Introduction
One of the most important components of the dairy cattle production system is the knowledge of pregnancy status of the herd. This information is helpful in avoiding wasted re-inseminations, shortening the open days, and culling the cows, thus increasing the herd productivity (Ferguson and Gallian, 2011; Giordano et al., 2013; Tiplady et al., 2021). Different methods of pregnancy detection are by veterinary practitioners. These may be direct or indirect methods. For example, during the mating period, pregnancy status can be determined by non-return rate or indirectly by milk progesterone level and measurements of pregnancy associated glycoprotein in milk and blood, or by using more invasive techniques such as transrectal palpation and ultrasonography. Each method for determination of the pregnancy status has its own advantages and disadvantages. The milk progesterone and pregnancy associated glycoprotein tests are not cost effective and may give misleading results due to reproductive problems. The determination of non-return rate is not so reliable if the farm does not use strict estrus determination methods (Ricci et al., 2017; Tiplady et al., 2021). Pregnancy diagnosis by milk progesterone measurement at day 24 has an accuracy of 83.3 % (Muhammed et al., 2000) and 85 % (Sheldon and Noakes, 2002). However, by using milk component determination, it will be faster to detect or confirm pregnancy during routine milk recording can be helpful in maintaining the farm records on the pregnancy status of dairy cows. Moreover, this method is cost-effective and non-invasive (Brand et al., 2020). Therefore, different pregnancy detection methods can be useful for breeders.
Bovine milk reflects not only milk related disorders but also metabolic disorders such as ketosis (De Roos et al., 2007). Because cow's milk is affected by metabolism, it can be used to determine other conditions that affect metabolism. Pregnancy, one of the major physiological events, affects metabolism through hormonal and metabolic changes by meeting the needs of the growing fetus (Bell et al., 1995; Sguizzato et al., 2020). Comparing pregnant and non-pregnant lactating animals, the reserves can be used by different body functions. Besides, it is already known that pregnancy in mid and late lactation causes changes in milk composition in dairy cows (Olori et al., 1997; Loker et al., 2009; Penasa et al., 2016). Moreover, hormones are released from the fetal-placental unit around day 100 of pregnancy (Bachman et al., 1988). These hormonal differences under the influence of pregnancy increase the regression of the mammary gland (Brotherstone et al., 2004) and the milk yield shows a decrease after 4-5 months of pregnancy (Bachman et al., 1988; Olori et al., 1997; Roche, 2003). As lactation progresses, the changes in milk composition might coincide with a cow becoming pregnant. Thus, the analysis of milk composition may be useful in determining the pregnancy status of dairy cows.
In the present study, we intended to investigate if the determination of possible pregnancy-related changes in the milk components during the routine sampling can be effectively used to detect pregnancy in dairy cows. Thus, the aim of the present study was to evaluate the diagnostic accuracy of milk components to determine pregnancy and its usability at farm level in the mid and late lactation cows.
Material and methods
Milk samples were collected according to the "Regulation on Studying Procedures and Principles of Animal Experiments of Ethics Committees" of the Ministry of Agriculture and Forestry (2014, Republic of Turkey) and the regulations of Animal Experiments Local Ethics Committees of Hatay Mustafa Kemal University. In addition, necessary approvals were obtained from the enterprise.
Farm and management
The study was conducted in Saray Agriculture and Livestock Inc. in Kayseri, Turkey. The barns were naturally ventilated and lighted artificially. The cows were fed a total mixed ration (TMR) according to meet their nutritional requirements as recommended by the NRC (NRC, 2001). The TMR was prepared daily using a vertical mixer and offered three times in equal portions. The composition of the TMR is shown in Table 1.
Table 1. Chemical composition and ingredients of ration
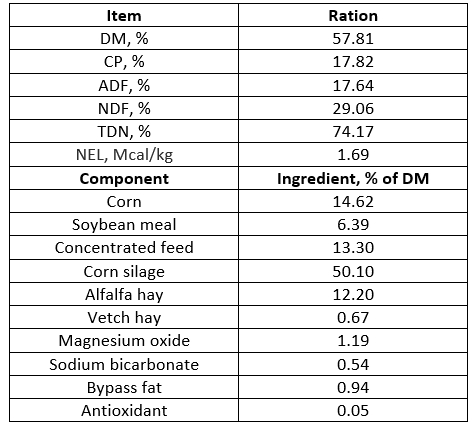
Animals and grouping
Healthy primiparous Holstein cows were examined in the study. The animals were grouped according to their lactation period. As stated by Yoon et al (2004), the lactation of cows was classified as mid (100-200 days in milk) and late lactation (>200 days in milk). Sixty-one of the Holstein cows (N non-pregnant=41 and N pregnant=20) were in the mid-lactation and seventy-two of the Holstein cows (N non-pregnant=37 and N pregnant=35) were in the late lactation. Pregnancy status was determined by an experienced farm veterinarian and recorded in the herd tracking system.
Milk sampling and determination of milk quality parameters
Cows were milked three times, and the milk yield was recorded daily by an automated milking system. Milk samples were collected during the morning milking with an automated milking system (AfiMilk, Afkim, Israel) in the enterprise. All mammary lobes of the cows were checked for mastitis by the veterinarian of the enterprise using the California Mastitis Test (CMT) before milk sampling. Teat end cleaning was performed with 70 % ethanol and fore-milk was discarded before sampling. Samples were collected from each quarter in sterile falcons with a volume of 50 mL. Analyses of the quality parameters of the milk samples were performed in the enterprise laboratory.
The samples were prepared according to the protocol of the Lactoscan SCC kit (18.05.2021/R) for the determination of milk somatic cell count and measured with a milk somatic cell counter (Lactoscan SCC 6010, Bulgaria). Approximately 5 mL of milk was collected and transferred to tubes for determination of milk pH. The pH of the milk was measured using a pH probe (Hanna pH meter, HI83141, USA). Fat, fat-free dry matter, protein, lactose, freezing point and electrical conductivity which are the main quality parameters of milk were measured using a milk analyzer (Milkotester Master Classic LM2-P1, Bulgaria). In addition, somatic cell count and composition of milk were determined by validated equipment.
Statistical analysis
Statistical analyses were performed using Stata SE version 15.1 and MedCalc version 9.2.0.1. To determine the parametric test assumptions, variables were evaluated using the Kolmogorov-Smirnov test and the Levene test. SCC data were normalized and transformed to Somatic Cell Score (SCS=log2 (SCC/100.000) + 3) (Ali and Shook, 1980) after measurement of SCC. Descriptive statistics were presented as "Mean ± Standard Error of Mean (SEM)". In each lactation period (mid and late lactation), differences in milk quality parameters and milk yield according to pregnancy status were evaluated using Student's t test. Receiver operator characteristics (ROC) analysis was used to determine a predictive threshold with milk quality parameters (SCC, fat, fat-free dry matter, protein, lactose, freezing point, electrical conductivity and pH) for differentiation of the pregnancy status in each lactation period. ROC curves for detection of pregnancy were obtained for each milk quality parameter. Sensitivity, specificity, likelihood ratio, 95 % confidence interval and area under the curve (AUC) were calculated for each variable. AUC values of = 1, >0.9, 0.7-0.9 and 0.5-0.7 indicated excellent accuracy, high level of accuracy, moderate accuracy and poor accuracy, respectively (Zakian et al., 2018). The thresholds of milk quality parameters for determining pregnancy status were presented as a figure in each lactation period as the result of ROC analysis. Statistical significance was considered as p<0.05.
Results and discussion
The aim of the present study was to evaluate the usability of milk composition traits for pregnancy diagnosis. The use of milk composition parameters can be useful tool for detecting pregnancy status during the routine milk examinations. The use of these parameters in routine on-farm milk controls can be beneficial to the enterprise by providing an idea about pregnancy. Prediction accuracy of pregnancy is useful for preliminary screening (Toledo-Alvanado et al., 2018). As described by Toledo-Alvanado et al., (2018), pregnancy diagnosis could be performed by milk components (Figure 1 and 2).
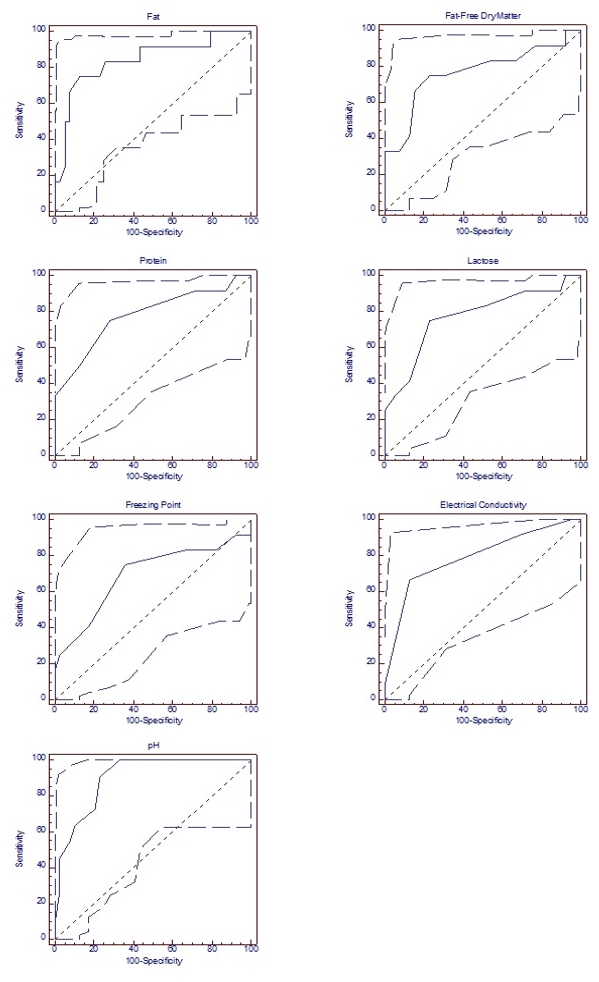
Figure 1. The ROC curve analysis determined the cut-off value for fat, fat-free dry matter, protein, lactose, freezing point, electrical conductivity and pH for predicting the pregnancy in the mid lactation
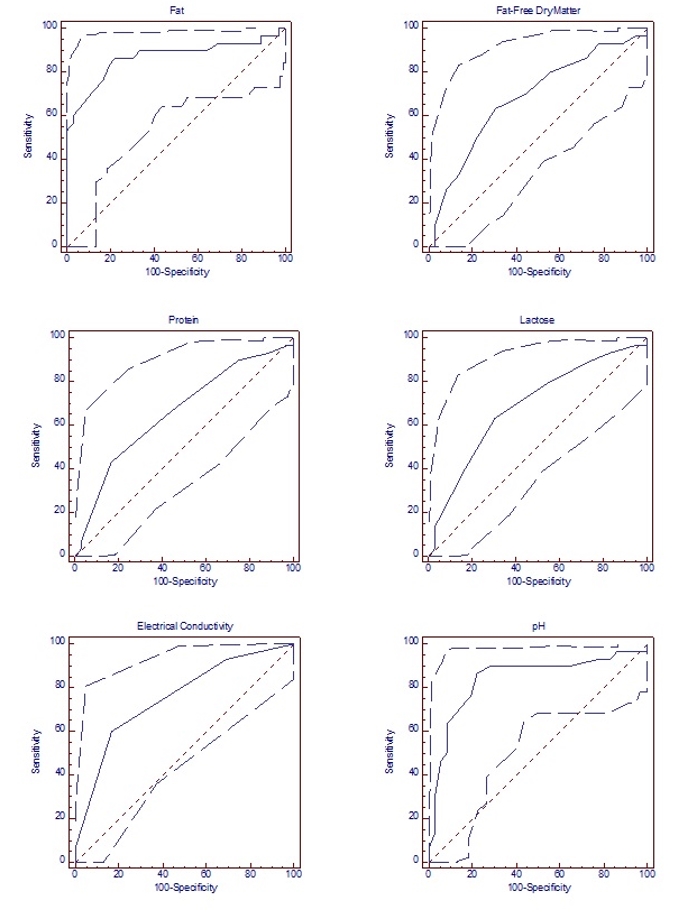
Figure 2. The ROC curve analysis determined the cut-off value for fat, fat-free dry matter, protein, lactose, electrical conductivity and pH for predicting the pregnancy in the late lactation
The average milk yield of cows was 26.17±0.67 kg/day, which was lower than in the study performed by Bohmanova et al. (2009). A possible reason for such difference could be the selected period of lactation for the analysis. As lactation progresses, milk production decreases physiologically. Thus, the animals in the present study were in mid and late lactation cows and this might be the effect of the difference between the studies. On the other hand, the mean milk yield during the lactation was different in pregnant and non-pregnant cows. The possible explanation in such difference could be the effect of gestation (hormonal differences and energy usage of the fetus) as previously described by (Oltenacu et al., 1980; Brotherstone et al., 2004; Leclerc et al., 2008; Bohmanova et al., 2009).
The milk quality parameters according to pregnancy status in the mid and late lactation are summarized in Tables 3 and 4, respectively. SCC was higher in the pregnant group than in the non-pregnant group, but there was no statistically significant difference for mid lactation cows (p>0.05). Similarly, SCC was not different between pregnant and non-pregnant cows in the late lactation group. Increase in somatic cell count is the gold standard for determination of udder infection. High SCC counts may inhibit the corpus luteum function and shorten the pregnancy period due to insufficient progesterone release from the corpus luteum. Due to the infection of udder, increased inflammation metabolites and SCC may induce premature calving. On the other hand, advanced pregnancy period reduces the milk somatic cell count, which has not effect on fetus development (Isobe et al., 2014). Even the present study showed that the SCC count was similar in both groups and lower than the threshold for mastitis. Although, lactation period has an effect on milk composition as well as pregnancy status on these parameters, the results showed that SCC had no impact on pregnancy status, similar to studies Loker et al., (2009) and Penasa et al., (2016).
Table 2. Milk quality parameters in pregnant and non-pregnant cow groups (Regardless of lactation period)
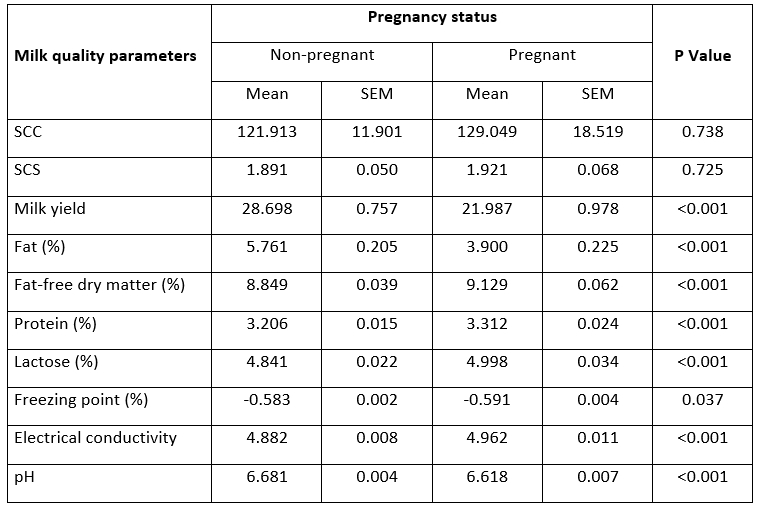
SEM: standard error of mean
Table 3. Milk quality parameters in the mid lactation
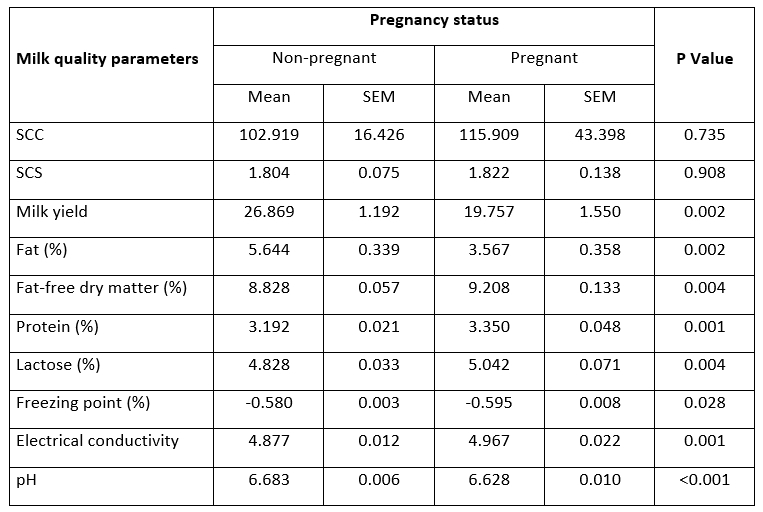
SEM: Standard error of mean
Table 4. Milk quality parameters in the late lactation
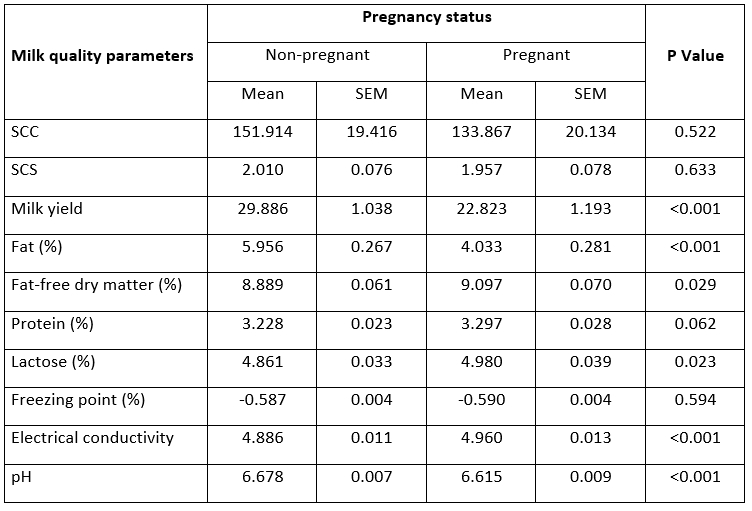
SEM: Standard error of mean
Milk fat, freezing point and pH parameters were observed to be greater in the non-pregnant group compared to the pregnant group (p<0.05) in both mid and late lactation cows (Table 3 and 4). Similar results were obtained by Penasa et al. (2016). As Rodriguez et al. (1985) previously described, the fat content of milk increases as pregnancy progresses. The present study showed different results from this finding. The explanation for this difference could be the day of pregnancy. Since, Penasa et al., (2016) showed that the fat composition of the milk decreased from day 1 to 120 of pregnancy. Olori et al., (1997) reported that milk fat content increased after the 6th month of pregnancy. However, according to the results of the present study, there might be changes in milk parameters in different lactation periods regardless of the day of gestation. Contrary to Toledo-Alvaeado et al., (2018), their study explains that milk fat content is not enough for pregnancy diagnosis, but the present study showed a higher AUC value (Table 5 and 6) (Figure 1 and 2). On the other hand, protein content was informative for determination of pregnancy status, which is similar to the present study, regardless of lactation period and in mid lactation cows. The reason for this difference between the studies was the animal selection method. They used animals only ≤91 days after insemination.
Several factors can affect the freezing point of milk of dairy cows. However, Wiedemann et al. (1993) already explained that milk freezing point was not affected by energy or protein concentration of the ration. Besides, all cows in the present study were fed in similar rations. However, Bjerg et al., (2005) stated that milk freezing point was influenced by water intake and increased temperature and sunshine hours. However, in the present study, all milk samples were collected at the same time. Furthermore, only mid lactation pregnant cows showed lower freezing point (Table 3). However, late lactation pregnant and non-pregnant cows showed similar freezing points (Table 4). The possible explanation for this difference could be the effect of lactation on milk composition. On the other hand, lactose might have a role on the milk freezing point. Findings of some previous studies indicated that high lactose content results in lower milk freezing point (Bjerg et al., 2005; Kedzierska-Matysek et al., 2011; Costa et al., 2017). In this study, lactose levels were higher in mid lactation pregnant cows. This could be a reason for the lower milk freezing point. Because lactose differences were greater in mid lactation cows in comparison to late lactation cows.
Milk pH decreased slightly in pregnant cows in the mid and late lactation (Table 3 and 4). Milk acidity increases in the early stages of pregnancy and then decreases as gestation progresses (Rodrigues et al., 1985). Similarly, Bohmanova et al., (2009) and Penasa et al., (2016) showed an increase in acidity at early stage of gestation. However, in the present study, pregnancy stage was not evaluated. Only routine sampling days for milk analysis were used to determine pregnancy status. In the mid and late lactation, milk pH is a useful biomarker for determination of pregnancy status with the AUC of 0.901 and 0.841, respectively (Table 5 and 6) (Figure 1 and 2).
Fat-free dry matter, protein, lactose and electrical conductivity parameters were higher in the pregnant group (Table 2). In the present study, protein content increased significantly regardless of lactation period. Mid lactation cows showed increase in milk protein content (Table 3). However, milk protein levels were similar in pregnant and non-pregnant late lactation cows (Table 4). The possible differences among these results between groups could be due to the distribution of pregnancy days between groups. It has previously been reported that milk protein content of cows increases with the number of months of pregnancy. The present study supports this finding. The possible explanation for the increase in milk protein content could be the increase in estrogen due to placental release. Increased estrogen concentration decreases milk yield and increases the percentage of milk protein (Hutton, 1958; Parkhie et al., 1966). Lactose concentration in pregnant and non-pregnant animals regardless of lactation status was significantly different (p<0.05) (Table 2). When compared in late and mid lactation group, milk lactose concentration was higher in pregnant animals (Table 3 and 4). This finding similar to Penasa et al., (2016). The increase in previous studies was recorded after 5th month of gestation (Olori et al., 1997; Roche, 2003). A possible reason for the increase could be the udder glucose utilization mechanism. The udder uses glucose independently of insulin. Thus, the increased glucose level to meet the needs of pregnancy may have turned into lactose in the udder tissue, where it is transported directly through the blood, resulting in increased lactose concentration in the milk. The electrical conductivity of the milk may change depending to the health status of the cows. These changes are mostly associated with mastitis (Nielen et al., 1995; Norberg et al., 2004). However, de Mol (2000) described that milk electrical conductivity was associated with other disorders. Moreover, the present study showed that electrical conductivity during the mid and late gestation was associated with pregnancy status of dairy cows (Table 3 and 4).
Table 5. The ROC analysis results of the mid lactation
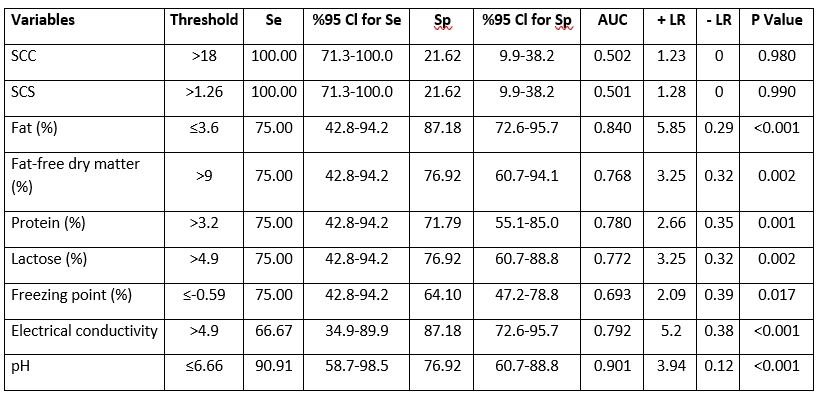
Se: Sensivity; Sp: Specifity; CI: Confidence interval; AUC: Area under the curve
Table 6. The ROC analysis results of the late lactation
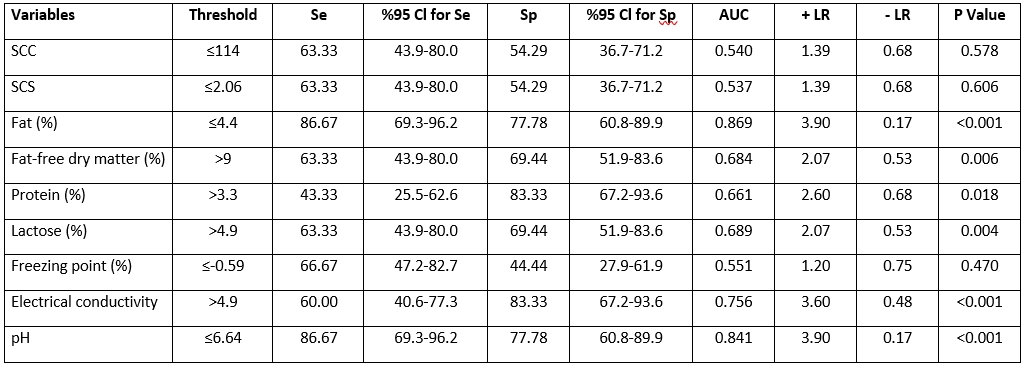
Se: Sensivity; Sp: Specifity; CI: Confidence interval; AUC: Area under the curve
Electrical conductivity has a higher likelihood ratio as well as high AUC values for mid and late lactation cows (Table 5 and 6) (Figure 1 and 2). Milk electrical conductivity was lower in pregnant cows in comparison to non-pregnant cows (Table 2). The reason for this change was directly related to milk fat concentration. The decrease in milk electrical conductivity was related with increased milk fat content (Woolford et al., 1998). Besides, milk electrical conductivities of all cows were within physiological ranges (Norberg et al., 2004) for pregnant and non-pregnant cows in mid and late lactation (Table 3 and 4).
Conclusion
Milk pH, fat, electrical conductivity, protein, lactose, FFDM, and freezing point were the best predictors for diagnosing of pregnancy status with the AUC values (0.901, 0.840, 0.792, 0.780, 0.772, 0.768 and 0.693 respectively) in the mid lactation. Furthermore, fat, pH, electrical conductivity, lactose, FFDM, and protein could be a useful diagnostic tool for pregnancy with the AUC values (0.869, 0.841, 0.756, 0.689, 0.684 and 0.661 respectively) in the late lactation. The current study demonstrates that analysis of milk composition could be useful for determining pregnancy status and prediction of pregnancy directly from milk composition seems practical.
Funding
This work was supported by the Scientific Research Project Fund of HATAY MUSTAFA KEMAL ÜNİVERSİTESİ under the project number 21.GAP.004.
Dijagnostička točnost sastojaka mlijeka kod dijagnostike gravidnosti u krava srednje i kasne laktacije
Sažetak
Ciljevi ove studije bili su utvrditi granične vrijednosti procjenom mogućnosti upotrebe broja somatskih stanica (SCC) i pojedinih fizikalno-kemijskih parametara mlijeka (udio masti, bezmasne suhe tvari (FFDM), proteina, laktoze, točke zamrzavanja, električne vodljivosti i pH) za promatranje statusa gravidnosti kao i u svrhu korištenja navedenih parametara kao bioloških markera u dijagnozi statusa gravidnosti. U ovu su studiju bile uključene prvotelke holstein pasmine (n=133) u srednjoj i kasnoj laktaciji. Uzorci mlijeka sakupljani su u sterilne epruvete za analizu SCC i fizikalno-kemijskih parametara. U svakom razdoblju laktacije, SCC, prinos mlijeka i fizikalno-kemijski parametri analizirani su Student t-testom u odnosu na status gravidnosti. Za određivanje prediktivnog praga korištene su krivulje odnosa specifičnosti i osjetljivosti klasifikatora (ROC), korištenjem SCC i fizikalno-kemijskih parametara mlijeka za razlikovanje gravidnih i negravidnih krava. Za sve krave u srednjoj i kasnoj laktaciji razine SCC bile su slične. Sredinom laktacije, FFDM, udjeli proteina i laktoze te električna vodljivost bili su viši, a prinos mlijeka, udio masti, točka ledišta i pH bili su niži u gravidnih krava (p<0,05). U kasnoj laktaciji, FFDM, udjeli proteina i laktoze te električna vodljivost bili su značajno viši, a prinos mlijeka, udio masti i pH bili su značajno niži u gravidnih krava (p<0,05). Udjeli masti, proteina i laktoze, FFDM, točka ledišta, električna vodljivost i pH bili su najbolji prediktori za dijagnozu gravidnosti kod krava u srednjoj laktaciji s vrijednostima površina ispod ROC krivulje (AUC) 0,840, 0,768, 0,780, 0,772, 0,693, 0,792 i 0,901. Udjeli masti, proteina i laktoze, FFDM, električna vodljivost i pH mogu biti korisni dijagnostički alati za određivanje gravidnosti kod krava u kasnoj laktaciji s AUC vrijednostima 0,869, 0,684, 0,661, 0,689, 0,756 i 0,841. Zaključno, komponente mlijeka mogu se koristiti kao brzi, lako dostupni i jeftini markeri za procjenu dijagnoze statusa gravidnosti kod prvotelki holstein krava.
Ključne riječi: AUC; holstein krave; laktacija; komponente mlijeka; ROC analiza
References
https://doi.org/10.3168/jds.S0022-0302(80)82959-6
https://doi.org/10.3168/jds.S0022-0302(88)79638-1
https://doi.org/10.3168/jds.S0022-0302(05)73001-0
https://doi.org/10.3168/jds.2008-1782
https://doi.org/10.3168/jds.2020-18367
https://doi.org/10.1016/j.livprodsci.2003.07.014
https://doi.org/10.1677/joe.0.0170121