INTRODUCTION
In bone biology research, analyzing gene expression patterns is a common technique for understanding the mechanisms and signaling pathways involved in bone formation and remodeling (1–4). Quantitative reverse transcription polymerase chain reaction (RT‐qPCR) stands as the predominant method for this, particularly when evaluating a limited number of genes (5). This tool offers high sensitivity, reproducibility, and repeatability (1). However, the outcomes of RT‐qPCR can be influenced by various factors such as the starting quantity of mRNA, its purity and integrity, the reverse transcription yield, and the amplification efficiency (6, 7). Consequently, the incorporation of internal control becomes imperative. For normalization, several strategies can be used, with the most frequent being normalization against an endogenous reference gene exhibiting stable expression across all specific tissue samples (8, 9).
The choice of an appropriate reference gene for normalization in gene expression studies is crucial to ensure accurate and reliable results. Ideally, a reference gene should exhibit consistent expression levels in the specific tissues and cells, while being minimally influenced by the experimental conditions. While many studies conventionally employ a single reference gene for normalization, certain widely used reference genes in rat tissue expression analyses, such as beta-actin ( ACTB), glyceraldehyde-3-phosphate dehydrogenase ( GAPDH), 18S rRNA ( RRN18S), hypoxanthine-guanine phosphoribosyltransferase ( HPRT1), and beta-2-microglobulin ( B2M), have demonstrated inconsistent and unstable expression patterns across different experimental conditions (5, 8). It is therefore strongly recommended that the selection of reference genes be carried out on an individual basis for each research group, considering the specific tissues, treatment regimens, and experimental conditions (8, 10–12).
Several conventionally utilized reference genes may not be optimal for normalizing gene expression concerning bone markers during bone cell differentiation (12–14). Limited data exists on the selection of reference genes specific to rat alveolar bone tissue. According to Kirschneck et al., the most stable combinations of reference genes for combined dental, periodontal, and alveolar bone in rats include peptidylprolyl isomerase B ( PPIB) and tyrosine 3/tryptophan 5-monooxygenase activation protein, zeta polypeptide ( YWHAZ) genes in untreated rats, and PPIB and B2M in orthodontically forced rats (15). For developing rat long bones, succinate dehydrogenase (SDHA) and TATAA-box binding protein (TBP) emerged as the most stable during the prenatal phase, while postnatally the highest stability was observed with YWHAZ and GAPDH (16).
Many investigations have probed gene expression influenced by olanzapine across various tissues and species; however, the majority lack details on their reference gene selection methodology (17–19). Predominantly, studies focusing on olanzapine-induced gene expression in rats were conducted on brain tissue and often employed a single reference gene without prior validation of its suitability. The genes ACTB and GAPDH were frequently chosen in these studies (20–23).
To date, no research has meticulously assessed the stability of reference gene expression in olanzapine-treated rat bone tissues, and no universally accepted reference gene or gene combination has been identified. While olanzapine is known to influence bone tissue (24), its precise mechanism remains elusive. Some studies have associated olanzapine with induced bone resorption and bone loss (25–27), while others have reported its role in increasing bone density (28, 29). Given the potential multifaceted impact of olanzapine on bone, multiple signaling pathways may be involved. The influence of olanzapine on the Wnt/β-catenin signaling pathway, acknowledged as pivotal in bone turnover, emerges as a potential candidate for investigation (30).
The primary objective of this study focused on examining the expression stability of 12 candidate reference genes. This is geared towards identifying robust internal control genes for analyzing olanzapine-induced bone remodeling in rat alveolar and femoral bones. To achieve this, we employed the comparative delta Ct method, geNorm, NormFinder, and BestKeeper algorithms, and a comprehensive ranking system to assess the stability of these candidate genes.
EXPERIMENTAL
Animal model and bone collection
We undertook an experiment involving 12 male Wistar rats, aged between 16 and 18 weeks at the start of the study. Animal treatment and care adhered to previously established methodologies (31, 32). All the rats were maintained under standardized housing conditions with a regulated temperature (23–25 °C) and humidity, and a consistent 12-hour circadian cycle. Their diet consisted of the Teklad Global Rodent Diet 2016 (Harlan Laboratories, The Netherlands), complemented by unrestricted access to water.
Six of these rats were administered a daily dose of 2 mg kg–1 olanzapine (Krka d.d, Slovenia) p.o. over an 8-week period. The remaining rats, serving as controls, were given a placebo (saline solution) for the same time duration. After the 8-week period, all the animals were euthanized. The maxilla of each rat was cut in half, retaining the left segment inclusive of all teeth. All the soft tissue components, such as mucosa, musculature, and ligatures, were removed, ensuring only the osseous and dental tissues remained. From the femur, the distal third was sectioned, capturing the epiphysis, metaphysis, and a fragment of the diaphysis – a region renowned for heightened bone turnover under physiological parameters. Similarly, the non-osseous tissues, inclusive of musculature, ligatures, ligaments, and bone marrow within the medullary cavity, were removed from the femur. Subsequently, all the specimens were promptly preserved in liquid nitrogen.
Precautions were taken to prevent RNA degradation, which was critical to maintaining the integrity of the samples. Sterilized instruments were utilized throughout the sample collection phase. Additionally, RNaseZap spray (Ambion, USA) was applied to all surfaces and tools in direct contact with the tissue samples. The excision of the soft tissues from the samples was conducted on chilled glass plates to further preserve the sample quality.
All the procedures involving animals and the overarching study protocol were subject to review, and received approval from the “Ethics Committee for Animal Experiments of the Administration of the Republic of Slovenia for Food Safety, the Veterinary Sector, and Plant Protection” (Approval No. 34401-62/2008/20). All the methodologies and care protocols strictly adhered to the guiding principles outlined in “The Care and Use of Animals”.
RNA extraction and cDNA synthesis
The frozen samples underwent mechanical pulverization in liquid nitrogen using a mortar and pestle. The extraction of RNA adhered to previously published methodologies (33). Once optimal granularity was achieved, an aliquot of each powdered specimen weighing 120 ± 30 mg was collected in a 1.5-mL microcentrifuge tube infused with 1 mL of Trizol (TRIzol Plus RNA Purification System, Life Technologies, USA) to inhibit RNA degradation. This mixture was subsequently subjected to ultrasonic homogenization to diminish sample viscosity, thereby augmenting cellular membrane lysis and facilitating enhanced RNA release from the cells. RNA extraction ensued, utilizing the PureLink RNA Mini Kit (Invitrogen, USA) in alignment with the prescribed manufacturer’s protocols. The RNA concentration and purity metrics were determined spectrophotometrically by a NanoDrop ND-1000 (Thermo Fischer Scientific, USA). Meanwhile, the structural integrity of the RNA was assessed utilizing the Agilent RNA 6000 Nano Kit (Agilent Technologies, USA) alongside the Bioanalyzer 2100 (Agilent Technologies). The RNA extracted from the bone samples of all 12 animals demonstrated RNA integrity number (RIN) values above 7 and was deemed suitable for subsequent complementary DNA (cDNA) synthesis.
For cDNA synthesis, reverse transcription was carried out in a PeqSTAR Thermal Cycler (Peqlab Biotechnologie, Germany) using the High-Capacity cDNA Reverse Transcription Kit (Applied Biosystems, USA), adhering to the manufacturer’s guidelines. Each transcription reaction incorporated 750 ng of RNA. The reaction parameters were as follows: an initial phase at 25 °C for 10 minutes, 37 °C for 120 minutes, and 85 °C for 5 minutes. Post-transcription, cDNA samples were preserved at –20 °C pending subsequent analyses.
Quantitative RT-PCR assay
A quantitative RT-PCR assay was used to evaluate the stability of the candidate reference genes across distinct sample cohorts, specifically the maxillary and femur bone tissues extracted from the rats given either a placebo or olanzapine. The assay utilized the TATAA Reference Gene Panel (A103P, TATAA Biocenter AB, Sweden) in conjunction with the HOT FIREPol Probe qPCR Mix Plus (Solis BioDyne, Estonia). This panel encompasses assays for 12 reference genes: ACTB, B2M, GAPDH, beta-glucuronidase ( GUSB), HPRT1, phosphoglycerate kinase 1 ( PGK1), peptidylprolyl isomerase A (cyclophilin A, PPIA), 60S acidic ribosomal protein P0 ( RPLP), RRN18S, TBP, tubulin beta 5 class I ( TUBB5), and YWHAZ.
All the reactions were carried out on the LightCycler 480 platform (Roche Diagnostics Ltd., Switzerland). Each reaction, with a total volume of 20 µL, adhered to the manufacturer's directives. The amplification parameters were defined as follows: an initial denaturation at 95 °C for 12 minutes, succeeded by 40 amplification cycles comprising 15 seconds at 95 °C and 45 seconds at 60 °C, and concluding with a final elongation phase at 40 °C for 30 minutes. Both standards and sample assays were made in duplicate to ensure consistency.
To maintain the integrity and accuracy of the assay, several controls were used. A positive control evaluated the polymerase chain reaction efficiency; a no-template control (NTC) confirmed the absence of reagent contamination; and a no reverse transcriptase control (NRT) assessed gDNA contamination in the RNA samples.
Analysis of reference gene stability
For data analysis, we used GeNorm and NormFinder algorithms, the comparative delta Ct method, and BestKeeper software.
GeNorm: This algorithm determines gene stability based on the M value, which measures the average pairwise variation of a reference gene against all other reference genes. Genes with an M value below 1.5 are considered stable, with those having the lowest M values exhibiting the most stable expression. Additionally, GeNorm determines the optimal number of reference genes required for normalization by calculating the pairwise variation values (V) between normalization factors after stepwise inclusion of the reference genes, based on their M-values. The inclusion of each additional reference gene can result in a decrease or increase in the V-value. A cut-off for the V-value was set at 0.15, below which the inclusion of additional reference genes is not required for normalization (6).
NormFinder: This algorithm utilizes a mathematical model to evaluate both intra- and intergroup variability in reference gene expression. Combining these factors produces a candidate gene stability value. The model further suggests the optimal number and the best combination of the reference genes (34).
Comparative Delta Ct Method: This method compares the relative expression of gene pairs within each sample to identify the most stably expressed reference genes. For all gene pairs and across all samples, delta Ct values were calculated by subtracting the Ct value of one candidate gene from the Ct value of another within the same sample. The standard deviation (SD) of the delta Ct values was determined for each pair of genes and the mean SD for each candidate gene was calculated, based on its pairwise comparisons with the other candidate genes. Genes with lower mean SDs are considered more stable because their expression levels are less variable relative to genes with higher mean SDs (35).
BestKeeper Software: BestKeeper analyses the expression levels of up to ten candidate genes to identify the optimal reference genes through pairwise correlation analysis. Initially, genes are ranked based on the variation in their threshold cycle (Ct). Greater variation, represented by the standard deviation (SD) of the Ct values, points to lower expression stability. Genes with an SD exceeding 1 are viewed as unstable and are excluded. The software then computes the BestKeeper index for each sample as the geometric mean of all the stable candidate gene Ct values. The correlation between this index and individual genes is ascertained using Pearson correlation analysis. Notably, the most stable genes are characterized by a low SD value and a high correlation coefficient. Since BestKeeper can only analyze ten candidate genes and our panel had twelve, we excluded the two least stable genes (YWHAZ and TUBB5) based on the NormFinder results (36).
Finally, to evaluate the collective stability of the reference genes, we derived a comprehensive ranking using the geometric means of the ranks obtained from the described algorithms.
RESULTS AND DISCUSSION
Our study aimed to identify the most suitable reference genes for examining bone remodeling modulated by olanzapine. We evaluated the gene expression stability of our chosen candidate genes across four distinct sample groups: maxillary and femur bone tissues from rats, treated with olanzapine or given a placebo. Initial evaluation using descriptive statistics revealed that all the candidate genes hit the detection threshold with similar Ct values. This suggests consistent expression levels in both olanzapine-treated and untreated bones, be they alveolar or femoral. Of the genes, RRN18S displayed the highest expression levels, having median Ct values ranging from 7.64 to 8.01. In contrast, TBP was the least expressed, with Ct values between 27.55 and 28.75. Fig. 1 illustrates the distribution of Ct values for each gene across the sample groups. As expected, no amplification was observed in the NTC, confirming the absence of reagent contamination or primer dimer formation. In the NRT, low-level amplification was observed only for the most highly expressed gene, RRN18S, suggesting minor gDNA contamination. The Ct values were much higher in the NRT versus the mean Ct of the other samples (35.4 and 7.8, respectively), which indicates that less than 0.0000008 % of the total signal originates from gDNA, which was considered negligible. No amplification product was present in the NRT for the other candidate genes. For positive control, the amplification product was present in all the reactions at Ct values below 30.
To ascertain the most consistently expressed reference genes, we analyzed our results with the geNorm, NormFinder, and BestKeeper algorithms, in addition to the delta Ct and comprehensive ranking methods.
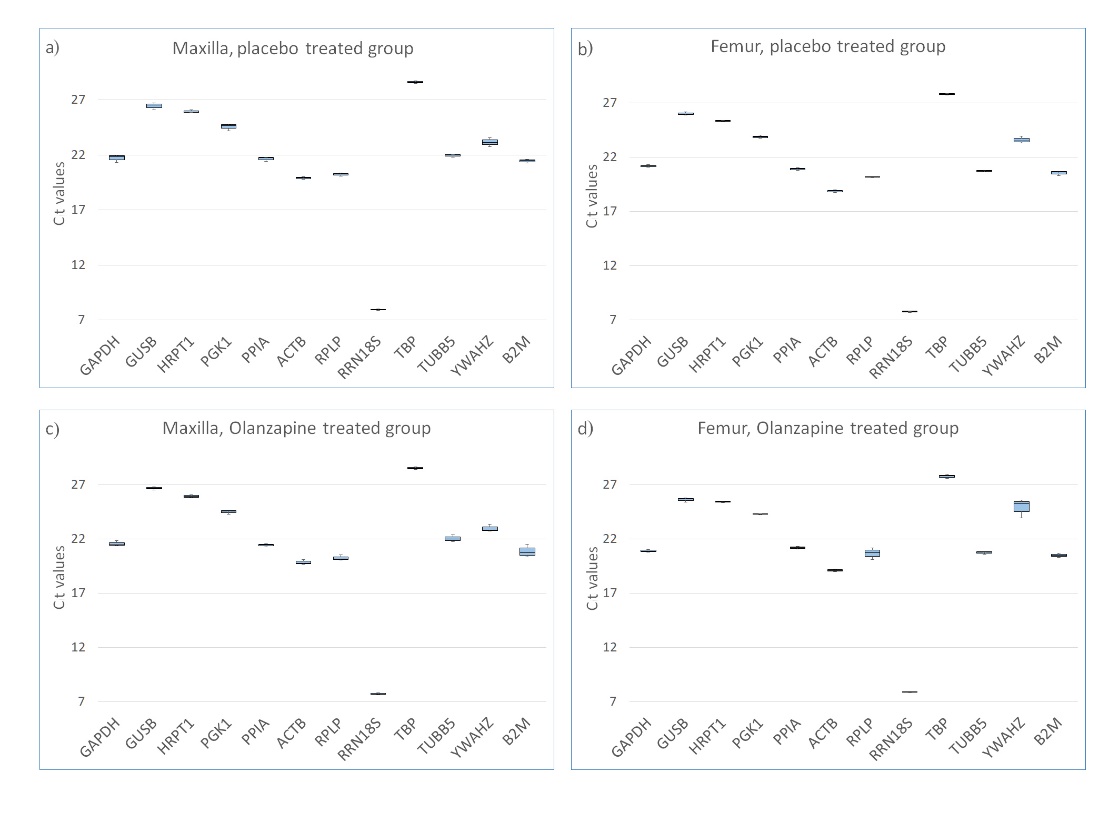
Fig. 1. Median and distribution of Ct values for each candidate gene obtained with RT‐qPCR in: a) maxilla in placebo-treated rats; b) femur in placebo-treated rats; c) maxilla in olanzapine-treated rats and d) femur in olanzapine-treated rats. Each box shows the lower 25th and upper 75th percentiles with median Ct values; the whiskers mark the minimum and maximum of the Ct values in each data set.
GeNorm analysis
GeNorm is a widely used tool for reference gene selection. It calculates the average pairwise variation between a candidate gene and all others, ranking them by their stability value, or M value. Genes with the lowest M values are considered to have the most stable expression. A recommended cut-off M value of 1.5 is used to identify stable genes. To determine the ideal number of reference genes for qRT‐PCR normalization, pairwise variation values were calculated with the cut-off set at 0.15. If below this threshold, adding another reference gene is unlikely to enhance data normalization (6). In our study, the M values for all the evaluated candidate genes were under 1.5, ranging between 0.151 and 0.506. ACTB and TBP emerged as the most stable genes, both having an M value of 0.151. The pairwise variation analysis indicated that the best number of reference genes for RT‐qPCR normalization was four, with the prime combination being ACTB, TBP, HRPT1, and PPIA (Table I and Fig. 2).
Table I. Expression stability of candidate reference genes. Ranking by GeNorm, NormFinder, and BestKeeper algorithms, delta Ct analysis, and comprehensive ranking using the geometric mean
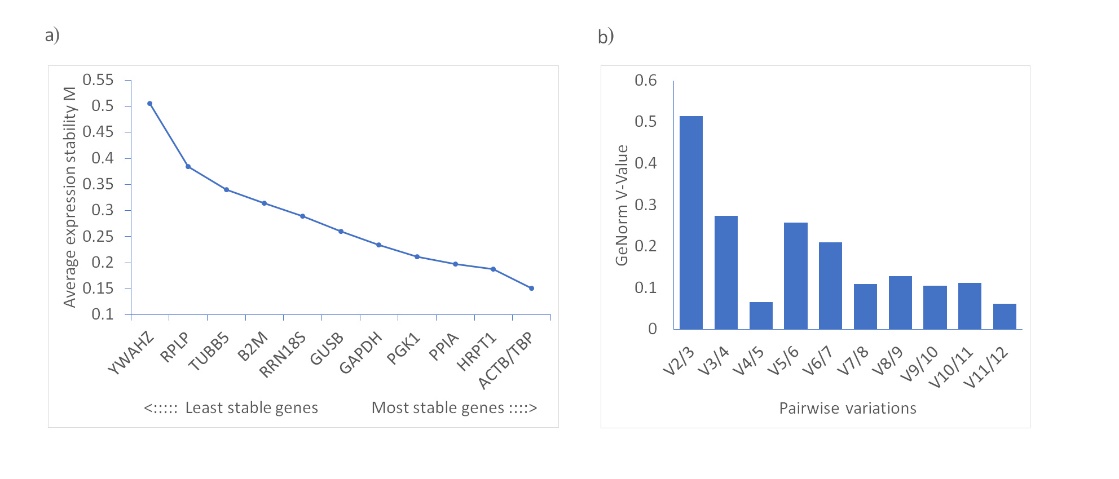
Fig. 2. Expression stability of candidate reference genes, calculated with GeNorm: a) ranking based on average stability numbers (M values); b) pairwise variation analysis for the determination of the minimal number of required reference genes for reliable normalization.
NormFinder analysis
Unlike GeNorm, which ranks genes by pairwise variation, NormFinder uses a mathematical model-based approach to estimate both intra- and intergroup variability in reference gene expression. This is then combined into a candidate gene's stability value (SV). The gene with the lowest SV is deemed the most stable. Additionally, NormFinder can determine the optimal number of reference genes for reliable normalization by computing the cumulative standard deviation as each gene is added (34). According to this algorithm, the optimal number of reference genes for investigating olanzapine-influenced bone remodeling is three. The prime trio consists of PPIA, HPRT1, and PGK1, with a combined SV of 0.047. Notably, these genes also exhibit the lowest individual stability values, at 0.065, 0.077, and 0.097, respectively (Table I and Fig. 3).
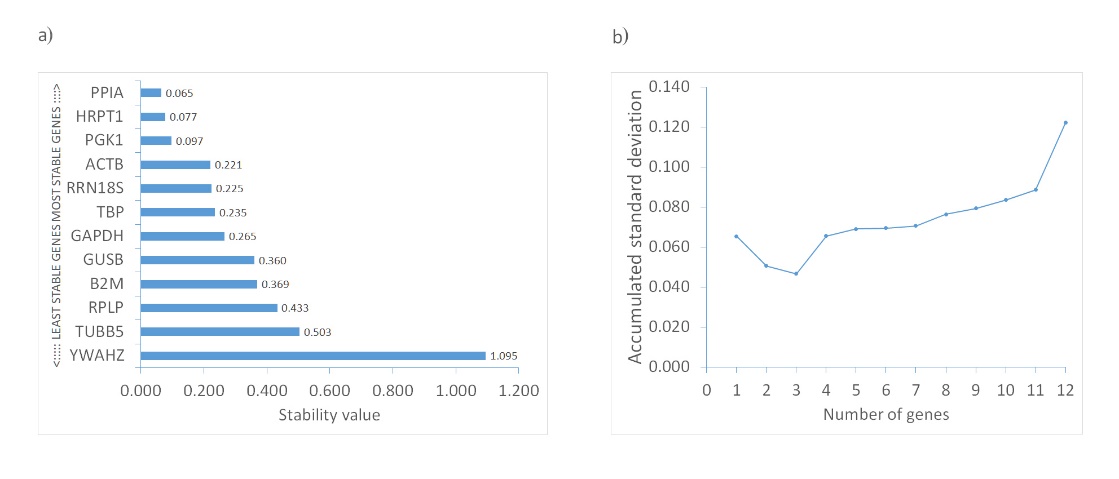
Fig. 3. Expression stability of candidate reference genes calculated with NormFinder: a) ranking based on calculated stability value (SV); b) determination of optimal number of reference genes using accumulated standard deviation calculation for each added candidate gene.
Comparative delta Ct analysis
The ΔCt method examines the relative expression between pairs of candidate genes within each sample, determining gene expression stability by evaluating the consistency of expression differences across the samples. In our study, the Ct values ranged from 7.8 for RRN18S to 27.8 for TBP. As shown in Fig. 4, PPIA and HRPT1 emerged as the most stable genes, with average standard deviation values of 0.37. Conversely, YWAHZ and RPLP were the least stable, exhibiting average SDs of 1.14 and 0.59, respectively (Table I).
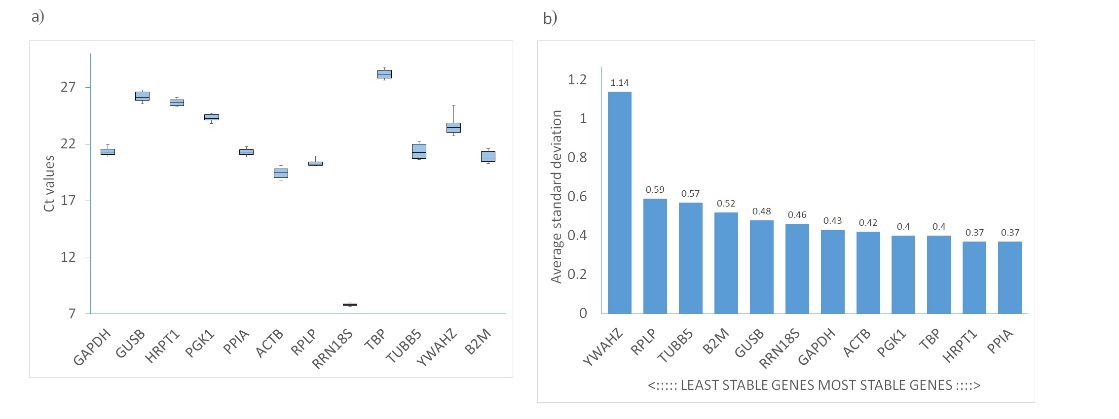
Fig. 4. a) Ct values of all candidate reference genes. Each box shows the lower 25th and upper 75th percentiles with median Ct values; the whiskers mark the minimum and maximum of the Ct values in each data set; b) expression stability determined using the comparative delta Ct method.
BestKeeper analysis
BestKeeper initially ranks candidate reference genes based on the standard deviation (SD) of their Ct values. In our study, the SD values of all 10 included genes were below 1, deeming every candidate gene stable. The most stable gene was RRN18S (SD = 0.08), followed in order by PPIA (SD = 0.24), PGK1 (SD = 0.25), RPLP (SD = 0.26), and HPRT1 (SD = 0.28). A deeper ranking involved calculating the pairwise correlation of candidate genes and their correlation with the BestKeeper index (Table I). RRN18S and RPLP were marked as unstable due to their weak correlation with the BestKeeper index, having correlation coefficients of 0.354 and –0.049, and p-values of 0.250 and 0.876, respectively (Table II). Consequently, these genes were omitted from further analysis.
Table II. Repeated pairwise correlation analysis of candidate reference genes vs. BestKeeper index (BK)
Comprehensive ranking
The GeNorm, NormFinder, and BestKeeper algorithms utilize unique methodologies to assess gene expression stability. While there was a general consistency in the rankings from each method, we observed some discrepancies in our results. Given the comparable reliability of all the methods, we aimed for a consensus by introducing a comprehensive ranking system. This system calculated the geometric mean of the individual rankings created by each algorithm.
From this consolidated analysis, three genes demonstrated notably consistent stability: PPIA, HRPT1, and PGK1, with a geometric mean (GM) rankings of 1.6, 2.3, and 3.2, respectively. Conversely, the genes YWAHZ, RPLP, TUBB5, and B2M were identified as the least stable, having GM rankings of 12.0, 10.7, 10.3, and 8.7 respectively, as detailed in Table I.
We assessed the expression stability of twelve candidate reference genes related to bone remodeling in rat alveolar (calvarial) bone and femur, under the influence of the antipsychotic drug olanzapine. These genes were selected based on their prevalent use in bone research. Their stability was determined and ranked using five distinct methods: GeNorm, NormFinder, the delta Ct method, BestKeeper, and a comprehensive ranking method.
Choosing appropriate reference genes specific to the experimental conditions is crucial for ensuring the reliability of results in expression studies using qPCR (37). In rat bone expression research, GAPDH and ACTB are frequently used as reference genes, often without prior validation. However, numerous studies highlight that these genes' expression can vary significantly based on the experimental protocols, tissue choice, and treatment, so the assumption that traditionally employed reference genes remain stable under all conditions is misguided. It is essential to select fitting internal controls tailored to the distinct conditions of each study (13, 37–45).
Kirschneck et al. (15) determined the most stable genes for dental, periodontal, and alveolar bone tissue in rats under different experimental conditions. They evaluated genes in control rats, those with periodontitis, and those with an applied orthodontic coil placed between the molars and incisors. Their findings suggested PPIB, YWHAZ, and ACTB to be the most stable under their overall conditions. Consequently, they recommended using PPIB and YWHAZ as reference genes in rat dental research.
In our study, we evaluated the stability of the candidate reference genes in alveolar and femoral rat bone, comparing groups treated with olanzapine to those given a placebo. The four algorithms used for ranking gene stability provided similar outcomes, with three genes, PPIA, HRTP1, and PGK1, consistently among the top five in stability. The other two varied: ACTB and TBP were highlighted by the GeNorm and delta Ct methods; ACTB and RRN18S by NormFinder; and GAPDH and GUSB by BestKeeper. A comprehensive ranking determined PPIA, HRPT1, TBP, PGK1, and ACTB to be the most stable candidates. Although BestKeeper initially ranked RRN18S as the most stable and included RPLP in its top five, their weak correlation with the BestKeeper index led to their exclusion from the final ranking. It is noteworthy that while most studies continue to use a single reference gene for normalization, this approach can cause bias and inaccuracies in up to 25 % of cases (6).
Our findings strongly recommend the use of multiple reference genes, especially when examining subtle differences in gene expression. According to GeNorm and NormFinder, the ideal number of reference genes for our experimental conditions stands at four and three, respectively. GeNorm recommends ACTB, TBP, HRPT, and PPIA, whereas NormFinder suggests PPIA, HRPT1, and PGK1. Notably, GeNorm identified TBP and ACTB as the most stable candidates, but these genes received considerably lower rankings from the other algorithms. This discrepancy could be attributed to GeNorm's inherent bias towards co-regulated genes. Based on our findings, we recommend utilizing a combination of PPIA, HRPT1, and PGK1 for studies on olanzapine-induced bone remodeling. Conversely, we caution against relying on B2M, TUBB5, RPLP, and YWAHZ, due to their consistently low stability rankings in our tests.
Our findings concur with several other studies, suggesting that ACTB, and especially GAPDH, have better-suited alternatives as reference genes for studying bone remodeling in olanzapine-treated rats. While our selection varies greatly from previously published results, it is crucial to note that most of those studies did not focus on bone tissue. Interestingly, none of the genes demonstrating high stability in our study ranked highly in our earlier models (46, 47). This underscores the importance of meticulously selecting reference genes before any target gene expression analysis. Tailoring this choice based on the study’s design and objectives can greatly enhance the quality of the results.
CONCLUSIONS
In this study, we emphasized the importance of tailored reference gene selection when examining bone remodeling modulated by olanzapine in rats. By employing methodologies such as GeNorm, NormFinder, BestKeeper, comparative delta Ct, and comprehensive ranking, we determined that traditionally used reference genes such as ACTB and GAPDH may not be appropriate for this specific research model. Our findings suggest the utilization of a trio of reference genes, PPIA, HRPT1, and PGK1, for more accurate normalization of RT-qPCR results in our model. This research accentuates the need for an optimal selection of reference genes, which should be harmonized with the study's protocol, tissue selection, and objectives to derive reliable and unbiased results.
Availability of data – The datasets generated and/or analyzed during the current study are available from the corresponding author upon reasonable request.
Conflict of interest – The authors declare no conflict of interest.
Funding– This work was supported by the P3-0293 research programme financed by the Slovenian Research Agency and Ad Futura – the Slovenian Human Resources Development and Scholarship Fund. The funders had no role in the design and conduct of the study, the analysis and interpretation of the data, or the preparation, approval, and submission of the manuscript for publication.
Authors contributions– Conceptualization, G.D, M.D. and J.M.; investigation, I.P.Ž. and S.D.I.; original draft preparation, G.D. and I.P.Ž.; review and editing, G.D., M.D., J.M. and I.P.Ž. All the authors have read and agreed with the published version of the manuscript.