UVOD
Forests are ecologically and economically one of the most important parts of the terrestrial ecosystem. Forests, a carbon sink and an energy source in nature, play a critical rolein maintaining the ecological balance. However, forests are endangered by fires, which not only destroy vegetation and reduce carbon storage but also forest structure. Forest fires are ecologically responsible for carbon emissions, global warming, deforestation, and desertification (Sivrikaya and Kucuk 2022) but also contribute to global warming due to carbon emissions (Bustillo Sánchez et al. 2021). Forest fires cause loss of life as well as economic and natural resource losses every year (Rosavec et al. 2013).
Forest fires are a disaster in the dry season in conifer forest regions such as in the south of Türkiye (Goltas et al 2017). According to past fire records (from 1988 to 2020) in Türkiye, the annual average number of hectares burned was 10806, but in 2021, 139503 ha of forest area was burned (i.e., 13 times the annual average) (GDF 2022). The damage caused by fires in extreme meteorological conditions is greater due to climate change (Kucuk and Sevinc 2023). Therefore, the importance of modeling and mapping the potential of forest fires is obvious. This will allow for effective planning of firefighting efforts, making quick and focused decisions, and allow the implementation of emergency measures.
Forest fire danger has increased in Mediterranean countries such as Türkiye, Greece, Spain, Italy, Portugal, and France (Quintano et al. 2011, Bilgili et al. 2021). The term fire danger refers to the growth of the fire, the difficulty of its control, and the potential damages (NWCG 1996, Saglam et al. 2008). In countries whose forests are threatened by fire, fire danger potential models are developed using environmental factors (Cipriani et al. 2011, Pereira et al. 2012, Kucuk et al. 2015). Models estimating Forest Fire Danger Potential (FFDP) have been developed in various regions that have different fuel and climatic conditions around the world (Kucuk and Bilgili 2007, Bilgili et al. 2019a, Baysal 2021).
Modeling and mapping of FFDP requires complex temporal and spatial analyses (Saglam et al. 2008). Because FFDP is affected by complex temporal and spatial processes in combination with fixed and changeable environmental factors, such as fuel characteristics, topography, and weather conditions (Kucuk and Bilgili 2008; Yavuz et al. 2018; Bilgili et al. 2019b, Sevinc et al. 2020, Kucuk et al. 2021).
Estimation of FFDP is a complex problem because there are many main and sub-criteria to be considered in the decision process. FFDP is estimated with different tools and methodologies to reach the most reliable results. Some researchers have used regression analysis and logistic models (Andrews et al. 2003, Wang et al. 2018), Maxent models (Goltas 2022), Bayesian network model (Sevinc et al. 2020), and Neural Network Model (Liang et al. 2019). Researchers have used multi-criteria decision-making approaches in most of the studies of fire risk and danger estimation in recent years since this approach (MCDM) is a flexible and easily applicable method. Also, combining MCDM with GIS is an effective method for modeling and mapping FFDP (Gheshlaghi et al. 2020, Sivrikaya and Küçük 2022). The use of Geographic Information Systems (GIS) allows the effective and economic distribution of resources.
Although there are some studies estimating FFDP (Saglam et al. 2008, Gungoroglu 2017), there have not been enough studies on FFDP modeling and mapping in the Mediterranean basin, which is dominated by Pinus brutia and maquis species vulnerable to fire.
Saglam et al. (2008) conducted a study in the Korudag forest planning unit in northwestern Türkiye; they estimated the Fire Danger Potential by using variables such as species composition, stand development age, canopy cover, slope, and aspect. Laneve et al. (2020) developed the Fire Danger Potential Index in Sardinia, Italy, using variables of wind speed, topography, and local solar radiation (aspect). Sharples et al. (2009) used only meteorological parameters to create the Fire Danger Index in the central USA.
The use of meteorological parameters in addition to fuel properties and topography variables in the prediction of FFDP will increase the accuracy, because there is a close relationship between forest fires and meteorological factors. The factors affect fire ignition, fire severity, spreading rate, and the difficulty of fire control (Deeming 1972, Kucuk and Sağlam 2004). Therefore, it is critical to use meteorological factors in fire danger models. In this paper spatial and temporal (i.e., monthly) evaluations were used because forest FFDP changes as meteorological factors change. Also, we preferred to evaluate FFDP on a monthly basis using field verification, as we think it may be more useful to fire managers.
The objectives of this paper were (i) to model and map the monthly forest fire danger potential using GIS-based AHP that is one of the most common MCDM techniques, (ii) to make field verification of model results, and (iii) to assess (reveal) the FFDP change on a monthly basis. This study can be used for effective fire prevention and suppression, rapid decision making and implementation of emergency measures.
MATERIJALI I METODE
Study area – Područje istraživanja
The study was conducted within the Muğla Regional Directorate of Forestry (RDF) in southwestern Türkiye (Figure 1). Fifty-six percent (1,158,925 ha) of the Muğla RDF, which has a total of 2,051,212 ha, is covered with forests. The vegetation is composed of Calabrian pine ( Pinus brutia Ten.), Anatolian black pine ( Pinus nigra Arnold), cedar ( Cedrus libani A. Rich.), stone pine ( Pinus pinea L.) , oak ( Quercus spp.), Turkish sweetgum ( Liquidambar orientalis Mill.) and eucalyptus ( Eucalyptus camaldulensis Dehn.) trees, and the shrubs that form the maquis. Different tree species have varying levels of vulnerability or resistance to fire. Forests in Türkiye, especially consisting of Calabrian pine and Anatolian black pine, as well as of some maquis species, have a high fire risk and danger potential.
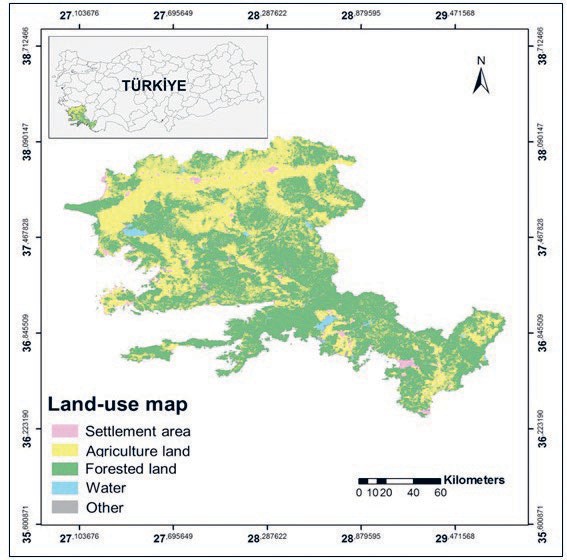
Figure 1. Land-use map of the study area Slika 1. Karta područja istraživanja
(Mutch and Quigley 1993, Kucuk et al. 2005). In addition, sixty-seven percent of the forests of the study area are below 500 meters altitude. Therefore, there is dense dead and living cover, and this situation increases the fire risk and danger potential. Human activities in and near these forests are common because agricultural areas are intertwined with the forests; the area is one of the most popular tourist destinations in Türkiye and has many facilities for tourists. There are many mining sites nearby, construction takes place near the forests, and the number of wind turbines in the area is increasing day by day. The increase in human activities has increased the risk of anthropogenic forest fires. In 2021, 369 fires broke out in the study area and a forest area of 52,219 ha was damaged by fire (GDF 2022).
Variables and data collecting – Varijable i prikupljanje podataka
Topography – Topografija
Topography is one of the constant factors affecting fire behavior. There are differences in fuel characteristics depending on the aspect. Southern aspects of the northern hemisphere have more sunlight, higher temperatures, strong winds, and low humidity. Accordingly, the lower fuel humidity on south-facing slopes increases the fire risk by making the fuel more suitable for ignition. Elevation is one of the topographic factors affecting the fire in terms of weather conditions and changes in fuel characteristics. Slope is effective on the spread direction and spread rate of the fire (Attri et al. 2020). Slope, aspect, and elevation variables representing topographic features were derived from the digital elevation model (DEM) using ArcGIS 10.5. (Esri 2017).
Meteorological parameters – Meteorološki parametri
High temperatures not only cause fuel to dry but also cause fuel to need less heat to reach its ignition temperature. The highest temperatures and lowest levels of relative humidity occur at noon, and therefore, the fire risk and danger are highest at this time of day. Therefore, we used the monthly maximum temperature instead of the monthly average temperature as the independent variable. We downloaded the monthly maximum temperature maps from the open source website (URL-1) and then we cut these maps according to the study area using ArcGIS 10.5. (Esri 2017).
As it is known, precipitation increases the moisture content of fuel and therefore reduces the fire probability. We obtained monthly precipitation maps from the open-source website (URL-1) and extracted the necessary data to produce maps of monthly precipitation in the study region using ArcGIS 10.5 (Esri 2017). Wind highly affects the direction and spread of fire (Kucuk and Sağlam 2004). There is a very close relationship between wind speed and the rate of fire spread. As the wind speed increases, the rate of fire spread also increases. We used maximum temperature, precipitation, and wind speed variables representing meteorological parameters for modeling FFDP .
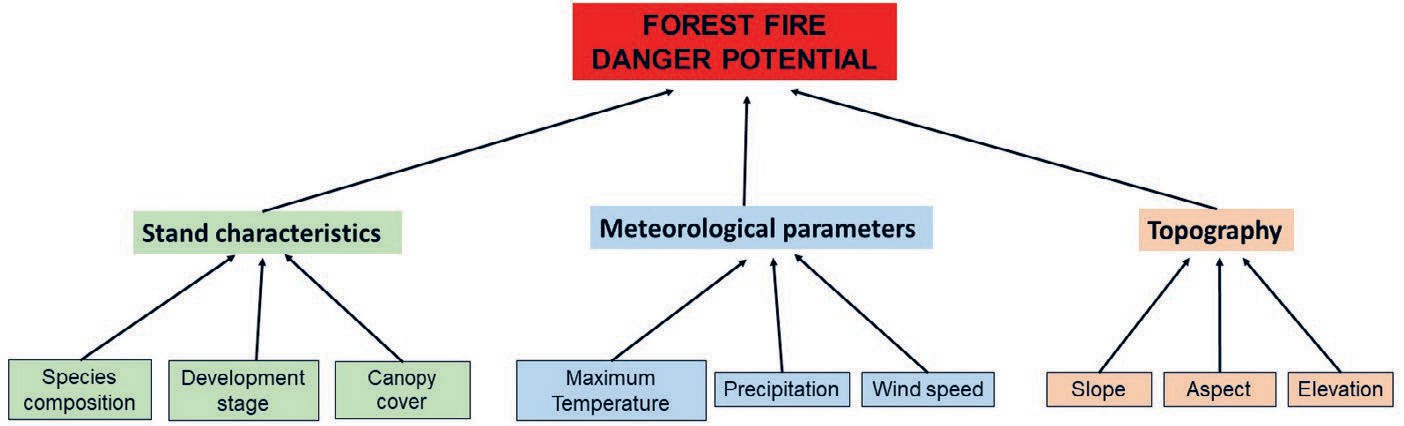
Figure 2. Hierarchy construction of forest fire danger potential (FFDP)
Slika 2. Hijerarhijska konstrukcija potencijala opasnosti od šumskog požara (POSP)
Stand characteristics – Karakteristike šumskih sastojina
Fuel is defined as organic material that can ignite and burn, living or dead, on the cover or top. Fuel characteristics such as the fuel type, its continuity, amount and size affect fire behavior (Kucuk et al. 2015). Fuel type affects the flammability of fuel, while fuel continuity affects fire intensity, direction, and the rate of spread. The amount of fuel also affects the burning rate (Küçük et al. 2005). In this study, species composition representing the fuel type, stand canopy cover representing the continuity and the amount of fuel, and the development stage representing fuel size were used as the explanatory variables. Stand maps in the forest management database obtained from GDF were reclassified according to tree species composition, development stage, and canopy cover, and stand characteristics variables representing fuel characteristics were prepared using the ArgGIS 10.5. Maps of stand characteristics prepared in vector format were converted to raster format.
Modeling of forest fire danger potential using AHP method – Modeliranje potencijala opasnosti od šumskog požara AHP metodom
Analytical Hierarchy Process (AHP) is a tool that can be used in various study topics to simplify and synthesize complexity. AHP is a multi-criteria decision-making method in which the relative weights of possible decision alternatives or outcomes are given a functional value based on a mathematical representation of pairwise comparisons (Saaty 1990). AHP includes five steps: hierarchy construction, pairwise comparisons, calculating weights, checking for consistency, and generating results (Saaty 2001).
To begin AHP, it is necessary to identify the parts of the decision that contribute to the overall outcome and construct the hierarchy. We have established a hierarchic order for FFDP in line with the classical AHP technique (Figure 2). In order to model the FFDP, the main criteria affecting the fire behavior are topography, meteorological parameters, and stand characteristics (forest fuel properties). Nine subcriteria related to the main criteria were determined, and a hierarchy was constructed (Figure 2).
To determine the importance of the main criteria and subcriteria using the AHP method, the opinions of 5 fire specialists were taken and each fire specialist presented her/his own opinion according to a pairwise comparison scale (Table 1). In order to obtain the values representing the group, a pairwise comparison matrix was formed by averaging the expert opinions.
Table 1. Pairwise comparison scale (Saaty, 1990)
Tablica 1. Skala matrica uspoređivanja (Saaty, 1990)
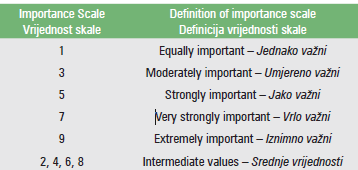
We calculated the normalized weight coefficients with a total value of 1 (Table 3). After that, we calculated the consistency ratios (CR) of the matrix to determine whether the experts’ answers were consistent. If the Consistency Ratio (CR) value is less than 0.1, the matrix is sufficiently consistent and can be used to calculate results. If the Consistency Ratio (CR) value is greater than 0.1, pairwise comparisons should be made again by experts to increase the consistency of the final decision.
Furthermore, the variable classes are given a fire danger level of extreme, high, medium, low, and very low according to the danger potential of each class (Jaiswal et al. 2002). Then each fire danger parameter in itself is created in ArcMap 10.5 (Esri 2017). Six maps were derived by grading from 1 to 5 by reclassification using the GIS program (Table 2). In the values assigned to the parameters, the value “1” indicates that the fire danger is very low, and the value of “5” means that the fire danger is extreme. FFDP maps were produced monthly using Equation 1.
To test the model accuracy, we compared the FFDP maps with fires in the period 2008–2018 (Figure 3, Table 4). After that, we did a monthly assessment for each of the fire danger classes (Table 4). We then looked at the distribution of fires that occurred between 2008 and 2018, by fire danger class, on a monthly basis.
Table 2. Classification of relative effects of criteria.
Tablica 2. Klasifikacija relativnih učinaka kriterija.
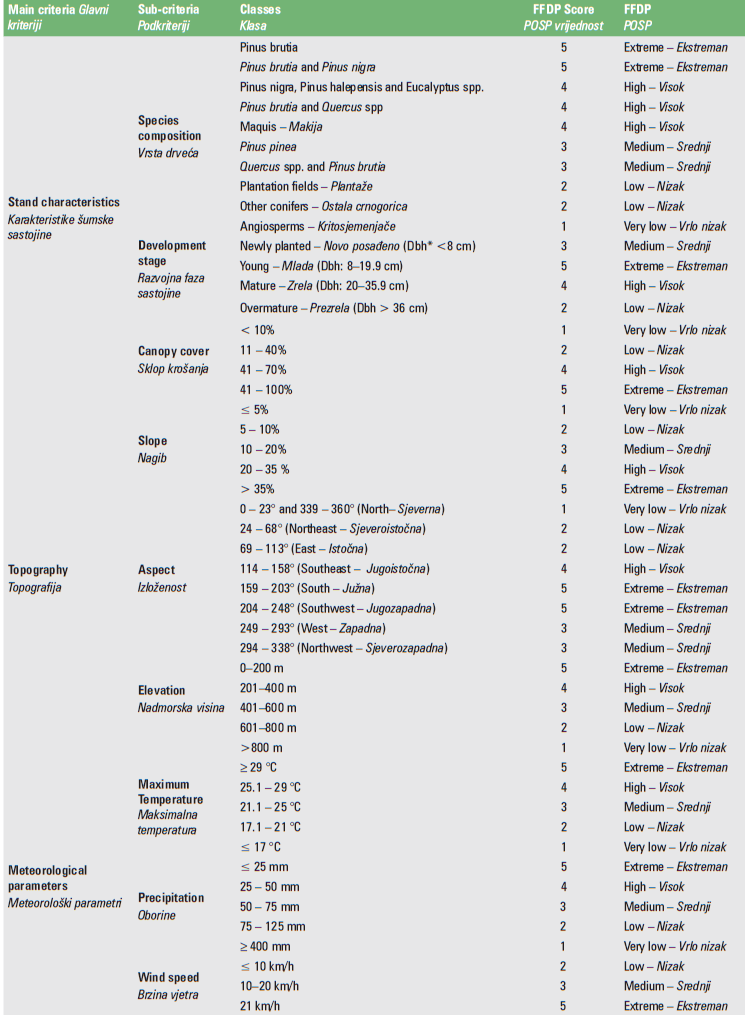
*Dbh = Diameter at breast height – Prsni promjer
REZULTATI I RASPRAVA
In this study, we assessed the forest fire danger potential monthly during the fire season by using the AHP method as the environmental variable (i.e., meteorological parameters, topography, stand characteristics). It is necessary to determine the importance of each criterion to estimate the weights in preparing fire danger potential maps using AHP. In this study, the AHP pairwise comparison matrix was created by taking expert opinions to determine the weights of the criteria and sub-criteria. Consistency ratios (CR) of meteorological parameters, stand characteristics, and topography criteria were calculated (Table 3). According to the findings, the CR value of all criteria and sub-criteria values is less than 0.1; that is, all pairwise comparison matrices have sufficient and acceptable consistency.
While meteorological parameters were the most important main criteria affecting the FFDP with a weight of 66%, they were followed by stand characteristics with a weight of 21%. Considering the sub-criteria, the four most important factors affecting the FFDP were maximum temperature (40%), precipitation (18%), species composition (%13) and wind speed (8%) (Table 3, Equitation 1).
FFDP = 0.4 × MaxTemp + 0.18 × P + 0.08 × WS +
0.13 × SC + 0.04 × CC + 0.04 ×DS + 0.04 ×S +
0.05 × A + 0.03 × E (Eq. 1)
Where:
FFDP = Forest fire danger potential MaxTemp = Maximum temperature P = Precipitation,
WS = Wind speed,
SC = Species composition,
CC = Canopy cover,
D = Development stage, S = Slope,
A = Aspect, and E = Elevation.
We mapped the monthly FFDP using Equation 1 by GIS. In the monthly FFDP representation on the map, we used red color for extreme fire danger, orange for high, yellow for medium, green for low, and blue for very low (Figure 3). The results showed that the fire danger potential changes significantly on a monthly basis. Forest lands in the study area of 2% in May, 50% in June, 65% in July, 61% in August, 25% in September, and 0% in October belong to the extreme danger class (Figure 3).
We performed the accuracy assessment of the model on a monthly basis. For model evaluation, we compared the fire locations from 2008 to 2018 with those on the FFDP maps and then compared the actual number of fires in each category and the fire danger class (Table 4). The results showed that the potential fire danger class of the study area was extreme (50%, 65%, and 61%, in June, July, and August, respectively. It was high in May and September (74% and 68%, respectively). In October, it was observed that the areas belonging to the medium (83%) danger class were in the majority and were more affected by the fire (Table 4). The
amount of burned area per fire has the highest value in the extreme danger class in August and July (3.39 ha and 2.14 ha, respectively) (Table 4). The amount of burned area was higher in areas with high and extreme fire danger classes (Table 4).
In a similar study, Saglam et al. (2008) evaluated FFDP on a spatial and temporal scale. According to the Fire Danger Potential results, 3,068 ha of the study area represented medium, 4,729 ha high, and 4,728 ha extreme danger class in 1987. By the year 2000, 597 ha of the study area representedlow, 208 ha medium, 4448 ha high, 6569 ha extreme danger class. In the study area, it was emphasized that together with fire extinguishing and afforestation activities, it caused the growth and development of the forest, the accumulation of vegetation and combustible material, more continuity in the vertical and horizontal stand structure, and thus contributed to the increase of the fire danger potential between 1987-2000. Although their results are similar to our study in terms of the influence of stand characteristics and topography on fire danger potential, meteorological parameters were also taken into account in addition to these variables in our study. The most important variable was meteorological parameters, followed by stand characteristics and topography factors, respectively.
Laneve et al. (2020) developed the Daily Dynamic Fire Danger Potential Index in Sardinia, Italy, using variables of wind speed, topography, and local solar radiation (aspect). The results showed that 17% of the study area was classified as very low, 39% as low, 26% as moderate, 13% as high, and 54% as representing extreme danger. 12% of the fires occurred in very low, 21% in low, 31% in medium, 13% in high, and 23% in extreme danger class areas. Fuel properties, topography, wind, and temperature variables used in estimating the fire danger potential are similar to those used in this study.
Sharples et al. (2009) combined meteorological information with fuel moisture content estimates to create a fire danger index. The meteorological variables used to estimate the fire danger potential are similar to this study. Unlike their study, topography and fuel characteristics were also used as variables in the modeling of fire danger potential, and it was revealed that especially fuel characteristics were among the main variables affecting fire danger.
Table 3. Normalized weights of main criteria and sub-criteria
Tablica 3. Normalizirani ponderi glavnih kriterija i podkriterija
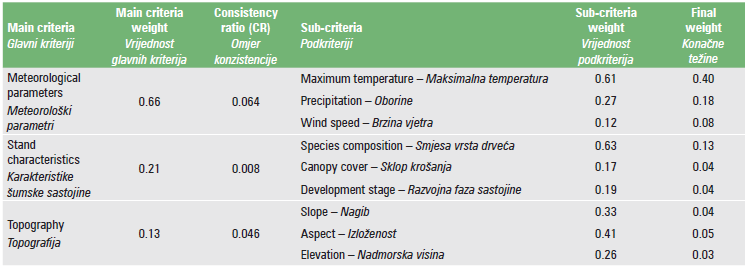
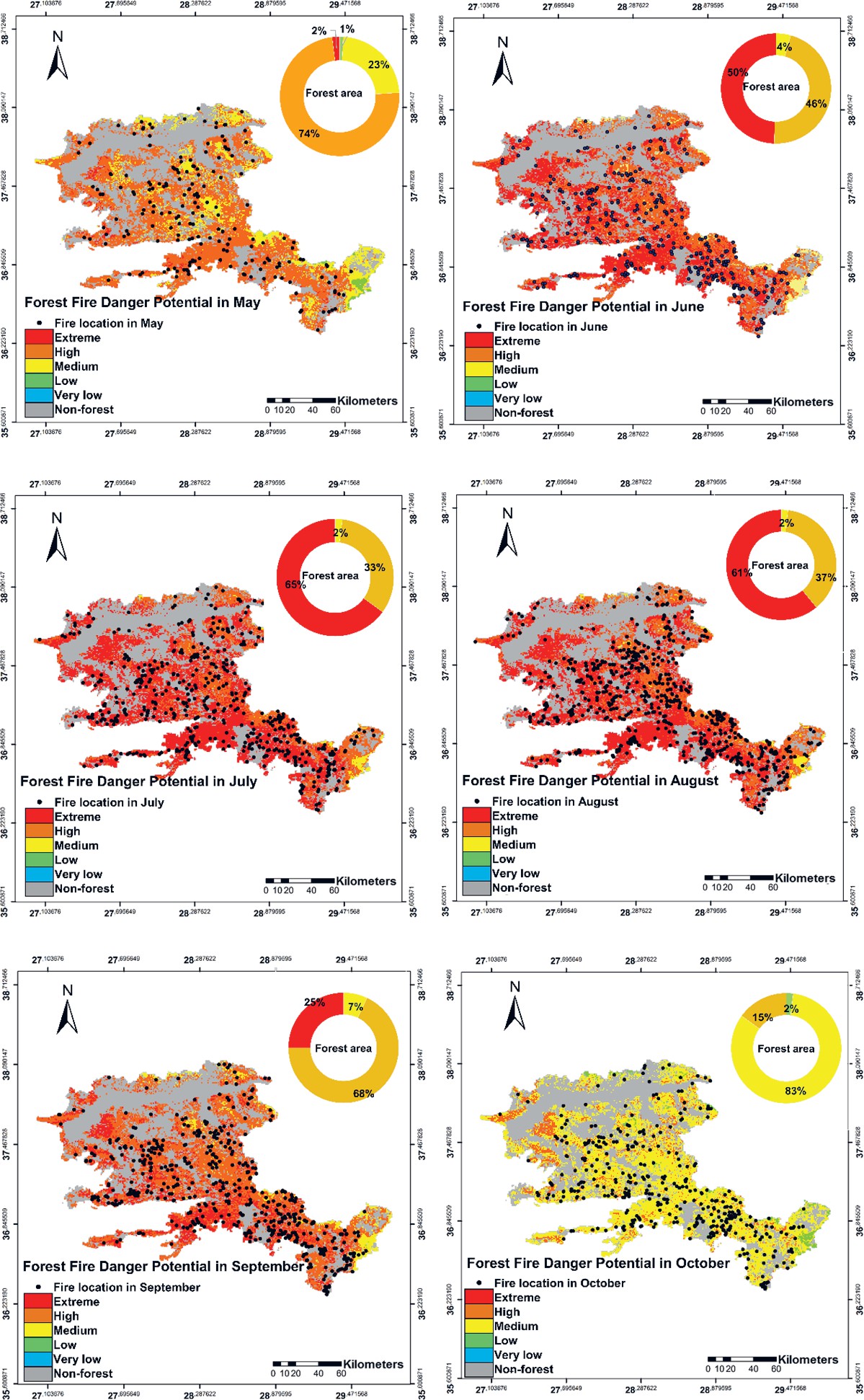
Figure 3. FFDP maps and fire location in the period 2008-2018.
Slika 3. FFDP karte i lokacija požara u razdoblju 2008.-2018.
Table 4. Potential fire danger categories of forest areas and monthly comparison of estimated FFDP maps and fires that occurred between 2008 and 2018
Tablica 4. Potencijalne kategorije ugroženosti šumskih površina od požara i mjesečna usporedba procijenjenih FFDP karata i požara koji su se dogodili u razdoblju od 2008. do 2018.
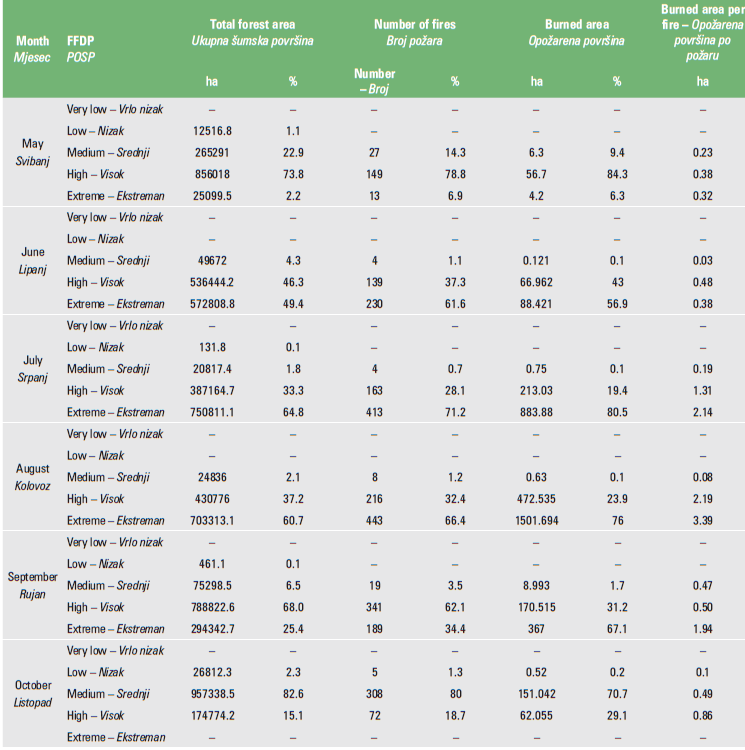
ZAKLJUČCI
In recent years, the forests in the Mediterranean basin, including Türkiye, have been seriously affected by forest fires. Despite the increasing fire prevention efforts of the fire department, the annual number of fires and the resulting damage is increasing significantly. It has become increasingly important to reveal the danger potential of a possible fire on a spatial and temporal scale. For this reason, modeling and mapping of FFDP can help fire organizations in pre-fire management planning, firefighting efforts, and post-fire studies. FDDP can also guide the application of silvicultural treatments. Modeling and mapping of the fire danger potential was conducted using the multi-criteria decision-making method (AHP) technique by fixed and variable environmental factors such as fast and easily accessible stand characteristics, topography, and meteorological parameters as variables.
The points where the FFDP model and maps can be used are given below:
FFDP can be predicted for a particular area based on fixed and changeable environmental factors.
FFDP maps may help to decide to warn the public against a forest fire and restrict human activity in the forest when the fire danger is high.
Considering FFDP can contribute to the spatial planning of forest roads and firebreaks as a preventive measure.
FFDP can contribute to determining areas with high fire danger potential and planning silvicultural treatments such as thinning and pruning in these areas.
The FFDP of a possible fire can be predicted, and thus, it will help to prepare an effective and successful fire suppression plan.
Considering FFDP can contribute to the temporal and spatial planning of controlled burning treatments.
FFDP maps that are updated in each plan period depending on the environmental factors and included in the forest management plan will be very useful to mitigate fire and damage by fire.