INTRODUCTION
Social media is clearly viewed as a source of public discourse and people use it to share and receive information. The huge amount of online content on social media is crucial in the tourism industry, particularly regarding tourist decision-making processes (Distaso & Mccorkindale, 2017). This information created and shared by social media users, referred to as user- generated content (UGC), includes online reviews, comments, photos, and videos (Sangkaew & Zhu, 2022). UGC by social media users can attract new visitors and keep existing clients loyal be-cause today’s consumers trust electronic word-of- mouth (e-WOM) and online reviews more than advertising (Zhang et al., 2010). For example, WOM shared through mobile phones (mWOM) is determined by both relational and external elements (Velicia-Martin et al., 2023). In addition, these online reviews and ratings have a powerful influ-ence on consumer behaviour. For example, online opinion platforms such as Airbnb, TripAdvisor, and Google Maps reviews, are used as deciding tools for where to travel or what to eat, and what to buy based on the feedback pro-vided by other users (Von Helversen et al., 2018).
Food tourism has recently become a mainstream interest, as seen in the proliferating evidence on social media (The World Food Travel Association, 2020). So, in these online reviews, food is frequently one of the most discussed topics (Liu et al., 2019; Wang et al., 2017). Food photo posting and reviewing have become a recent trend on social media platforms (Javed et al., 2021; Wang et al., 2017). Tourists take food photos while travelling and post them on social media to show others where they dined and what local food they consumed (Tsai & Wang, 2017). Due to this ongoing expansion of UGC on social media, several studies have used this UGC data from restaurant reviews to explore consumer experiences (Mathayomchan & Taecharungroj, 2020), decision-making (Trivedi & Singh, 2021), and the quality of restaurant service (Mondo et al., 2022; Renganathan & Upadhya, 2021). Moreover, natural language processing (NLP) techniques such as sentiment analysis and text mining have been mostly used to analyse these online reviews.
Although previous studies performed sentiment analysis to describe food experiences on online platforms, the anal-yses were limited to investigating online reviews at restaurants (Mathayomchan & Taecharungroj, 2020). In order to consider the whole range of local food offer, different food-related experiences at the destination must be con-sidered. For example, local markets or street food as authentic food experiences have not been included in previous studies (Björk & Kauppinen-räisänen, 2019) and can provide a different view to local food tourism experiences. Moreover, previous research to analyse online reviews has not produced evidence to examine the impact of the COVID-19 pandemic on food travel experiences. Greater understanding and insights into consumer sentiment evo-lution regarding the COVID-19 pandemic are still lacking (Sangkaew & Zhu, 2022). Due to the coronavirus (COVID-19) pandemic at the beginning of 2020, the pattern of consumption behaviour can considerably change. The pandemic has made people worried about travelling, which has directly affected food tourism (Fountain, 2022). Nevertheless, there is no evidence that the public perceptions of customers’ food experi-ences through ratings and online
reviews changed after the outbreak of the pandemic. As a side effect of COVID-19, tourism brands and tourist destinations take into account the sentiments of customers on online platforms to develop strategies to generate business. It is thus vital to understand how COVID-19 affects the perception of food experience. There is, therefore, a need to address and better understand COVID-19 informational crisis and gauge public sentiment so that appropriate policy decisions can be implemented (Samuel et al., 2020).
Gastronomy plays a crucial part in attracting visitors to some destinations (Kivela & Crotts, 2006; Rousta & Jam-shidi, 2020). Food can be fascinating for tourists and has become part of some countries’ brand image. Eating out in a restaurant or café, enjoying street food, or visiting the local markets are part of the local food tourism offer (Yagoda, 2022). Given the tourism potential of local food, some public entities have launched campaigns to high-light and promote it as part of the national marketing strategy (Sritama, 2018). Thus, it is necessary to create a positive tourism experience for tourists and to understand which elements contribute to tourists’ behaviour, espe-cially in recent years when COVID-19 pandemic has completely changed the food tourism scenario. Thus, the main contribution of this article is to provide better knowledge on food tourism eWOM by considering the whole range of experiences and the effects that a pandemic may have caused.
The study aims to investigate the travellers’ food experiences by analysing the online reviews from Google Local Guide platform. In this research, the methodological contribution is to develop a novel approach to sentiment anal-ysis by adapting the techniques of word cloud generation and the n-gram algorithm. Sentiment analysis, often known as an opinion mining tool, is a type of natural language processing (NLP) from machine learning used to classify whether data is positive, neutral, or negative (Liu, 2012). A word cloud is a graphical representation of text data in the form of tags, which are frequently single words whose significance is visualised by their size and colour. A novel method of N-gram feature is a classification model based on the set of co-occurring words present in corpus text.
Therefore, to provide a comprehensive understanding of the travellers’ perspectives on food experiences at the destination, the research questions are the following: (RQ1) What are the travellers’ sentiments towards food expe-riences found in Google Maps reviews?; (RQ2) What are the major attributes of food experiences that people share on online reviews?; (RQ3) Are there differences in opinions about food experiences before and after COVID-19 restrictions were eased and allowed them again?
The remainder of the paper is organised as follows: after this introduction, a thorough review of related literature on food tourism experience, COVID-19 perceptions, online reviews and sentiment analysis is provided. The methodol-ogy employed in the study is explained in section 2. Section 3 presents the results and analysis of the data, followed by the discussion in section
4. Section 5 provides the implications of the study. Finally, the conclusion of this paper along with its limitations is given in the last section.
LITERATURE REVIEW
Food experiences affect affinity to a place and the tourism destination image (Choe & Kim, 2019). Firstly, the rela-tionship between food and tourism is presented. Then, the public’s perception of food during the COVID-19 pan-demic and its effect on tourism is reviewed. Finally, the important role of social media and online reviews is ex-plained.
Food Experience and Tourism
Food and tourism are undeniably related. Food and beverages are a vital part of the travel experience (Wang et al., 2017). Gastronomy significantly affects the tourism industry. Kim et al. (2009) demonstrate that food and eating out are important activities on trips and holidays. Gastronomy in each region represents that place’s cultural uniqueness, which plays an essential role in attracting tourists to the destination, especially in Asian countries like China, India, Japan, Thailand, and Vietnam, which is a prominent gourmet destination for travellers (Kivela & Crotts, 2006). Chen & Huang (2016) describe four elements in the relationship between food and tourism. Food is cultural identity; food is an essential part of tourist attractions; food is a tourism product; and food is a crucial part of the travel experience. Food experiences are descriptions of how people feel when dining out or engaging in food-related activities. By interacting with the food, one can experience a culture in a variety of ways, such as eating out, visiting food markets, grocery shopping, other food-related activities, festivals or events, cooking classes, and food tours (Cohen et al., 2014; Kivela & Crotts, 2006; Zhang et al., 2010).
More and more people are searching for food activities through social media platforms. Customer reviews, feed-back, and product rating scores influence their choices (Lu & Stepchenkova, 2015). Food is an attraction that in-spires people to travel (Choe & Kim, 2019). A thorough review of studies reveals that food experiences are not only about the cuisine, it is also about the personnel, the service, the environment, the atmosphere, the decorations, etc. (Desmet & Schifferstein, 2008; Pérez Gálvez et al., 2017; Young et al., 2018).
As seen in Table 1, there are many different factors that contribute to dining experiences. Kleinhans et al. (2016) summarised the dimensions influencing the dining experience. They identified food, physical environment, ambi-ence, service, price, health/ cleanliness, and social factors are the prominent aspects of the dining experience. Jeaheng & Han (2020) found nine dimensions
that tourists are concerned regarding street food consumption: cul-tural and local experiences, menu and atmosphere, staff service, core food quality, value for money, product at-tractiveness, staff proficiency, packaging and portions, and tradition and authenticity. According to Stone et al. (2018), elements leading to memorable food experiences are diverse. They found five essential elements that help tourists have memorable culinary experiences. These attributes are food/beverages, location/ setting, companions, occasion, and touristic elements (Choe & Kim, 2019). In the research by Hyun (2010), food quality, service quality, price, location, and environment are the key attributes that influence restaurant customers’ decisions. Regard- ing sharing memorable experiences on mobile social media, findings by Wong et al. (2020) indicated scenery, enter-tainment, and interaction are three dimensions affecting tourists’ sharing behaviour. Mathayomchan & Taecha-rungroj (2020) explore the principal attributes of customer experiences in restaurants in the UK posted on Google Maps reviews. They present four dimensions: food, service, atmosphere, and value (price).
Table 1: Analysis of food experience dimensions
Dimension Description in previous literature References
Food / Menu / Product
Physical environ- ment / Ambience characteristics
Food quality, food tastes, packaging and portions, food attractiveness.
Characteristics of the physical environment, such as health and cleanliness.
Hyun (2010); Jeaheng & Han (2020); Kleinhans et al. (2016); Mathayomchan & Taecharungroj (2020); Stone
et al. (2018).
Hyun (2010); Jeaheng & Han (2020); Kleinhans et al. (2016); Mathayomchan & Taecharungroj (2020); Wong
et al. (2020).
Service Service quality, staff proficiency. Hyun (2010); Jeaheng & Han (2020); Kleinhans et al.
(2016); Mathayomchan & Taecharungroj (2020); Stone
et al. (2018).
Place / location / setting
Where the food experience takes place. Hyun (2010); Stone et al. (2018).
Price / value for money
Evaluation on the value for money of the food.
Hyun (2010); Jeaheng & Han (2020); Kleinhans et al. (2016); Mathayomchan & Taecharungroj (2020).
Activity Touristic elements; entertainment; activities
linked to the food experience.
Social atmosphere Social factors; Cultural and Local experienc-
es; tradition and authenticity; companions; occasion; interaction
Stone et al. (2018); Wong et al. (2020).
Jeaheng & Han (2020); Kleinhans et al. (2016); Stone et al. (2018); Wong et al. (2020)
Food Perception during the COVID-19 Pandemic
The spread of the COVID-19 crisis has significantly reduced global travel since March 2020. The tourism sector has suffered tremendously from this dramatic drop in international travel (Škare et al., 2021). Gastronomic tourism has been one of the sectors most affected by the COVID-19 pandemic (Fountain, 2022). In Thailand, tourism activity based on food, particularly in restaurants, markets, festivals, bars, cafes, and shopping malls, was suspended by order of the government (Sereenonchai & Arunrat, 2021). The sudden decline in the number of customers forced many eateries to close.
After the COVID-19 shutdowns, countries around the world have opened up again, but businesses are struggling to devise strategies to survive (Richards, 2020). According to reports, some consumers are concerned about food cooked outside the home (International Food Infomation Council, 2020). People have changed their dietary habits to meet their perception of the level of food security (Thomas & Feng, 2021; Janssen et al., 2021). This is consistent with a previous study in which health concerns contribute significantly to whether tourists consume local food (Choe & Kim, 2019). Dedeoğlu et al. (2022) examined Chinese tourists’ different perceptions of food consumption in Italy and Thailand during COVID-19. The result found that travellers to Thailand perceive the risks of contract-ing COVID-19 while consuming local food there as low, which has a positive effect on their intention to consume that food, while the perceived risk is higher in Italy, which has a negative effect on travellers’ intentions to consume food there. This is because tourist attitudes toward food consumption are a significant factor in their behaviour when visiting tourist destinations (Young et al., 2018). Jeaheng & Han (2020) studied the effect of Thai street food on tourists’ post experiences and their perceptions during their visit to Thailand. They found that the variable of the perceived risks of consuming Thai street food plays a significant role as a moderator.
The role of COVID-19 in the relationship between customer satisfaction and behavioural intentions in local food consumption has been the focus of several studies. Rehman et al. (2022) revealed the degree to which tourists’ intention and satisfaction with local food are related to their anxiety about COVID-19. However, there are still re-search gaps in this regard the impact of COVID-19 on food experiences posted on online reviews.
Online Reviews
Social media platforms have become the search engines many people use to decide when and where to travel or dine. They have an enormous influence on the decision-making process of most potential travellers (Distaso & Mccorkindale, 2017). Increasingly, the increase of user-generated content (UGC) on social media platforms and the constant posting of travel destinations have become prominent ways to enhance people’s travel plans. Tourism and hospitality customers can easily share travel-related content, resulting in the widespread availability of UGC (text, images, video, and audio) on social network sites (Lu & Stepchenkova, 2015). What is more, these experiences come from interactions that people have with brands (Wong et al., 2020).
There is some evidence that these media often motivate people to travel (Liu et al., 2019; Bakshi et al., 2019). Likewise, the feedback from customers on social media has a remarkable effect on the image of a destination. Electronic word-of-mouth (e-WOM) is particularly influential in decisions related to accommodations, travel ex-penses, and transportation. In contrast, e-WOM from local residents has a greater effect on decisions about gas-tronomy and safety (Lu & Stepchenkova, 2015). Ratings and reviews play an enormous role in purchasing deci-sions. Users can give good and bad feedback on the brand. Tantrabundit (2018) indicated that the negative opin-ions of previous customers could have a greater effect on changing new customers’ purchasing decisions than posi-tive ones. Positive reviews can inspire future customers to visit a destination. On the other hand, if the feedback is negative, the atmosphere of trust can be instantly undermined (Li et al., 2022).
Many scholars have reviewed data from online communities like Twitter, Facebook, TripAdvisor, and Airbnb to examine clients’ perceptions of brands (Amadio & Procaccino, 2016; Leung et al., 2013; Nicolau et al., 2020; Santos et al., 2022). For example, the database from Twitter was used to explore purchasing behaviour re-garding tourism services (Philander & Zhong, 2016). Some studies analysed online reviews from TripAdvisor and used sentiment analysis to explain tourists’ experiences (Sangkaew & Zhu, 2022; Taecharungroj & Matha-yomchan, 2019; Stoleriu et al., 2019). Reviews on Google Maps, known as Google Local Guide reviews, have been mentioned in tourism and hospitality research for a few years (Taecharungroj & Mathayomchan, 2019; Shin et al., 2022). The Google Local Guides, now available on Google Maps, not only help users to find a desired destination and get around, but also to connect with other Google Maps users. Besides providing maps and routes, Google Maps allows users to view and share real-time experiences during visiting destinations.
With the growing influence of Google Maps (Georgiev, 2022), it needs to be understood by looking at users’ experi-ence sharing on this application. To fill this gap, this study will reveal the perceptions of tourists’ experiences of food in tourist destinations using review data from Google Local Guides.
Sentiment Analysis
Sentiment analysis refers to classifying subjective texts’ emotional status and attitudes with implicit sentiments (Avinash & Sivasankar, 2019). It is generally used for analysing textual data to gauge the ratio of sentiment value of user feedback on social media and online platforms (Garcia & Berton, 2021; Jain et al., 2021; Santos et al., 2022; Shin et al., 2022; Taecharungroj & Mathayomchan, 2019). Sentiment analysis, including text mining and natural language processing (NLP) techniques, assists in detecting users’ opinions toward the brand and product from customer reviews (Suresha, 2020). Based on the polarity score, the categories of emotions in a statement can be assessed to be positive, negative, or neutral (Imran et al., 2022). In terms of sentiment classification, there are different options to do so. Many authors use different machine-learning (ML) approaches to perform textual analysis (Islam et al., 2021; Samuel et al., 2020; Srivastava et al., 2019; Taecharungroj & Mathayomchan, 2019). For example, Garcia and Berton (2021) use multiple ML algorithms and features in a comparative analysis of their methodology to examine the COVID-19-related tweets from Brazil and the United States. In their study, Twitter posts were analysed using sentiment analysis and topic modelling approaches. Saura et al. (2019) performed sentiment analysis with a Supervised Vector Machine (SVM) algorithm to identify key factors in UCG on Twitter for the development of successful startups. Boon-Itt & Skunkan (2020) studied topic modelling based on unsupervised machine learning to collect tweet data for public perception of COVID-19. A lexicon-based approach was adopted to demonstrate eight basic emotions: anger, fear, anticipation, trust, surprise, sadness, joy, and disgust. In the study, the most common topics in the tweets were visualised by unigram feature through word clouds. TextBlob Python library is also used to determine positive, negative and neutral sentiments based on polarity scores on online reviews (Aljedaani et al., 2022, Imran et al., 2022, Naseem et al., 2021).
Qualitative data analysis software, such as Nvivo or MaxQDA, has also been employed as a research method to explore the sentiments (Santos et al., 2022; Sánchez et al., 2022; Saura et al., 2018; Saura et al., 2019). For example, Saura et al. (2019) performed sentiment analysis to identify key factors in UGC on Twitter for the development of successful startups and Santos et al. (2022) analysed Airbnb users’ experiences in a Brazilian tourist destination.
METHOD
This research analyses Google Local Guide reviews to study online opinions of travellers’ food experiences. The study focuses on dining experiences posted in reviews in Ayutthaya city, Thailand. Phra Nakhon Si Ayutthaya or Ayutthaya is chosen in this research because it is a popular destination in Thailand for both foodies and tourists due to many restaurants, food markets, floating markets, night markets, and outstanding street delicacies (see Appendix 1). The Ancient City of Ayutthaya is a significant cultural site in Thailand, and it has been a UNESCO World Herit-age Site since 1991 (UNESCO, 2023). Along with its outstanding historical attractions, Ayutthaya’s traditional cui-sine highlights its unique culture and history. Around 8 million tourists visited Ayutthaya in 2018, making it one of Thailand’s most famous tourist destinations (Ministry of Tourism and Sports of Thailand, 2018). Besides, Ayuttha-ya has been recently selected by Michelin Guide as one of five prominent gastronomic cities in Thailand (Michelin Guide, 2021).
The methodology of the sentiment classification system used in the study, we performed the script programmed in Python coding language. The researcher processes data using Colaboratory (Google Colab) as the software. The Python Programming language was coded through Google Colab for data analysis (Google, 2021). The reason we chose Google Colab for running Python models is that it is a free Jupyter notebook in the cloud created by Google. Another attractive feature is that Google Colab supports many machine learning libraries that are simple to load in the notebook. The research scheme begins with data collection from Google Maps reviews described in Section 2.1. Next, the procedure of data preparation was conducted as presented in section 2.2. Finally, section 2.3 details the evolution of NLP-based sentiment analysis performed in the study. The methodology applied in the study is shown in Figure 1.
Review of Dataset and Data Collection
The data in this study was collected from Google Local Guide platform by searching key terms and using web-scraping techniques. The extracted data is saved in an unstructured CSV file with the following information of each comment: name of the food location, place coordinates, global place rating, review rating, review date, number of likes, link to review images, and textual comment.
In order to select the comments, the first key term searched in Google Maps is “restaurants or cafes” and the zip code of Ayutthaya city is introduced to filter the ones located in this area. Then, in order to find the websites of the seven famous landmarks related to food events, the following terms are searched: “food market”, “street food market”, and “floating market”. These seven gastronomic landmarks are: the Ayutthaya Floating Market, the Krung Si Ayutthaya Market, the Ayutthaya Night Market, the Bang Ian Night Market, the Chao Phrom Market, the Ayutthaya central shrimp market (Talat Klang Market), and the Ayutthaya Municipal Market (Na Wang Market). Finally, 35,001 comments were retrieved and grouped into two separate datasets. The first dataset of 22,516 posts contains reviews posted before the COVID-19 pandemic, from January 1st, 2016, until January 12th, 2020, while the second set of 12,485 posts contains reviews shared during the COVID-19 pandemic from January 13th, 2020 until August 18th, 2022. The data are divided in this way because on January 13th the Ministry of Public Health declared the first case in Thailand and people began to perceive the risk derived from this (Plipat, 2020).
Data pre-processing
Python programming language was used to prepare the raw data in this step. The primary libraries imported for data pre- processing were Pandas (to put text data into data frames), NumPy (to create number sequences), and Matplotlib (to visualise the data). The data pre-processing stage is similar to the data transformation technique in that it cleans and prepares text data before feeding it to the natural language algorithm (see Figure 1 and Table 2). NLTK (Natural Language Toolkit) for natural language processing was mainly used in this study. In the first step, all of the gathered reviews from Google maps were transformed into a data frame. The dataset from the first extrac-tion was cleaned by removing any punctuations, numbers, website links, and emojis in the text. Moreover, the re-trieved terms were converted to lowercase letters. Stop word removal was then launched using a stop word list by the NLTK corpus package. Stop words are used to filter out closed words without lexical meaning in the text analy-sis (e.g. “a”, “the”, “I”, “is”, “are”, “and”, “of”, “for”, “about”) (Hickman et al., 2020). In the next step, the reviews were broken down into words and each word was stored individually for further use in an analysis called “tokenisa-tion”. Tokenisation refers to the process of splitting a sentence, or text into a list of single words or “tokens” that can be considered as discrete units (Banks et al., 2018). Lastly, Lemmatization is carried out. It is an efficient pre-processing technique that enhances model performance by analysing the word morphology and reducing words to its lemma (Ahuja et al., 2019). In other words, the aim of lemmatisation is to inflect words to their common roots (e.g. came to come, eats to eat). The Spacy lemmatiser function, a popular NLP system, was used to perform word transformation in this step. Figure 1 and Table 2 present the overview and examples of the preprocessing stage.
Table 2: Example of output of preprocessing stage
After cleaning the text, exploratory data analysis reveals insights about the datasets. The statistical description of the two datasets is computed. Next, sentiment analysis uncovers the emotional direction behind the words. The TextBlob text processing library for natural language processing was then used to classify sentiments as positive, negative, or neutral. The TextBlob library provides the rules-based sentiment score function. TextBlob gives a text’s polarity and subjectivity scores for sentiment
classification. Reviews with polarity are determined as positive, nega-tive, or neutral sentiments based on polarity scores. Text is defined by a value in the range [-1 = negative, 0 = neu-tral, +1 = positive]. The level of subjectivity can reveal which reviews contain personal opinions and feelings rather than facts [0 =objective, 1 = very subjective].
Table 3 illustrates an example of the sentiment output automatically computed by this procedure. Then, the word cloud module is carried out to visually represents the frequency of words contained in the reviews. Word cloud is a graphical representation of text data in the form of tags. The relative importance of individual frequency words in the textual contents is indicated by their size and colour. Thus, the word cloud package in python is imported to generate customised word clouds. In the following step, the N-gram model is used for feature extraction in senti-ment analysis (Avinash & Sivasankar, 2019). In technical terms, the N-gram model is considered as a bag-of-words feature that involves collecting the occurrence of words from given speeches based on a contiguous sequence of n words. For example, for the sentence “Thai food delicious”, if n = 1 (called unigrams), then the N-grams are “Thai” while if n = 2 (called bigrams), then the n-grams are “Thai food”. If n = 3 (called trigrams), then the N-grams are “Thai food delicious”. To generate the N-gram model, the CountVectorizer method is used to reform the corpus of tokens, or individual words, into a matrix form of N-gram counts. In this process, we employ the CountVectorizer package from the Python scikit-learn library to build the matrix of token counts. With the bigram model, different terms were grouped to identify the main dimensions of sharing food experiences on Google Maps reviews. In order to answer the three research questions of the study, all these analyses (i.e. TextBlob Sentiment Analysis, Word clouds, word frequencies, N-gram models) are combined together.
Review lengths and word count results reveal online user behaviour related to sharing food experiences on social media. In the 35,001 reviews on food experiences on Google Local Guides, people usually write feedback of approximately 98 characters, including text, emojis, and symbols. Most reviews have an average of 17 words. Figure 2 presents the rating scores given by customers in studies. Remarkably, the average ratings given in reviews before and during the pandemic are both above 4 (4.06 and 4.16, respectively). Most people who had food experiences in Ayutthaya gave a score of 5.0, meaning they were delighted with their experiences at the destination. The unexpected finding was that despite concern about hygiene and social distancing due to the COVID-19 pandemic, people gave establishments higher ratings than before the pandemic. However, there was only a slight difference in percentages between the two situations.
Figure 2: Review rating comparison of food experiences given in reviews before and during the pandemic
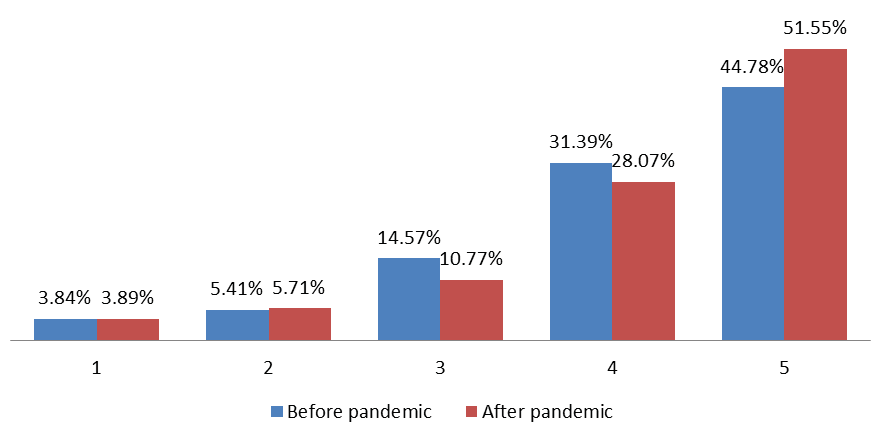
Source: Own elaboration
Sentiment analysis
Figure 3 presents the sentiment polarity obtained based on the score in reviews. The polarity is classified into positive, neutral, or negative sentiment for the whole period. Most customers have a positive attitude about food experiences, followed by neutral and negative feelings, respectively. Regarding public opinion related to food events before the pandemic, 72.58% of the reviews expressed positive sentiment, followed by the neutral feeling (20.99%) and the negative sentiment (6.44%) (Figure 3a). This is consistent with tourists’ sentiment trends for food reviews during the pandemic. During the pandemic, the number of positive reviews increased to 76.87%. In contrast, neutral views fell to 16.76%. In a similar trend, negative sentiment slightly decreased (6.37%) in a reflection of attitudes about food activity during the pandemic (Figure 3b). When compared, the overall results of the two sentiment datasets are interesting. There was no statistically significant difference in all three sentiments in the reviews before and during the COVID-19 pandemic. This indicates that customers visiting Ayutthaya frequently expressed satisfaction with their food experience during both periods.
Figure 3: Comparison of sentiment polarity on review data toward food experiences
Source: Own elaboration
(a) before pandemic (b) during pandemic
Word cloud analysis
After a sentiment analysis, word cloud analysis was applied to explain the further sentiment results by extracting the most frequency of terms in reviews. Figure 4 shows word cloud outputs widely posted on Google Maps reviews related to food experiences before and during the COVID-19 outbreak. Before COVID-19, the leading terms of positive sentiment are “food”, “good”, “fresh”, “shrimp”, “delicious”, etc. (see Figure 4a). Similarly, some positive keywords are found to be the same before and during COVID-19, comprising “food”, “good”, “fresh”, “shrimp”, “delicious”, etc. (see Figure 4b). In term of neutral sentiment on food experiences, before COVID-19 is characterised by “food”, “star”, “service”, “shop”, “like”, etc. (see Figure 4c), whereas the top words during the pandemic are “shrimp”, “food”, “eat”, “go”, “think”, etc. (see Figure 4d). No similar terms are found in the case of neutral sentiment, except for “food”. Word cloud results of the negative sentiment that characterised the food experiences before the pandemic include “service”, “taste”, “hot”, “chicken”, “large”, etc. (see Figure 4e), while during the pandemic, different keywords with negative sentiment can be found, namely “expensive”, “price”, “taste”, “issue”, “matter”, etc. (see Figure 4f).
Figure 4 The distribution of word clouds towards food experiences before and during the pandemic
(a) positive before the pandemic (b) positive during the pandemic
(c) neutral before the pandemic d) neutral during the pandemic
e) negative before the pandemic (f) negative during the pandemic
Source: Own elaboration
The intriguing aspect of these findings is that the travellers tended to be satisfied with food in destinations even during the COVID-19 pandemic. The finding pointed out that consumers favoured dishes like shrimp and seafood for their freshness and flavour. These results were consistent with the fact that fresh fish and river prawns are popular local dishes for foodies visiting Ayutthaya. The results also reveal that, besides the food, the quality of service and the ambience also make significant impressions on customers, particularly during the COVID-19 pandemic. This would be due to intense competition among food businesses during the COVID-19 outbreak. Without neglecting hygiene, businesses look for policies that increase trust and reflect customers’ concerns. Customer service strategies become a solution to the problem of recovering business. Businesses are becoming more conscious of the importance of service quality. This is obvious in the pre-pandemic result that service implies dissatisfaction with food experiences. Another critical word cloud result, price, is detected to be unpleasant as inexpensive, which business owners need to take into account. When consumers express their dissatisfaction online, it is possible that they will include information that can be useful to the owners of the businesses. This is because understanding the root causes of customer complaints can be a powerful tool in determining in which order to address issues.
Bigram analysis
Bigram analysis provides empirical output to support the opinions expressed in the review. The N-gram model is applied to evaluate the research approach. According to the findings, the bigrams work better than the other N-grams because two consecutive words give a better view to describe each sentiment (Ahuja et al., 2019). Bigram analysis helps to observe the principal terms that customers often mention in their comments (Srivastava et al., 2019). The sentiment outputs aggregated into the same polarity class from the previous section identified trends in co-occurring and correlated words for the proximity context around each word. The findings provided the research-ers with information that assisted them in determining the underlying terms that exist in the reviews of food experi-ences at the destination. The tendency of food experience sentiment mentioned in the reviews is classified as de-picted in Figure 5.
Figure 5: Bigram analysis of the review text
Source: Own elaboration
Throughout the bigram results, several terms were grouped to identify the crucial dimensions of sharing food experiences on Google Maps reviews (Figure 6). According to review data of tourists in Ayutthaya collected before COVID-19, there are six groups of sharing food experience aspects (summarised in Figure 6a). Reviews of food and products (e.g., “delicious food”, “good food”, “fresh shrimp”, “variety food”) are what people mostly share in a positive way on Google Local Guides, followed by atmosphere (e.g., “nice atmosphere”, “good atmosphere”, “lot people”, “good view”), service (e.g., “parking lot”, “good service”, “bad service”, “wait long”), and place (e.g., “float market”, “nice place”, “night market”). Price (e.g., “good price”, “reasonable price”, “quite expensive”) and activity (e.g., “boat ride”).
Compared with the bigram result during the pandemic, food and product (e.g., “delicious food”, “good food”, “Tom Yum”, “Thai food”), atmosphere (e.g., “good atmosphere”, “good view”, “nice atmosphere”,). and service (e.g., “good service”, “service staff”, “terrible service”) are still the main dimensions of sharing food experiences at destinations (Figure 6b). It is noteworthy that the term service is mentioned much more frequently during the pandemic than prior to it. The topics of price (e.g., “price expensive”, “worth price”) and place (e.g., “float market”, “eat restaurant”) are discussed in smaller proportions. Posting regarding the activities in the reviews is not found as the main topic during the pandemic. It is surprising that the topic of food-related activities does not appear. Four dimensions – food and product, atmosphere, service, and price – have a positive valence, while the term place seems to tend to neutral to negative valence.
DISCUSSION
Examining reviews left by users on Google Local Guides helps to understand the tourists’ sentiments towards food experiences in tourism destinations. According to the results of sentiment analysis and sentiment polarity, and an-swering RQ1 (What are the travellers’ sentiments towards food experiences found in Google Maps reviews?), the reviews by users of food-related activity on Google Local Guides mainly represent positive emotions. This is in the same line with previous research, indicating that positive emotions rank highest across all restaurant categories (Renganathan & Upadhya, 2021). The study’s findings provide credence to the hypothesis put up by Islam et al. (2021) or Sangkaew & Zhu (2022), that travellers are more likely to talk favourably than negatively about their dining experiences or their food market experiences, respectively. This may be due to tourists’ satisfaction with culi-nary activities motivating them to share their experiences on social media (Yang, 2017).
The word cloud and N-gram analyses reinforce each other to clearly describe the key elements of the food experience in online reviews to answer RQ2 (What are the major attributes of food experiences that people share on online reviews?). This research develops a sentiment analysis approach to explore multidimensional terms frequently found for reviewing food experiences on the Google Maps platform. The empirical results establish six major dimensions of sharing food experiences on Google Maps reviews that are contrasted with previous literature on the topic, as seen in Table 4.
Table 4: Analysis of food experience dimensions
Dimension Words Description in previous literature*
Food / Menu / Product
Physical environment
/ Ambience characteristics
Food (e.g., TomYum, shrimp, seafood), dessert, beverages, the variety of food, tastes, and food quality
Environment, atmosphere, ambience, clean, construction, dirty, surrounding, tidy, wide, decoration, view, and smell
Food quality, food tastes, packaging and portions, food attractiveness.
Characteristics of the physical environment, such as health and cleanliness.
Service Employee, waiter, staff, agent people, parking, time, close, open, speak, wait, service and slow
Service quality, staff proficiency.
Place / location / setting
Eating establishments, restaurants, shops, and markets.
Where the food experience takes place.
Price / value for money
Price, fee, expensive, cheap Evaluation on the value for money of the food.
Dimension Words Description in previous literature*
Activity Type of other activity participation (e.g., cruising, boat, elephant ride)
Touristic elements; entertainment; activities linked to
the food experience.
Social atmosphere - Social factors; Cultural and Local experiences; tradition and authenticity; companions; occasion; interaction
*See Table 1 for references.
First, theme “food and product”, which represents the variety of food and products, food tastes, and food quality (Jeaheng & Han, 2020; Stone et al., 2018) is the topic that travellers most frequently comment in the reviews. This is logical as the food is the main element of these experiences. The food and product attributes are mainly men-tioned in positive comments and followed by the neutral feedback. This reflects that the most crucial criterion in users’ perception is the element linked to the food (Alqadi et al., 2020). The factor of taste/quality is found to have a positive effect on tourists’ attitudes toward local food (Rousta and Jamshidi, 2020). Thus, the perception of taste and quality is a crucial factor that tourists use to explain how they enjoy their food activities (Choe & Kim, 2019).
Second, “atmosphere” is frequently mentioned when describing experiences. Physical environment factors are often considered in research on gastronomic tourism (Hernandez-Rojas et al., 2021; Pattanapokinsakul & Phakdee-auksorn, 2015; Privitera et al., 2018). This is probably because most tourists are motivated to visit food destinations by the unique atmosphere of the places where they experience them (Pattanapokinsakul & Phakdee-auksorn, 2015; Privitera et al., 2018). Additionally, atmosphere related to food experiences (Chi et al., 2013) plays a signifi-cant role in food satisfaction and tourists’ behavioural intentions. Results of this study also support the fact that atmosphere is one of the most prevalent topics in positive reviews, which indicates higher satisfaction levels derived from this dimension.
Third, “service” dimension is also addressed in the comments. Positive service refers to being service-minded and friendly, while negative service indicates the opposite, terrible customer service. The quality of service is one of the critical elements of a memorable food experience affecting restaurant satisfaction (Stone et al., 2018). Alqadi et al. (2020) support the conclusion that service is one of the top three attributes on the tourist satisfaction scale.
Fourth, “place” dimension refers to the eating establishments and local markets, such as floating markets and night markets. Visiting food attractions such as local markets, food festivals, restaurants, and unique places to taste spe-cial dishes is a typical tourist behaviour (Privitera et al., 2018). The term place is mostly associated with optimism before the pandemic. However, place-related reviews after the pandemic tend to highlight negative experiences. Tourists are curious to taste local food when they travel (Kim & Eves, 2012) but, as we can see in the results of this study, if this experience is constrained, it may affect the location evaluation.
Fifth, “price” is an important element in motivating and influencing customers’ purchasing decisions. The food pric-es attract tourists to different destinations because they are reasonable, fair, good for value, etc. For example, street food is well known all over the world for its affordable prices (Jeaheng & Han, 2020). In tourists’ behavioural mod-els, it is proved that the price component directly affects the quality perception of the product or service by con-sumers (Forgas-Coll et al., 2012), in line with what is found in this study, where text references to price are directly linked to both positive or negative opinions.
Sixth, the term “activity” relates to the travellers’ experience gained from food tourism such as activities while visit-ing local markets, floating markets, or street food as well as a tour with dinner on a local river cruise. The dimension of posting relevant activity was mentioned and supported by previous research (Wong et al., 2020). This indicator refers to participating in various forms of entertainment activities with food and beverage and tourism. Sharing activities on social media while travelling is confirmed by Javed et al. (2021), as they found that uploading or shar-ing any activities on online communities is a form of self-expression. However, compared with the food experiences during the pandemic, this feature was largely absent from online review sharing during the pandemic. This may be a result of Thailand’s suspension of certain tourism activities at the beginning period of COVID-19. To revitalise activ-ities related to food tourism, this opportunity should be taken to promote gastronomic activities in Ayutthaya, such as food festivals, street food or food tours. The factors of taste/quality, value, and price value have a positive ef-fect on tourists’ attitudes toward local food (Rousta & Jamshidi, 2020), while the foodscape dimension, consisting of elements like place, atmospherics, food image, and cultural society have a significant role in building up better food memories (Björk & Kauppinen-Räisänen, 2019). Thus, the author recommends considering these elements for further research to develop and comprehend the gastronomic tourism content.
Finally, in this study, no dimension on social atmosphere has been separately defined as it happens in previous literature (Jeaheng & Han, 2020; Kleinhans et al., 2016; Stone et al., 2018; Wong et al., 2020). However, this social atmosphere is directly linked and mentioned in the text from other dimensions. For example, in the physical envi-ronment dimension, there is also a reference to social interaction and behaviour during these experiences. Thus, in an indirect way, the social component of food consumption is also considered to be relevant in this study.
In order to answer RQ3 (Are there differences in opinions about food experiences before and after COVID-19 re-strictions were eased and allowed them again?), a comparison of the sentiments expressed in texts in these two periods is carried out. Findings suggest that, even during the pandemic, more people gave positive than negative ratings to food destinations. Although the pandemic had a significant impact on consumers’ food behaviour (Foun-tain, 2022), we still see that the positive comments outnumber the negative ones. In addition, if we compare the dimensions included in comments before and after the pandemic, we can also see some differences. For example, there is an increase of the proportion of words linked to the service dimension. Due to the fact that service strategies have been implemented in order to mitigate the effects of COVID-19 on food experiences (Tuomi et al. 2021), it is important to notice that tourists also report more about services since the pandemic emerged. Conversely, there is a decrease of mentions linked to the place. This may be due to the fact that, due to closures and restrictions, the place where they eat the food is not as important. For example, for some time after the declaration of the pandemic, food consumption needed to be outdoors and social distancing should be maintained, so takeaway options and street food consumption was replaced in market and restaurant food consumption.
IMPLICATIONS
For theoretical contributions, the major findings of this study provide significant contributions to the current litera-ture in several aspects. First, the research advances the literature on the tourist behaviour of sharing experiences on social media. The study is not only positive experiences but also negative perceptions of dining experiences by clas-sifying sentiment categories. Also, the underlying dimensions of what people share about their experiences with others on social platforms were examined.
Second, the empirical evidence of differences between online reviews about food experiences before and during the COVID-19 crisis is exposed in this study. The modern technologies of sentiment analysis in the study can be a po-tential tool to accelerate the timely development of understanding tourist opinions and emotions towards destina-tions in the event of an epidemic.
Third, we also highlight the conceptional attributes of food experiences that people share on Google Local Guide review to the literature of tourism, while many scholars have commonly investigated reviews from online platforms like Twitter, Facebook, and TripAdvisor. Sentiment analysis in hospitality and tourism, Big data from the Google Local Guides platform, has presently not received much attention (Mehraliyev et al., 2022). We, therefore, encour-age future research to concern this platform as a powerful resource for data analysis.
Fourth, the article develops novel Natural Language Processing (NLP) techniques by using sentiment analysis, en-hanced with n-gram analysis, and word clouds to diagnose the sentiments of travellers’ perceptions posting in online reviews, whereas the existing literature refers to sharing food experiences by contention analysis and thematic anal-ysis approach (Wang et al., 2017). This is due to these technologies can facilitate the prompt understanding of cus-tomers’ sentiments and perceptions.
Moreover, this finding demonstrated how useful NLP techniques could be used not only to observe single words, but also to reveal how words are related to each other. With the aid of N-grams feature, we can determine which words frequently follow one another in a sentence or find co-occurrences and correlations between words that frequently follow one another. Evidently, using bigrams can explore a deeper understanding of the context of the sentiment direction. It can be novel knowledge for tourism scholars and practitioners to apply in future research because the analysis was done using Google’s Colab, which is a freely accessible cloud service. NLP capabilities can be applied to other tourism research topics to observe tourists’ behaviour and their attitude toward the brand image.
The research provides some important practical implications for tourism marketing. As part of the national strategy promoting gastronomic tourism, the insights provided can help the Tourism Authority of Thailand (TAT) and local tourism organisations to assess travellers’ sentiments and to understand the critical elements to increase tourists’ satisfaction in Ayutthaya. Given the tourism potential of local food, the TAT has published a campaign to highlight and promote gastronomic tourism (Sritama, 2018). The TAT (2019) plans to use food as a tourism marketing strat-egy to promote local destinations. To develop gastronomic tourism in Thailand under the TAT policy, it is necessary to create a positive tourism experience for tourists and to understand which elements contribute to increasing tourist satisfaction with their food experiences. Therefore, a comprehensive and detailed grasp of food experiences in Ayutthaya is required.
In addition, it can also contribute to business strategies for improving products and services by guiding potential brand image. Thus, the technique of opinion mining applied in this study helps all businesses, not only the tourism industry, to predict customer needs.
CONCLUSION
Findings of this study confirm that food is a key component in tourism destinations and it contributes to visitor experiences. They specifically reveal that: (1) users tend to share their positive food experiences rather than nega-tive ones; (2) Results summarise six critical attributes of sharing food experiences in reviews, consisting of food and product, atmosphere, service,
place, price, and activity; and (3) by comparing before and after Covid-19 periods, some differences are identified.
In spite of the existing literature on food experiences and eWOM, this article is innovative in different ways. First, it goes further in the understanding of food experiences as it is not limited to restaurant establishments, but it also includes street food consumption in night markets, street food stalls, etc.
Second, due to the recency of the COVID-19 pandemic, most of the previous studies have not considered its effects on UGC or have only examined the behaviour after the pandemic, without comparing it with what was commented before. Prior research to analyse online reviews has not produced evidence to examine the impact of the COVID-19 pandemic on food travel experiences. Greater understanding and insights into consumer sentiment evolution regard-ing COVID-19 pandemic are still lacking (Sangkaew & Zhu, 2022).
Third, this study uses sentiment analyses and NLP to explore people’s views toward food experiences in a tourist destination. These methods have been recently used in other tourism fields, such as hotels, but it is quite new to evaluate food experiences. Fourth, Google Local Guide is used as the source of data and its potential is acknowl-edged not only in terms of the number of reviews found on the platform but also the data elements that it includes (e.g., coordinates, number of likes, photos linked, etc.).
The study’s limitation is that reviews during the COVID-19 pandemic were unbalanced in the review dataset due to the lockdown. The dataset during the pandemic was collected in just a short period. The impact of the lockdown in Thailand affected the small number of reviews as food restaurants and food businesses were temporarily closed. Furthermore, one of the apparent limitations of using the bigram approach is the existence of the same set of terms but different positions (for example, ‘good food’ and ‘food good’). This can happen as bigram considers contiguous sequence words that often appear together (Liu, 2012). This research collected data from a single platform (Google Local Guide). In further work, the different data sources of food experience reviews, such as TripAdvisor and Twit-ter, might be compared to investigate the main attributes of food experience reviews.