Methods
The assessment was carried out using estimated classifications by demographic indicators. The study was based on data on mortality rates published in “Regions of Russia” and “Demographic Yearbook of Russia” by the Federal State Statistics Service (Rosstat). A database of cities of the Russian Federation for 2011–2015 was created according to information provided by Rosstat upon request. The dataset included 250 territorial units: 85 regions (including cities of federal significance) and 165 large cities with populations of over 100,000 (Fig. 1). Data on cities were not excluded from regional statistics. This was done intentionally since the analysis was not carried out for all cities, but only for some of the largest, which would not have provided a clear picture of the urban/rural population.
Figure 1 Federal subjects of Russia / Slika 1. Savezne teritorijalne jedinice Rusije
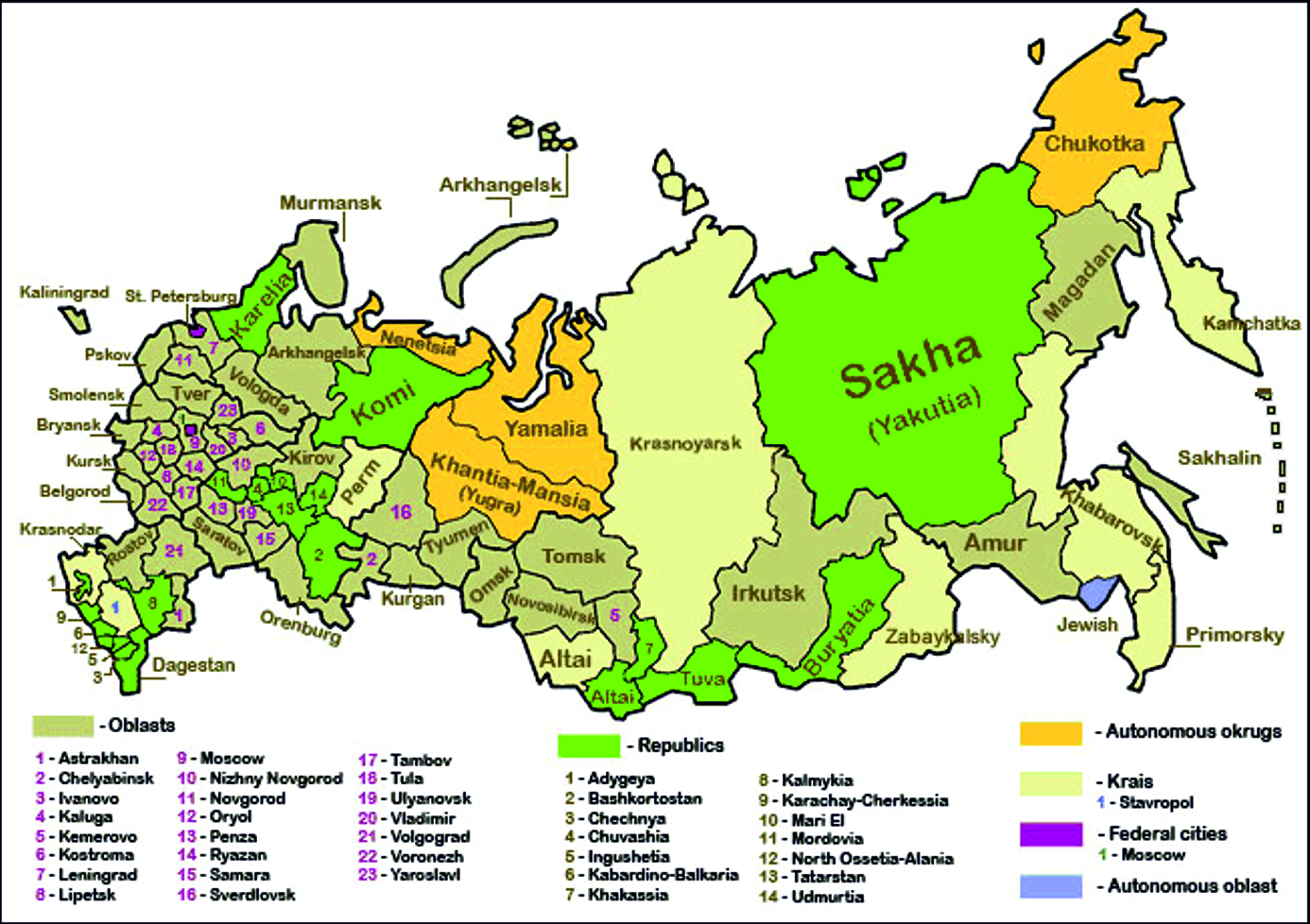
The selection of indicators for the database was carried out based on the tenth revision of the International Classification of Diseases (ICD-10). Altogether, 306 causes of death were analysed, including disease classes according to ICD-10 and individual nosological entities. Consequently, 140 causes of death were selected and included in the database. They were of prime importance in conducting a medical-geographical analysis of the health status of the urban population.
Based on the primary statistics, standardised mortality rates were calculated using the 1975 World Health Organization European standard. The direct standardisation method was used (Practical demography 2005,Denisenko et.al. 2009). To determine the derived indicators, an algorithm for the automated calculation of standardised medico-demographic indicators using MS VisualFoxPro 9.0 DBMS was developed. The programme developed allows selecting the desired combination (year, cause of death, age group, gender, etc.) and the subsequent output of MS Excel tables containing the age-specific mortality rate, standardised mortality rate, lower and upper boundaries of the confidence interval, and the length of the confidence interval.
Thus, processing the primary material allowed us to obtain data representing the age composition of the population in 5-year groups, mortality for reasons corresponding to the classes of diseases according to ICD-10, and several pathologies for men and women separately.
Based on the obtained indicators (6 types of causes of death: some infectious and parasitic diseases; tumours; diseases of the circulatory system; respiratory diseases; diseases of the digestive system; external causes of death) classification was carried out according to an algorithm developed by one of the authors (Author 1997).
In the applied typological algorithm, the entire set of parameters for any territorial unit (TU) is denoted by X={x1,…,xN}, where xi -th ТU, N the number of TU. The initial TUs are represented in the form of a TU-sign matrix, which reflects the measurement of indicators on a TU and contains rows and columns:
where
-i -th TU in M- dimensional space of parameters
- j -th parameter,
,
- the value of j -th parameter of i -th TU,
.
The next stage of TU classification is pre-treatment, including normalisation, weighing, and dimensionality reduction. Normalisation was carried out according to the variance and mathematical expectation. The purpose of this normalisation was to bring each indicator to a standard form (as a result, the mathematical expectation of any indicator becomes equal to zero, and the variance equal to one). Let:
-be the assessment of mathematical expectation of j -th parameter, and
- be the assessment of variance of j -th parameter.
Then normalisation means the recalculation
, i.e.
The next step includes the application of the principal component method. This method solves the problem of searching based on the existing system of attributes that describe a TU. The method of principal components should be used to correct the original feature space distorted by mutual correlations, reduce the amount of stored data without losing a significant part of information about the TU, visualize the TU in the feature space (for example, by displaying the TU in the form of points on a plane) and revealing hidden indicators, reflecting the essence of the process or phenomenon.
The simplest is the geometric interpretation of the principal component method. In a multidimensional parameter space, the TUs are considered as points whose cloud geometric arrangement, in the case of normal distribution, resembles an M-dimensional ellipsoid. The main axes of the imaginary ellipsoid are treated as the new parameters, sorted in the descending order of TU dispersion along the axes.
The most general relation is used to calculate the distance for M quantitative parameters:
Our classifications aimed to obtain homogeneous TU groups in M-dimensional attribute space, i.e. possible "types" of TU. For this, the largest distance was selected from the obtained Euclidean distances, and the two territorial units that it connected became the nuclei of homogeneous clusters. Clusters were formed by the distribution of the remaining territories between the two cores according to the minimum Euclidean distances. In the case of the allocation of a larger number of clusters, to determine the third core and all subsequent ones, each remaining territorial unit was substituted in the form of a core, and the rest distributed between the three cores according to the minimum distance. The procedure for determining the 4th core and the formation of a typology with 4 types was similar to that described above, to the maximum possible value specified in advance tmax, and similarly with the minimum value tmin. The resulting number of groupings can be analysed by the absolute and relative heterogeneity coefficients and, thus, we can choose the optimal number of clusters:
,
,
where k is the number of identified groups, p is the number of the orthogonalised coefficients to calculate distances, tmin,tmaxtmin,tmax are the maximum and the minimum number of groups, Iik,Ijk is the indicator (binary), pointing to the presence (1) or absence (0) of TUs in group k.
A sharp increase in Ak or Ok with a decrease in the number of identifiable clusters, indicates the increase in heterogeneity within the identified clusters, while a smooth increase in the coefficients is a sign of uniform increase. The threshold followed by a sharp increase in heterogeneity can be taken optimally as the final number of clusters. In our analysis, the isolation of five clusters in all calculations turned out to be optimal (Author 1997).
As a result of the classification of cities and regions of Russia, five large groups (macro types) were identified for men and for women. At the same time, two macro types can be characterised as extremes – they included a very small number of regions (often only one region). The remaining macro types were too large for detailed analysis. Therefore, for the other three macro types, the classification algorithm was repeated separately for each, to distinguish subtypes.
Results
As a result of the two-stage classification of the causes of mortality in large cities and regions of Russia, 5 macro types were obtained for men and women. For men, 12 subtypes were distinguished, and 10 subtypes for women (Figs. 2 and (4). Based on the results obtained, maps were prepared (Figs. 3 and (5). Maps legends were made in different, but adjacent colours, to emphasise that the types for men and women were not the same, but that there were some general trends. For men, more shades of red were used to show that their level was worse than that of women. The size of the charts corresponded to the total mortality in the subgroups, for men and women on the same scale, which allowed them to be compared visually. Let us examine the situation among men and women separately in more detail.
Figure 2 Typology by classes of causes of death and average values of types, for males 2015 / Slika 2. Tipologija po klasama uzroka smrti i prosječnim vrijednostima vrsta, za muškarce 2015.
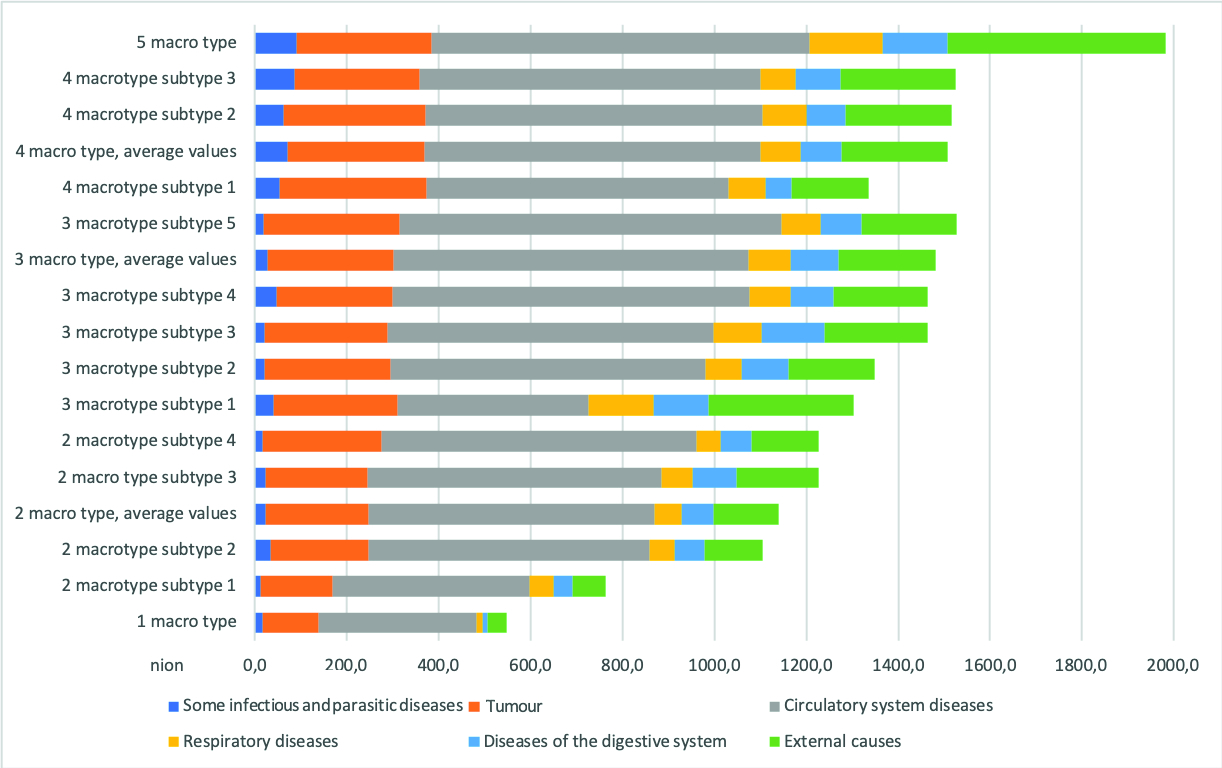
Figure 3 Typology by classes of causes of death, for males 2015 / Slika 3. Tipologija po razredima uzroka smrti za muškarce 2015.
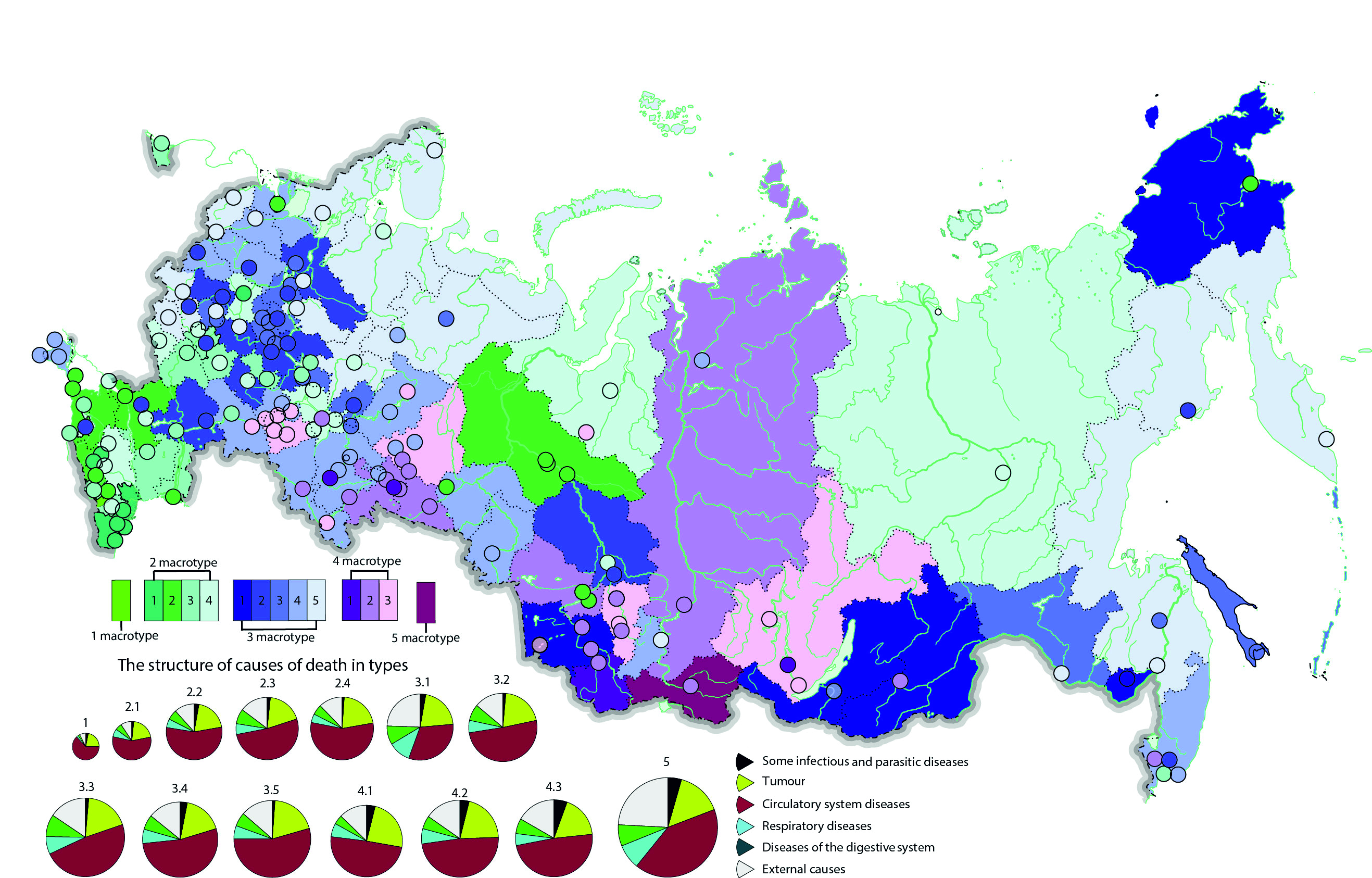
As mentioned above, two macrotypes of male mortality can be attributed to extremes. Macrotype No1 is characterised by minimum mortality values for all analysed indicators and includes only one region, the Republic of Ingushetia. Macrotype No5, on the other hand, is a representative of the maximum mortality values for all indicators. It also includes only one region, the Republic of Tuva. This may be due to the actual situation in the regions and the peculiarities of collecting statistical material. An explanation of this issue requires a more detailed local study of these regions at the municipal district level.
Macrotype No2is generally low in all-male mortality indicators. Most regions of this macro type are in the central part of European Russia. They include primarily Moscow and the Moscow region, the Republic of Mordovia and Tatarstan. Outside European Russia, only the Yamal-Nenets, Khanty-Mansi Autonomous Okrug, and Republic of Yakutia belong to this macrotype. It is interesting to trace the internal heterogeneity of mortality rates in this macro type. According to the classification algorithm used, four subtypes can be distinguished, which combine 94 territorial units.
The first subtype is characterised by the lowest rates within the group for all the considered causes of death and includes the Republic of Dagestan with the cities of Derbent, Kaspiysk, Makhachkala, Khasavyurt, the cities of the Stavropol Territory (Essentuki and Kislovodsk) and the city of Moscow. The remaining three types, against the background of low mortality rates in general, are distinguished by slightly higher values for some reason.
The second subtype is specific for increased mortality from infectious diseases. Regions of this type are located mainly in the North Caucasus (Krasnodar Territory, Kabardino-Balkarian, Karachay-Cherkess, and Chechen Republics, Rostov Region), while the Khanty-Mansiysk District with all its major cities and the city of Anadyr belong to the same type.
The third subtype of macro type No2 is characterized by increased mortality from diseases of the respiratory system, digestive system, and external causes. It includes the Astrakhan region, the Republic of Kalmykia, Lipetsk, Tambov regions, and the Republic of Mordovia, as well as the Kaliningrad region. It should be noted that this group includes only one city in the Russian Far East – Vladivostok.
Despite a significant decrease in mortality from external causes over the past decade, this category remains one of the top causes of death in Russia. In comparison to other developed and emerging countries, Russia has a substantially higher level of external causes of death (Rybakovsky et al. 2017). In particular, this problem concerns premature deaths among the working age population. At the same time, there is a convergence between regions in terms of mortality from external causes, while the interregional divergence of trends is mainly determined by cardiovascular diseases (Timonin et al. 2017).
The mortality rates from homicide and suicide have shown a steady decline since the 2000s. Another group of causes of death, injuries of undetermined intent, is growing rapidly and since 2014 has exceeded the total mortality rate from homicides and suicides (Yumaguzin and Vinnik 2019).Repetskaya (2019) has shown that recorded homicides account for only 20% of actual homicides. As a result, the real data on homicides and suicides is underestimated. Mortality from ill-defined conditions in Russia also has the fastest rate of increase compared to all other major causes of death. Insufficient investigation of the circumstances of the deaths of socially isolated people contributes to trends observed in mortality from ill-defined conditions (Gavrilova et al. 2008). This can lead to the underestimation of mortality from external causes.
Traditionally, alcohol consumption is considered a risk factor for increased mortality from external causes in Russia (Brainerd and Cutler 2005). For example, Russia had more than ten times the rate of male alcohol-attributable premature deaths compared with Sweden (Rehm et al. 2007). Despite the decline in alcohol-related mortality, alcohol is still the leading cause of the high risk of premature death among Russian adults (Zaridze et al. 2014). This risk factor is characterised by significant fluctuations over time. For example, the strongest negative correlation between changes in life expectancy and alcohol poisoning was found between 1984 and 2003. From 2003 to 2017, a consistent positive life expectancy trend was statistically independent of alcohol poisoning (Danilova et al. 2020). Hot spots of alcohol-related mortality mainly located in the territories of north-western and western Russia (Grigoriev et al. 2020). Despite the positive trends, these changes have not yet been sufficient to change radically the situation regarding external causes of death.
The fourth subtype is characterised by increased mortality from tumours and diseases of the circulatory system. It includes the Stavropol Territory with the cities of Stavropol and Pyatigorsk, and the Moscow Region with the cities nearest to the capital – Korolev, Mytishchi, Odintsovo, Podolsk and Ramenskoye. Of the remaining regions of the Russian Federation, only five regions belong to this subtype: the Republic of Adygea and the Belgorod region (with Belgorod and Stary Oskol), the Republic of Tatarstan (with Kazan, Naberezhnye Chelny, Nizhnekamsk), the Yamalo-Nenets Autonomous Okrug, and the Republic of Sakha. In addition, some cities in the southern regions are attributed to this type: Grozny, Volgodonsk and Taganrog. The only northern city of this type is Severodvinsk in the Arkhangelsk region, and the most eastern cities are Novy Urengoy, Yakutsk and Seversk in Tomsk region.
The macrotypes No3 and No4are generally close in terms of their average mortality rates, which is quite high. However, macrotype No4 is sharply distinguished by a higher mortality rate from infectious diseases (if we exclude the values of macrotype No5, these are the maximum mortality values for the entire sample). This is most clearly demonstrated in the third subtype, which includes three regions of the Urals and Siberia: Sverdlovsk, Irkutsk region (including the cities of Irkutsk and Bratsk) and Kemerovo region (including the city of Prokopyevsk), as well as one part of the Volga region – Samara (including Samara, Novokuybyshevsk, Syzran and Tolyatti). This corresponds quite well to the distribution of mortality from tuberculosis, one of the main causes of death from infectious diseases in the territory of the Russian Federation. The first subtype of macro type No4 is distinguished by the highest mortality rates from tumours among all the selected groups. Only the Altai Territory and the cities of Chelyabinsk, Salavat (Republic of Bashkiria), Noyabrsk (Yamalo-Nenets Autonomous Area), and Angarsk (Irkutsk Region) belong to it. This macro type includes other Ural (Chelyabinsk and Kurgan regions) and Siberian (Novosibirsk region and Krasnoyarsk Territory) regions. In general, this is a fairly small macro type in composition, uniting only 43 analysed administrative units, predominantly the regions and cities of the Urals and Siberia.
Macrotype No3 is the most differentiated: in total, five subtypes were distinguished. Of greatest interest is its first subtype, characterised by high mortality from respiratory diseases and external causes. It includes the Jewish Autonomous Region with Birobidzhan, Trans-Baikal Territory, the Altai Republic, Buryatia, and the Chukotka Autonomous Region.
Next, the fifth subtype should be distinguished, characterised by high mortality from diseases of the circulatory system and uniting the regions and cities of the European North (Arkhangelsk, Kostroma, Murmansk, Pskov regions, Nenets Autonomous Okrug, the Republic of Karelia and Komi) and the Russian Far East (Kamchatka, Khabarovsk Territory and the Magadan region).
Finally, the third subtype is characterised by high rates of mortality from diseases of the digestive system, also uniting the regions and cities of European Russia (Yaroslavl, Vladimir, Ivanovo, Tula regions, the Republic of Mari El, Udmurtia and Chuvash) and the Russian Far East (Amur and Sakhalin regions).
In general, it can be noted that macrotype No3 includes regions characterised by the most unfavourable situation in terms of mortality for almost all the reasons analysed, except mortality from infectious diseases. This is the most prevalent macro type and covers 116 analysed cities and regions.
It is interesting to note that macro types No2 and No4 are mainly characterised by the coincidence of the current mortality situation in the region and its cities. However, there are exceptions. So, the Moscow region is distinguished by significant spatial differentiation.
The difference in mortality between cities and regions is more noticeable in macro type No3. A striking example is Primorsky Krai, where the nearby cities of Artem, Ussuriysk, Nakhodka, and Vladivostok belong to completely different types of mortality.
Next, we consider the situation with mortality among women. Here we can distinguish only one macro type related to extrema. This is macrotype No5, where extremely high mortality rates from all the causes considered are recorded. Again, this macro type includes only one region – the Republic of Tuva.
Figure 4 Typology by classes of causes of death and average values of types, for females 2015 / Slika 4. Tipologija po klasama uzroka smrti i prosječnim vrijednostima vrsta, za žene 2015.
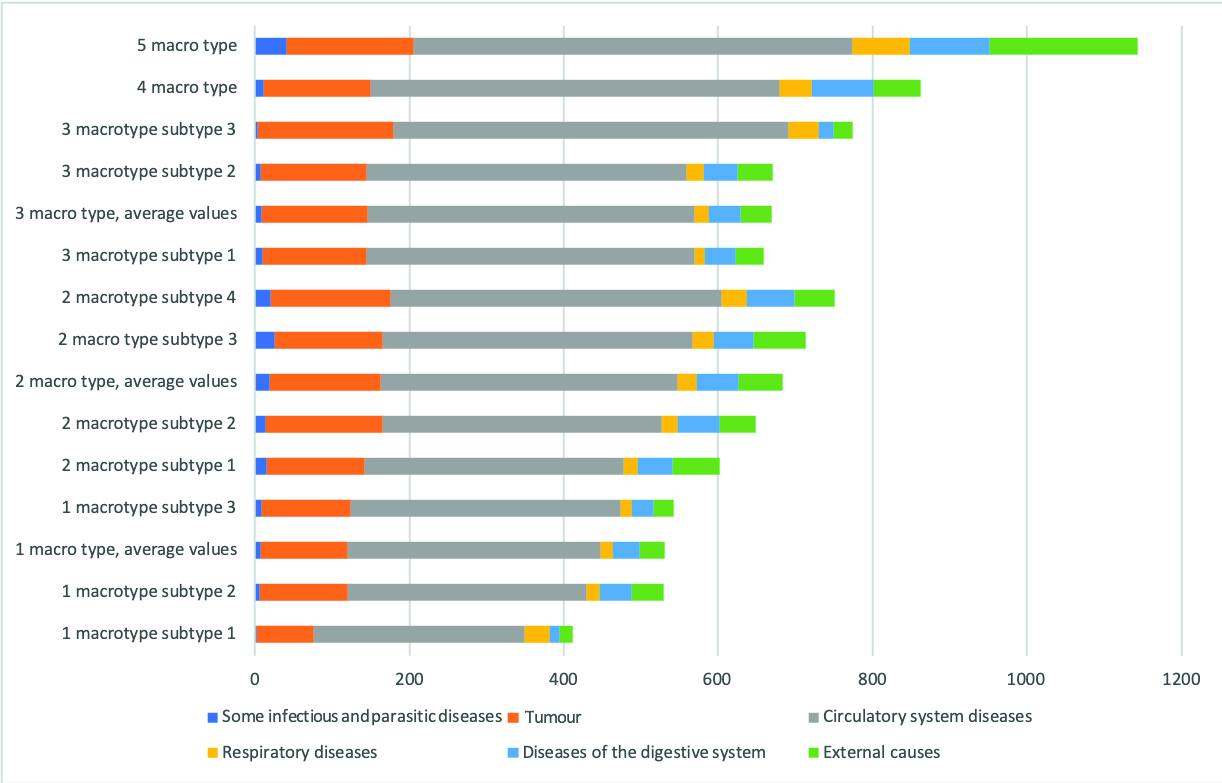
Figure 5 Typology by classes of causes of death, for females 2015 / Slika 5. Tipologija po razredima uzroka smrti, za žene 2015.
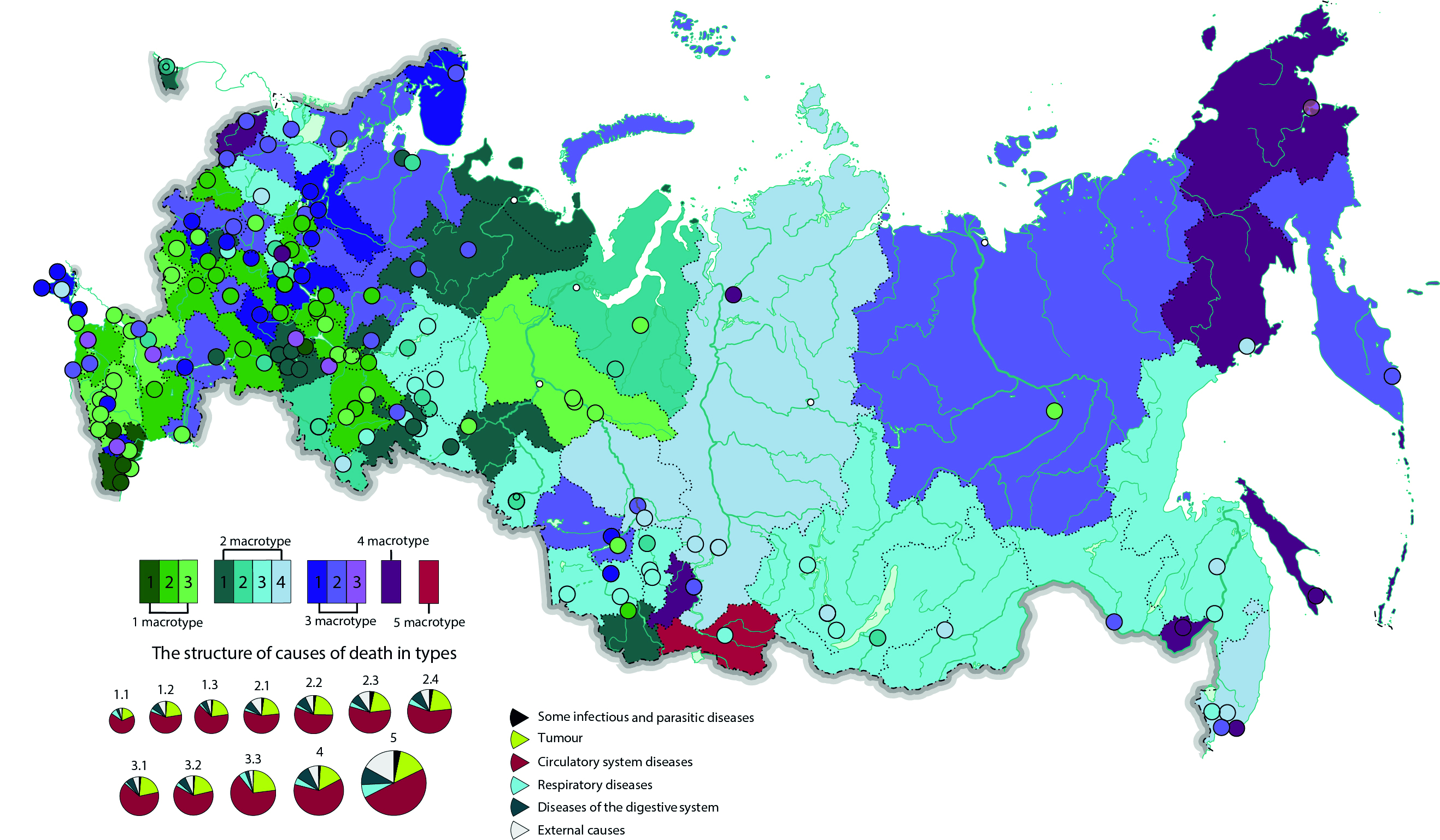
Macrotype No1 unites the regions and cities with the lowest female mortality for all reasons. The first subtype of this macrotype is noteworthy, because it is characterised by minimal mortality rates for all reasons and increased mortality from respiratory diseases. This type includes only the Republic of Dagestan with the cities of Makhachkala and Derbent, and the city of Nazran (Republic of Ingushetia).
The second and third subtypes of macro type 1 form two equal groups according to the number of territorial objects included (42 and 41, respectively). The second subtype is characterised by slightly higher mortality rates from diseases of the digestive system and external causes. It includes only cities and regions of the European part, stretching from the Republic of Kalmykia and the Krasnodar Territory to the Smolensk and Yaroslavl Regions in the north and the Republic of Bashkortostan in the east. In general, macrotype No1 is characterised by territorial confinement to the European part of the Russian Federation. Beyond it, low mortality rates are observed only in the regions of oil and gas production and in some large cities.
The third subtype, besides the background of low mortality rates, is characterised by a predominance of mortality from tumours and diseases of the circulatory system. These are the republics of the North Caucasus - Ingushetia, Kabardino-Balkar, Karachay-Cherkess, North Ossetia and Adygea, and the Stavropol Territory and Rostov Region. The Belgorod region with the city of Belgorod, the region that should probably be recognised as the most prominent in terms of mortality among women among the European regions of the Russian Federation, as well as the oil and gas production region, the Khanty-Mansi Autonomous Okrug, with all its major cities and the city of Tyumen, belong to the same type. Many cities in the European part of the Russian Federation belong to this type; not only the cities of the North Caucasus but also the southern European part (Astrakhan and Taganrog), the Volga region (Kazan, NaberezhnyeChelny and Nizhnekamsk), and Moscow with adjacent Balashikha and Korolev. It should be noted that some cities have severe climatic conditions - NovyUrengoy and Yakutsk.
Macro types No2 and No3 combine territorial groups with predominantly high mortality rates. Moreover, they are very close to each other in terms of mortality from tumours, diseases of the circulatory system, and respiratory diseases, but differ from each other in mortality from infectious diseases, digestive diseases, and external causes. Macrotype No3 is distinguished by rather low mortality from infectious diseases and lower mortality from diseases of the digestive system and external causes than macrotype No2.
The first subtype of macrotype No3 is characterized by low mortality rates from respiratory diseases against the background of rather high mortality rates from tumours and diseases of the circulatory system and includes 31 subjects. These are the regions within the European part of the Russian Federation - Vologda, Murmansk, Nizhny Novgorod, Oryol, Ulyanovsk Regions, and the Republics of Chechen and Crimea. Among the cities belonging to this type outside European Russia, Barnaul and Novosibirsk should be noted.
The second subtype includes a slightly larger number of subjects (38) characterised by a rather high mortality rate from diseases of the digestive system and external causes. These are not only European regions, but also the territories of Siberia and the Russian Far East – Novosibirsk Region, the Republic of Yakutia, Kamchatka Territory, and Petropavlovsk-Kamchatsky. St. Petersburg and many cities in the Moscow region (Domodedovo, Kolomna, Mytishchi, Noginsk and Odintsovo) and the Russian Far East (Blagoveshchensk and Vladivostok) should be noted.
The third subtype of macro type No3 includes only three cities – Grozny, Krasnodar, and Anadyr. In terms of mortality from tumours, circulatory system diseases, and respiratory diseases, this subtype is close to macro type No4, characterised by high mortality rates from all causes. However, lower mortality rates from infectious diseases, diseases of the digestive system, and external causes do not allow it to be attributed to this macro type.
In macrotype No2, the fourth subtype (high mortality rates from tumours, circulatory system diseases, respiratory and digestive organs) includes four regions that are not geographically related to each other (Vladimir, Tomsk Regions, Krasnoyarsk and Primorsky Territories) and 15 cities. These are many industrial cities (Achinsk, Angarsk and Krasnoyarsk), cities with severe climatic conditions (Magadan and Chita), and also the cities of the Moscow region (Orekhovo-Zuevo, Pushkino, Sergiev-Posad and Schelkovo), as well as Simferopol (Republic of Crimea). This type, along with macrotype No4, can be described as the most unfavourable in terms of mortality among women.
It is interesting to note that the third subtype, macro type No2, is characterised by a high mortality rate from infectious diseases and external causes. It is confined mainly to the territory of Siberia (Trans-Baikal Territory, Irkutsk (including Irkutsk, Bratsk), Kemerovo (including Novokuznetsk, Prokopyevsk), Omsk Region, Altai Republic and Buryatia), the Urals (Kurgan, Sverdlovsk Region (including Nizhny Tagil, Pervouralsk), and the cities of the Chelyabinsk region – Kopeisk, Magnitogorsk, Miass) and the Far East (Amur Region, the cities of Ussuriysk and Khabarovsk), but territories belonging to this type are also found in the European part of the country – the Leningrad, Tver, and Perm regions.
Macrotype No4 is characterized by high mortality rates for all analysed parameters (especially diseases of the circulatory system, respiratory system, and digestive system); the exception is mortality from infectious diseases. First, the Russian Far Eastern regions and cities are distinguished – Chukotka Autonomous Okrug, Magadan Oblast, Sakhalin Oblast, including Yuzhno-Sakhalinsk, Jewish Autonomous Oblast, including Birobidzhan, and also the city of Nakhodka, Primorsky Krai. In the Siberian regions, the Republic of Khakassia and the city of Norilsk should be attributed to the same macrotype, while in the European ones, only the Pskov region is included.
As among men, female mortality in most cities and regions of the Russian Federation is characterised by high rates. Territories with low mortality are traditionally primarily the regions of the North Caucasus. However, in the case of female mortality, this rule is not always applicable.
Unlike many high-income countries, Russia has yet to make an epidemiological transition and control mortality from cardiovascular diseases. Ischemic heart disease and cerebrovascular diseases are still the leading causes of death in all regions of Russia.
As for cancer, positive trends are associated with a decrease in mortality from lung cancer. This may be due to both a change in lifestyle (the reduction in the number of people using tobacco) and a change in the quality of tobacco products (the reduction of tar in cigarettes). The temporal patterns of breast cancer mortality in Russia are in line with other countries in Europe, although cervical cancer rates and the rate of occurrence in recent generations are rapidly increasing (Barchuk et al. 2018). The association between suicide and cancer presents a significant problem and can be considered as an indicator of social disintegration. There is a positive relationship between trends in suicides and all types of cancer in men and women, apart from cancer of the small intestine and prostate cancer in men (Razvodovsky and Zotov 2016). The real occurrence of suicide in cancer patients is considered to be under-reported (Razvodovsky 2017).
Regional differences in causes of death can be influenced by lifestyles that are difficult to consider at the national level due to significant cultural differences and income levels. Regional practices for coding causes of death can also significantly distort the structure of mortality.