and after their protégés’ doctoral dissertations respectively, while an average of 19 % and 21 % of mentors collaborating with their protégés before and after their dissertations in the fields of social sciences and humanities. Grouping scientific fields into STEM and Social in order to test whether the differences in
collaboration were statistically significant shows that they indeed are, with Pearson’s 2 being statistically significant (p < 0,05) for both collaborations before and after protégés’ doctoral dissertations.
TESTING THE HYPOTHESES
Before testing the hypotheses, it is important to note that, as is the case with any large sample, this one is no exception to outlier problems. Although, there are numerous ways of dealing with outliers and “conforming” them to the “normal” data, none of them are without problems. Additionally, because of the specific nature of this research, where outliers, in terms of very (un)successful mentors and protégés, are highly relevant for the analysis and interesting for the interpretation, they will be kept in the dataset. Apart from the study nature, the application of social network analysis was also a reason for this decision, as network analysis requires the studied network account for the largest possible set of data and connections, where eliminating hyper productive scientists would lead to eliminating a large number of connections needed to calculate centrality measures. Before applying linear regressions, it is necessary to check if their use is justifiable or, to be exact, whether the assumptions of independence of observations, homoscedasticity, linearity, and normality are satisfied. In order to test the influence mentors’ average productivity has on their protégés productivity both on the year they attained their PhD (H1), as well as five years later (H1a), two separate linear regressions were conducted. As Table 2 shows, Durbin-Watson statistics for the H1 regression models are 1,969 and 1,978 which indicates that, since the values are close to 2, the residuals of both models are independent and there is no correlation between them, meaning that the assumption of the independence of observations of both models is satisfied. Naturally, as it can be seen in Figure 1, the decision of keeping the outliers in the analysis affected the normality and homoscedasticity assumptions of both models, which means that it cannot be concluded that the spread of models’ variances is equal both for the residuals and the predicted values. However, as it can be seen in the Figure 2, both models show a linear relationship of independent and dependent variables, indicating that the linearity assumption of this regressions is satisfied. Apart from that, these plots offer a perfect graphical depiction of the network’s pronounced outliers. The first linear regression, testing H1, shows that mentors’ productivity is a significant (p < 0,05), positive and moderately strong predictor of their protégés’ productivity at the year of their PhD attainment, with a correlation coefficient of R = 0,488, and R2 = 0,238 (Table 2). Applying linear regression using the same mentors’ variable but testing for its predictability of protégé productivity five years after gaining their PhD, yields similar results with a correlation coefficient of R = 0,512, and an R2 = 0,262 (Table 2). This leads to a conclusion that mentors’
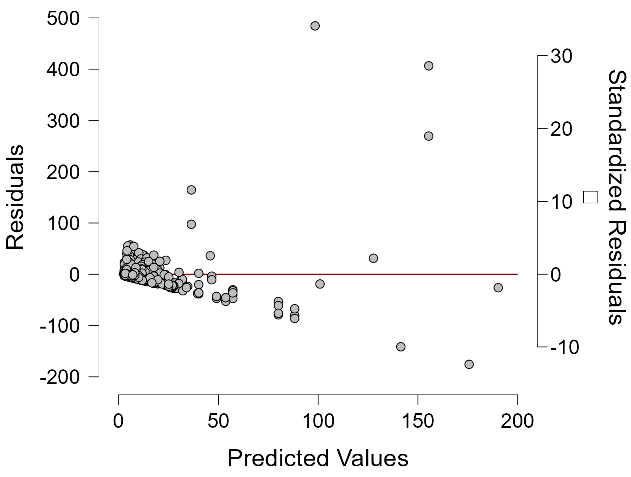
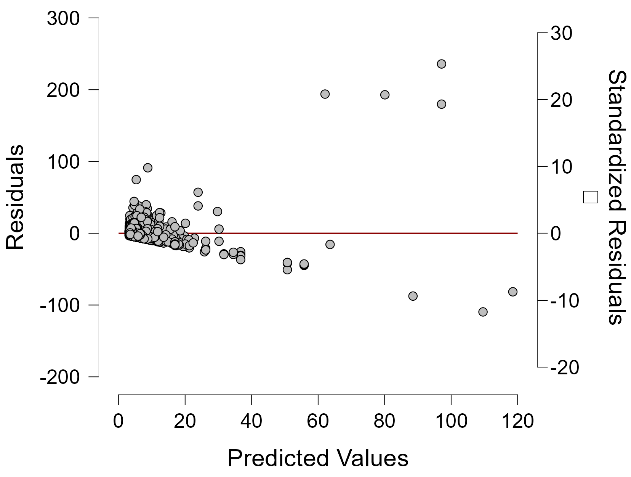
H2 stated that doctoral students who co-authored with their mentors after the completion of their studies will be more productive than those who did not so. In order to test it, a t-test was applied based on subgroups in which doctoral students either did or did not collaborate with their mentors, testing for both collaborations before PhD attainment as well as five years after and their respective effects on the number of published articles on the two respective time periods. As can be seen in Table 4, collaboration with mentors does seem to affect protégé productivity, with both protégés who co-authored articles with their mentors before and after attaining their PhD publishing more than their counterparts.
In order to test H2a, specifically, whether mentors’ “star” status and their collaboration with protégé had any effect on their protégés’ productivity, ANOVA was applied to the data, once again using Welch statistics and Game-Howell post hoc comparisons as equal variances could not be assumed according to Levene’s test. As Table 5 shows, collaborating with one’s mentor seems to lead to a higher productivity, with protégés who engaged in such activities publishing, on average, more than their colleagues who did not choose or did not have an option to do so during and after attaining a PhD. Additionally, if the protégé’s mentor had been assigned a star status, that protégé’s productivity is found to be statistically significantly higher than any other analysed subgroup, with a mean of 10 article published in five years. Perhaps unsurprisingly, protégés who have been mentored by scientists of lower levels of productivity, and who did not engage in co-authorships after attaining a PhD, published the least, and were significantly less productive both compared to the first category, as well as compared to the third category – protégés who, although were not mentored by highly productive scientists, did engage in co-authorships following their graduation. As Table 4 and Table 5 show, both H2 and H2a should be accepted, as mentor-protégé collaborations do seem to have a positive effect on productivity, with protégés who co-authored with their mentors publishing more than their counterparts both before and after attaining their PhD (H2), as well as protégés who collaborated with “star” mentors publishing statistically significantly more than any other examined group (H2a).
Finally, to test whether doctoral students whose mentors were more networked become also more networked themselves (H3 and H3a), linear regression was used again, along with the prior check of the justifiability of its use. Although linear regression was meant to be applied to both betweenness centrality and weighted degree centrality (WDC), exploring correlations between mentors’ and protégés’ betweenness centrality values shows that their Pearson’s r coefficient is low (0,095) so applying linear regression was not seen as methodologically sound. On the other hand, mentors’ WDC is moderately correlated with protégés’ WDC, with a Pearson’s r coefficient of 0,389, justifying the application of linear regression. As it can be seen in Table 6, this regression model’s Durbin-Watson statistic is 0,819 and indicated that the assumption of the independence of observations is satisfied. As was the case with previous regression models, outliers have also impacted this one’s normality and homoscedasticity (Figure 3), but not its linearity which is, together with the differently scattered but equally prominent outliers, shown in the Figure 4. As seen in Table 6, mentors’ WDC values are positively, moderately, and statistically significantly correlated with the WDC values of their protégés, indicating that, unlike betweenness centrality, weighted degree centrality values are, at least in part transitive in their nature. Considering this model’s R2 value of 0,151, it can be concluded that mentors’ weighted degree centrality explains slightly more than 15 % of their protégés’ one.
Model Summary – weighted degree centrality | |||||||
Durbin-Watson | |||||||
Model | R | R² | Adjusted R² | RMSE | Autocorrelation | Statistic | p |
H3 | 0,389 | 0,151 | 0,151 | 35,201 | 0,0904 | 1,8191 | 5,0542·10–9 |
In conclusion, as highly connected (high WDC values) mentors do seem to either use their connections to integrate new scientists into their network or simply function as bridges through which protégés can find co-authors easily, while H3 has to be rejected, H3a can be accepted. As WDC seems to play a role in helping protégés connect with various authors that are networked with their mentors, the same does not apply to the notion of betweenness centrality. Considering the definition of betweenness centrality being a position of “power” in which a specific author is more often a link between any two other specific authors, it is not entirely surprising that such a position is not easily transferrable to new authors, or in the case of this article, protégés. Even though they may be able to gain access to their mentors’ connections (and often they do, as shown in Table 7), they cannot easily move to positions in which they will be the most important bridges between authors, at least not that early in their careers.
STUDY LIMITATIONS AND FURTHER RESEARCH
Although this research focused exclusively on the relationship between young researchers’ and their mentors’ success, since doctoral students often work on scientific project, meaning they learn from more people at once and collaborate with other researchers besides their mentors, it would be interesting to explore the influence of the whole teams and project affiliation on future careers of Croatian doctoral students. Apart from the ever-growing project affiliations of the doctoral students, and their necessarily unique experience of scientific production and collaboration, recently there is also an increasing trend of Scandinavian model (article based) doctorates. This has made, as Lokhtina et al.26 state, co-authorship between doctoral students and their supervisors more relevant than ever. Such encouragement by faculties and other research institutions towards article-based doctoral dissertations should lead to a greater student-mentor collaboration, and consequently to more successful careers of young researchers and their institutions’ better reputation and positioning, which is also something that should be further explored. Additionally, some research findings show that scientists become the most successful when they gain intellectual independence form their mentors23; p.14077 and that, although there is a positive influence of “star” scientists on their protégés’ careers in early stages, that influence weakens over time and may even become a negative one21; p.1848. Hence, further research could also explore whether this was true in the Croatian context and whether researchers who, for instance, 10 years after gaining their PhD collaborate less with their mentors are indeed more successful and recognized than those who collaborate more and are, as Qi et al.21 put it, still in the shadow of a “star”. On the lines of mentor protégé collaboration after PhD attainment, it would be interesting to explore the presence of “gaming” the system or a form of shadow writing in which mentors are possibly included as authors in protégés’ future articles without actually contributing to them. Such a practice could be very well present, and it could have led to a overrepresentation of mentor-protégé collaborations but it was impossible to test for given the available data and could be further explored both in terms of questionnaires aimed directly to protégés or by conducting interviews to understand mentor-protégé collaborations and power relations better. In addition to all the above mentioned further research notes, it would also be interesting to inspect whether are there any differences across the scientific disciplines, as there are in their research and collaboration patterns in general. Finally, two limitations of this study are linked to its sample. While the first one concerns the very management system of the publication repository aggregator CROSBI, the second one is linked to a few specific cases of PhD students having more than one mentor. The first limitation as authors themselves oversee the updating of their profiles, the database could be incomplete or have some of the articles mislabelled. Such a practice could potentially have little repercussions on the profiles of younger researchers and those who are still in the process of advancing in their scientific positions as most universities ask their employees to keep their profiles updated and use them in career advancement evaluation while tenured professors could be found updating their profiles much less regularly. The second limitation, as explained in the chapter regarding sample and data collection, regards the few cases of students with more than one mentor. In such cases only the most productive and networked mentor has been retained in order to better explore correlations between most productive mentors.
CONCLUSIONS
The main goal of this article was to explore the interactions between PhD mentors and protégés in terms of productivity and networking, expecting that highly productive mentors will turn their protégés into highly productive authors via mechanisms of information sharing, good practice and introducing their protégés into their network of both strong ties (in terms of repeated co authorships) as well as weak ties (in terms of access to information outside their close vicinity). Mentor-protégé collaboration has been shown to be very impactful in terms of boosting protégés’ productivity during their doctoral study, but, and perhaps more importantly, it shows long term effects, as protégés who had highly productive mentors will continue to be highly productive even five years after their graduation, corroborating the findings of Li et al.8. Such a boost in productivity is not tied exclusively to being mentored by capable and productive scientists who will show their protégés all the “tricks” and good practices in getting published often and easily. It seems to be very much tied to both mentors and protégés engaging in co-authorships, both during the period of doctoral study, as well after the protégé has attained his/her new title. As this analysis shows, protégés that co-authored articles with their mentors published, on average, almost twice as much as those who did not during their doctoral study, and more than twice as much upon study completion. Such results are in line with findings of Li et al., Sekara et al., as well as Hu and Racherla and Hakkarainen et al., and Costa2,8,17,20,22, who show the impact mentoring in both a practical way (by “learning the ropes” of publishing practices) as well as in a social-capital way (by borrowing a mentor’s social capital in order to gain one own’s). And while mentor productivity seems to function well as a predictor of protégé productivity, collaboration could be interpreted as an equally, if not more important factor. Taking into account the results shown in Table 5, star mentors’ collaboration with their protégés will lead to protégés publishing an extraordinary number of articles, but protégés who had star mentors, and did not co-author articles with them upon completing their studies will, on average, publish just half as much as their “luckier” colleagues. And while that difference in the number of publications is statistically significant, another one, although not statistically significant, is perhaps more interesting in terms of exploring the connections between collaboration and mentor productivity, as it concerns the difference between protégés who had star mentors with whom they did not collaborate, and protégés who had “normal” mentors with whom they did collaborate. On average the former group published five articles in five years, while the latter published just a bit more, six articles in five years. Such a difference in the number of publications should be explored further as it did not appear to be statistically significant, but, at the same time could indicate the real value of collaboration in early career phases. Finally, exploring network values has shown that different centrality measures do indeed offer different opportunities to protégés. While betweenness centrality did not “pass the test” and was not included in linear regression analysis due to its low Pearson’s r values, that very result could be interpreted as a specific characteristic of betweenness, as a non-transitive measure which is strongly tied to an author that owns it and cannot readily “give it away”. On the other hand, weighted degree centrality, a value that measures an author’s connectedness to its scientific social surrounding in terms of (repeated) co-authorships does seem to be more transitive and seems to help newly fledged scientists in finding co-authors in their mentors’ networks, supporting Latour’s and Woolgar’s20 idea of “borrowing” social capital from one’s mentors. In conclusion, picking a mentor for one’s own doctoral dissertation seems to be a decision that will have consequences that are more far reaching than the immediate need to be mentored and attain a doctoral title. Collaborating with their mentors and picking up good practices in publishing will influence protégés’ careers much more than up to the point of “merely” becoming a philosophy doctor.
REMARKS
Footnotes:
[1] Betweenness centrality can easily be defined as an extent to which an author is in the shortest path between another pair of co-authors8.
[2] With degree centrality representing the number of co-authors a researcher has, and weighted degree centrality taking into account repeated collaborations with such co-authors.
[5] 5In order to compute a denser network than the one composed solely of scientific papers, it included authored books, edited books, book chapters, journal articles, conference proceedings papers, and conference abstracts.
Petersen A.M.: Quantitative and empirical demonstration of the Matthew effect in a study of career longevity. The Proceedings of the National Academy of Sciences,108(1),18-23, 2010.http://dx.doi.org/10.1073/pnas.1016733108
Hakkarainen K., et al.: How Does Collaborative Authoring in Doctoral Programs Socially Shape Practices of Academic Excellence?. Talent Development & Excellence,6(1),11-29, 2014.https://d-nb.info/105203893X/34
Acuna D., Allesina S., Kording K.: Predicting scientific success. Nature,489,201-202, 2012.http://dx.doi.org/10.1038/489201a
Musselin C.: New forms of competition in higher education. Socio-Economic Review,16(3),657-683, 2018.http://dx.doi.org/10.1093/ser/mwy033
Kamler B.: Rethinking doctoral publication practices: writing from and beyond the thesis. Studies in Higher Education,33(3),283-294, 2008.http://dx.doi.org/10.1080/03075070802049236
Merton R.K.: The Matthew Effect in Science: The reward and communication systems of science are considered. Science,159(3810),56-63, 1968.http://dx.doi.org/10.1126/science.159.3810.56
Billah S.M., Gauch S.: Social Network Analysis for Predicting Emerging Researchers. Proceedings of the 7th International Joint Conference on Knowledge Discovery, Knowledge Engineering and Knowledge Management,1,27-35, 2015.https://ieeexplore.ieee.org/document/7526900
Li W., et al.: Early coauthorship with top scientists predicts success in academic careers. Nature Communications,10(1),1-9, 2019.https://www.nature.com/articles/s41467-019-13130-4
Henriksen D.: The rise in co-authorship in the social sciences (1984-2013). Scientometrics,107(2),455-476, 2016.http://dx.doi.org/10.1007/s11192-016-1849-x
Kuld L., O’Hagan J.: Rise of multi-authored articles in economics: Demise of the ’lone star’ and why?. Scientometrics,114,1207-1225, 2018.http://dx.doi.org/10.1007/s11192-017-2588-3
Nabout J.C., et al.: Publish (in a group) or perish (alone): the trend from single to multi-authorship in biological articles. Scientometrics,102(1),357-364, 2015.http://dx.doi.org/10.1007/s11192-014-1385-5
Iglič H., et al.: With whom do researchers collaborate and why?. Scientometrics,112,153-174, 2017.http://dx.doi.org/10.1007/s11192-017-2386-y
Brajdić Vuković M., et al.: STEM colonization: Applying hard sciences’ socio-organisational patterns and evaluation procedures to the soft sciences in Croatia. European Journal of Education,55(4), 2020.http://dx.doi.org/10.1111/ejed.12421
-:Ordinance on the conditions for election to scientific titles (OG 84/2005). In Croatian.,https://narodne-novine.nn.hr/clanci/sluzbeni/2005_07_84_1633.html 2022.
-:Ordinance on the conditions for election to scientific titles (OG 26/2013). In Croatian,https://narodne-novine.nn.hr/clanci/sluzbeni/2013_03_26_447.html 2022.
-:Ordinance on the conditions for election to scientific titles (OG 28/2017). In Croatian,https://narodne-novine.nn.hr/clanci/sluzbeni/2017_03_28_652.html 2022.
Hu C., Racherla P.: Visual representation of knowledge networks: A social network analysis of hospitality research domain. International Journal of Hospitality Management,27(2),302-312, 2008.http://dx.doi.org/10.1016/j.ijhm.2007.01.002
Abbasi A., et al.: Egocentric analysis of co-authorship network structure, position and performance. Information Processing and Management,48(4),671-679, 2012.http://dx.doi.org/10.1016/j.ipm.2011.09.001
Beaver D.D.: Does collaborative research have greater epistemic authority?. Scientometrics,60(3),399-408, 2004.http://dx.doi.org/10.1023/B:SCIE.0000034382.85360.cd
Costa M.R.: The Dynamics of Social Capital in Scientific Collaboration Networks. Proceedings of the American Society for Information Science and Technology,51(1),1-4, 2014.http://dx.doi.org/10.1002/meet.2014.14505101137
Qi M., et al.: Standing on the shoulders of giants: the effect of outstanding scientists on young collaborators’ careers. Scientometrics,111(3),1839-1850, 2017.http://dx.doi.org/10.1007/s11192-017-2328-8
Sekara V., et al.: The chaperone effect in scientific publishing. The Proceedings of the National Academy of Sciences,115(50),12603-12607, 2018.http://dx.doi.org/10.1073/pnas.1800471115
Ma Y., Mukherjee S., Uzzi B.: Mentorship and protégé success in STEM fields. The Proceedings of the National Academy of Sciences,117(25),14077-14083, 2020.http://dx.doi.org/10.1073/pnas.1915516117
Lokhtina I., et al.: In pursuit of sustainable co-authorship practices in doctoral supervision: Addressing the challenges of writing, authorial identity and integrity. Innovations in Education and Teaching International,59(1),82-92, 2022.http://dx.doi.org/10.1080/14703297.2020.1799839